filmov
tv
GROKKED LLM beats RAG Reasoning (Part 3)
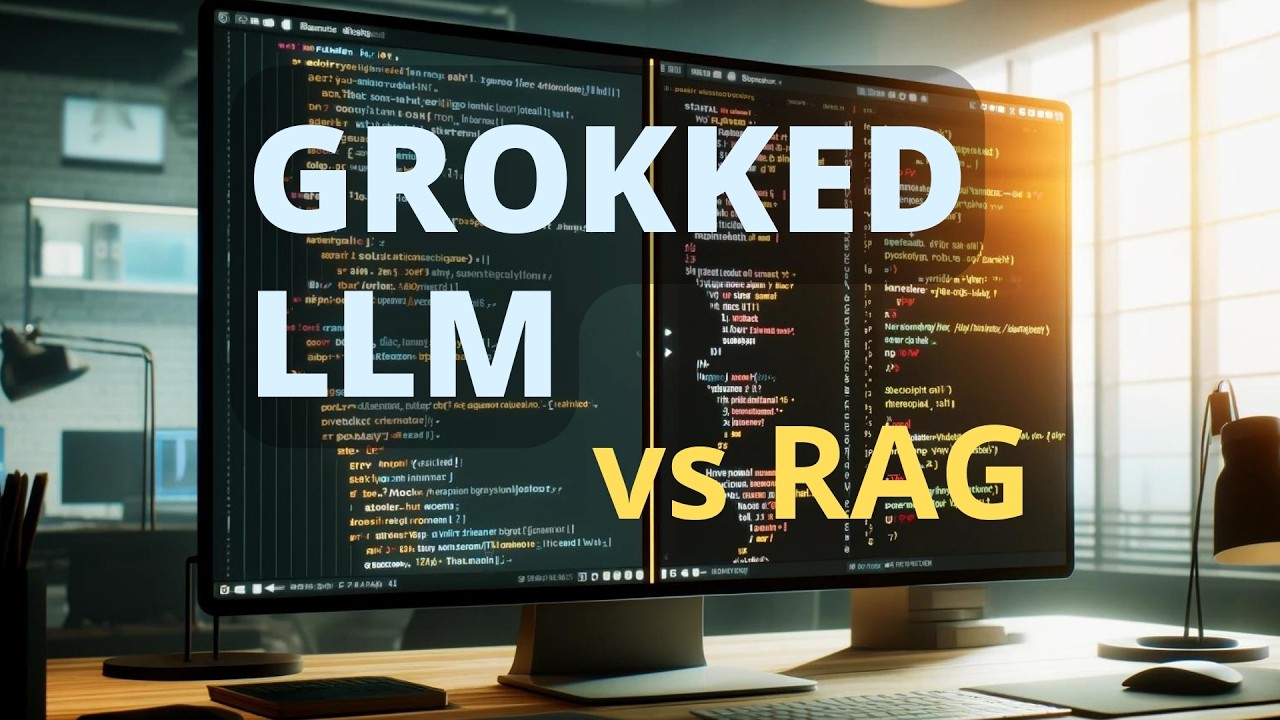
Показать описание
We open the black box of GROKKED LLMs and analyze each layer of the transformer architecture for its performance in causal reasoning, after the grokking phase transition of our LLM.
Current research in AI clearly indicates that established LLMs, like Gemini Pro 1.5 or GPT-4 Turbo fail in deep reasoning, even when integrated in complex RAG systems.
A Grokking phase transition is essential for LLM to active their performance phase, reaching close to 99% accuracy for "un-seen" tasks in the development and test datasets.
#airesearch
#ainews
#insights
Current research in AI clearly indicates that established LLMs, like Gemini Pro 1.5 or GPT-4 Turbo fail in deep reasoning, even when integrated in complex RAG systems.
A Grokking phase transition is essential for LLM to active their performance phase, reaching close to 99% accuracy for "un-seen" tasks in the development and test datasets.
#airesearch
#ainews
#insights
Комментарии