filmov
tv
Lesson 10 (2019) - Looking inside the model
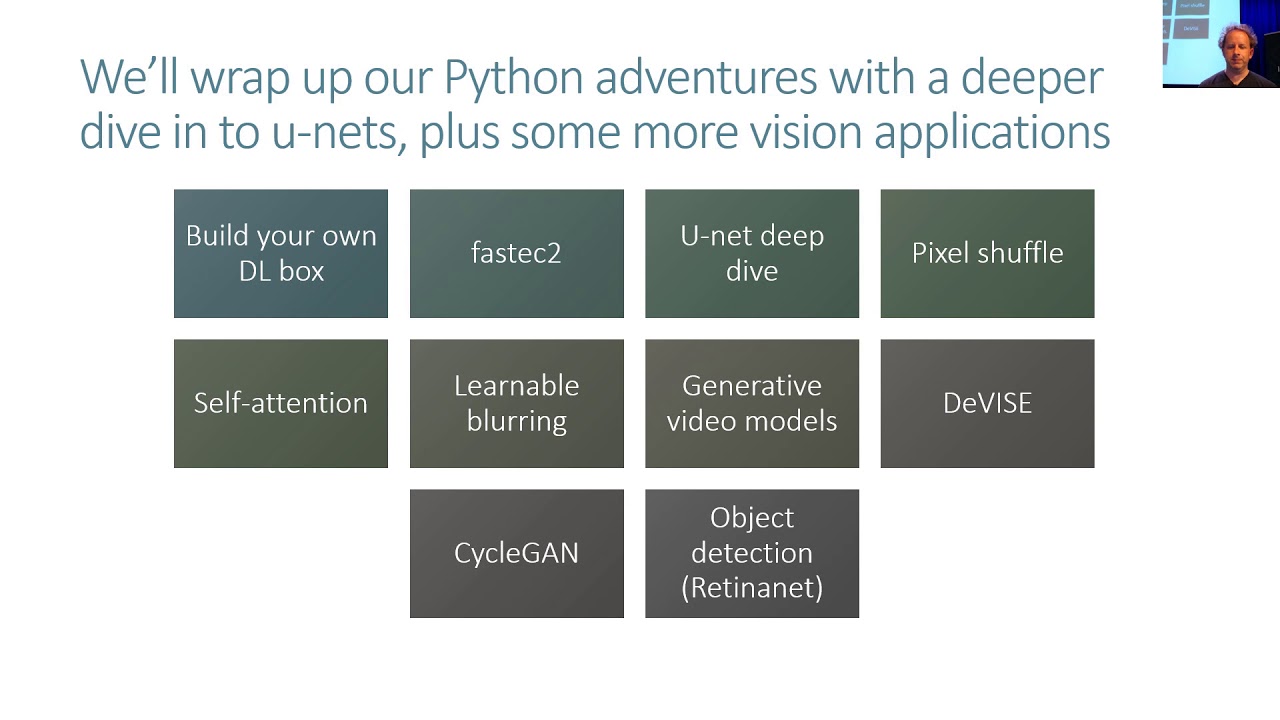
Показать описание
In lesson 10 we start with a deeper dive into the underlying idea of callbacks and event handlers. We look at many different ways to implement callbacks in Python, and discuss their pros and cons. Then we do a quick review of some other important foundations:
- `__dunder__` special symbols in Python
- How to navigate source code using your editor
- Variance, standard deviation, covariance, and correlation
- Softmax
- Exceptions as control flow
Next up, we use the callback system we've created to set up CNN training on the GPU.
Then we move on to the main topic of this lesson: looking inside the model to see how it behaves during training. To do so, we first need to learn about *hooks* in PyTorch, which allow us to add callbacks to the forward and backward passes. We will use hooks to track the changing distribution of our activations in each layer during training. By plotting this distributions, we can try to identify problems with our training.
In order to fix the problems we see, we try changing our activation function, and introducing batchnorm. We study the pros and cons of batchnorm, and note some areas where it performs poorly. Finally, we develop a new kind of normalization layer to overcome these problems, and compare it to previously published approaches, and see some very encouraging results.
Комментарии