filmov
tv
PromCon 2016: The Prometheus Time Series Database - Björn Rabenstein
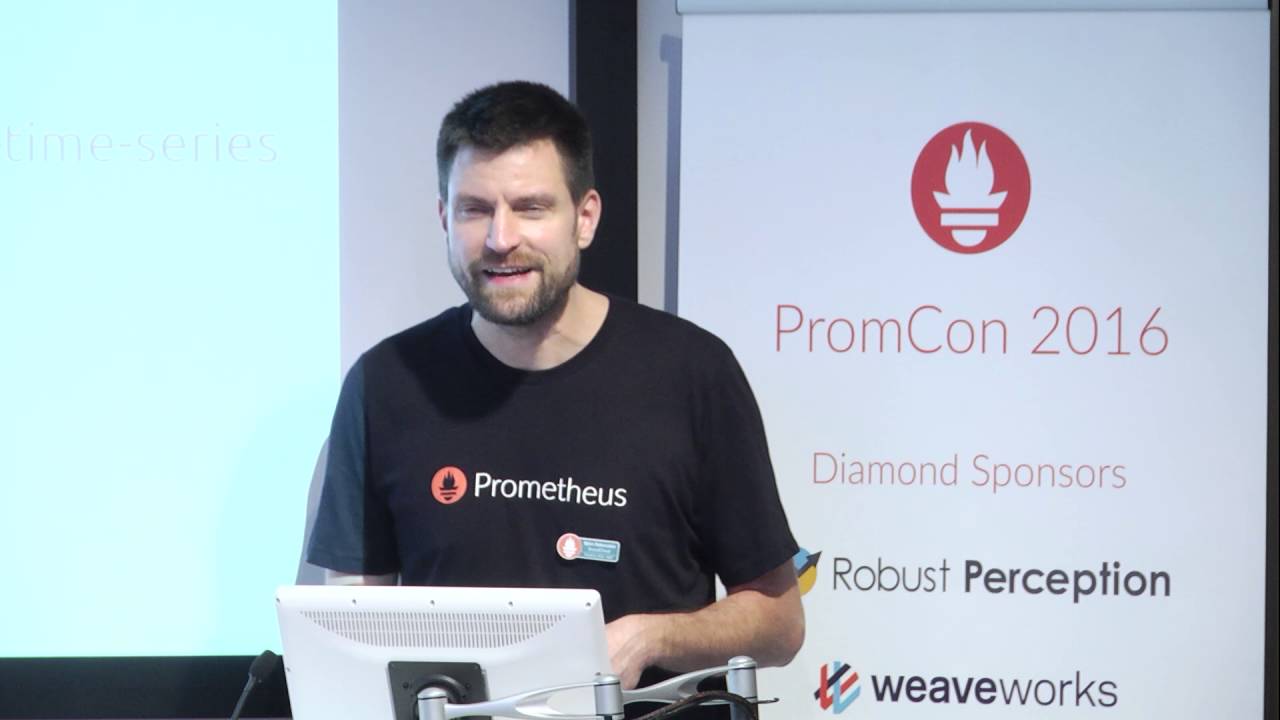
Показать описание
* Abstract:
Various time series databases (TSDBs) have been implemented on top of key-value stores with BigTable semantics. The TSDB that sits at the core of the Prometheus monitoring system started with a similar approach and was built on top of LevelDB. The Prometheus server as we know it today, however, uses a highly optimized custom storage layer for bulk sample data, enabling a single server to sustain an ingestion rate of 500,000 samples per second belonging to millions of time series. Very recent improvements of the in-memory representation of sample data resulted in an outstanding compression level of 1.3 bytes per sample in a typical production setup. A journey from fundamental challenges of TSDB design to details of the Prometheus storage layer.
* Speaker biography:
Björn is a production engineer at SoundCloud and one of the main Prometheus developers. Previously, he was a Site Reliability Engineer at Google and a number cruncher for science.
* Slides:
* PromCon website:
Various time series databases (TSDBs) have been implemented on top of key-value stores with BigTable semantics. The TSDB that sits at the core of the Prometheus monitoring system started with a similar approach and was built on top of LevelDB. The Prometheus server as we know it today, however, uses a highly optimized custom storage layer for bulk sample data, enabling a single server to sustain an ingestion rate of 500,000 samples per second belonging to millions of time series. Very recent improvements of the in-memory representation of sample data resulted in an outstanding compression level of 1.3 bytes per sample in a typical production setup. A journey from fundamental challenges of TSDB design to details of the Prometheus storage layer.
* Speaker biography:
Björn is a production engineer at SoundCloud and one of the main Prometheus developers. Previously, he was a Site Reliability Engineer at Google and a number cruncher for science.
* Slides:
* PromCon website:
PromCon 2016: The Prometheus Time Series Database - Björn Rabenstein
PromCon 2016: The History of Prometheus at SoundCloud - Tobias Schmidt
PromCon 2016: Dynamic Monitoring with Prometheus and Rancher - Chris Urwin, Edward Marshall
PromCon 2016: Scaling to a Million Machines with Prometheus - Matthew Campbell
PromCon 2016: Multitenant, Scale-Out Prometheus - Tom Wilkie
PromCon 2016: So You Want to Write an Exporter - Brian Brazil
PromCon 2016: Deploying Prometheus at DigitalOcean - Carlos Amedee
PromCon 2016: Prometheus Is Good for Your Small Startup - Ignacio P. Carretero
PromCon 2016: Prometheus Design and Philosophy - Why It Is the Way It Is - Julius Volz
PromCon 2016: Alerting in the Prometheus Universe - Fabian Reinartz
PromCon 2016: Lightning Talks - Deploying Full Prometheus Stacks via Juju Charms - JuanJo Ciarlante
PromCon 2017: Cortex: Prometheus as a Service, One Year On - Tom Wilkie
PromCon 2016: Conference Recap
PromCon 2016: Welcome and Introduction - Julius Volz
PromCon 2016: Lightning Talks - Why We Love Prometheus (and You Should Too) - Gil Fliker
PromCon 2016: Graphing MySQL Performance with Prometheus and Grafana - Roman Vynar
PromCon 2016: Lightning Talks - Prometheus on the Small Scale - Jonas Große Sundrup
PromCon 2016: Life of a Label - Brian Brazil
PromCon 2016: Prometheus as a Customer-Facing Monitoring Tool for SpatialOS - Dmytro Kislov
PromCon 2016: Lightning Talks - PromQL You Probably (Maybe) Don't Use - Brian Brazil
PromCon 2016: Lightning Talks - vulcan: An API-compatible Alternative to Prometheus - Ian Hansen
PromCon 2016: Lightning Talks - An Exploration of the Formal Properties of PromQL - Brian Brazil
PromCon EU 2019: OpenMetrics: What Does It Mean for You
PromCon 2017: Lightning Talk - Prometheus@Home - Richard Hartmann
Комментарии