filmov
tv
Animesh Garg - Building blocks of Generalizable Autonomy in Robotics
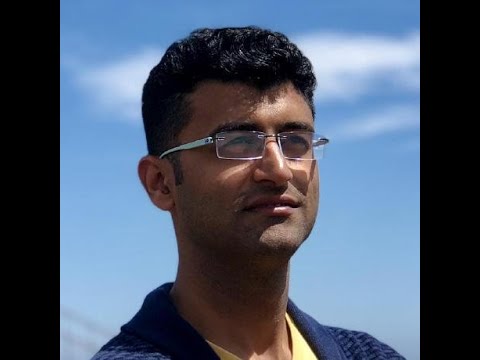
Показать описание
Talk abstract: My approach to Generalizable Autonomy posits that interactive learning across families of tasks is essential for discovering efficient representation and inference mechanisms. Arguably, a cognitive concept or a dexterous skill should be reusable across task instances to avoid constant relearning. It is insufficient to learn to “open a door”, and then have to re-learn it for a new door, or even windows & cupboards. Thus, I focus on three key questions: (1) Representational biases for embodied reasoning, (2) Causal Inference in abstract sequential domains, and (3) Interactive Policy Learning under uncertainty.
In this talk I will first through example lay bare the need for structured biases in modern RL algorithms in the context of robotics. This will span state, actions, learning mechanisms and network architectures. Secondly, we will talk about discovery of latent causal structure in dynamics for planning. Finally I will demonstrate how large scale data generation combined with insights from structure learning can enable sample efficient algorithms for practical systems. In this talk I will focus mainly on manipulation, but my work has been applied to surgical robotics and legged locomotion as well.
Speaker bio: Animesh is an Assistant Professor of Computer Science at the University of Toronto and a Faculty Member at the Vector Institute where he leads the Toronto People, AI, and Robotics (PAIR) research group. Animesh is affiliated with Mechanical and Industrial Engineering (courtesy) and UofT Robotics Institute. Animesh also spends time as a research scientist at Nvidia Research in ML for Robotics. Prior to this, Animesh was a postdoc at Stanford AI Lab. Animesh earned a Ph.D. from UC Berkeley, an MS from Georgia Institute of Technology and a BE from the University of Delhi. Animesh’s research focuses on machine learning algorithms for perception and control in robotics. Animesh aim’s to enable Generalizable Autonomy through efficient robot learning for long-term sequential decision making. The principal technical focus lies in understanding representations and algorithms to enable simplicity and generality of learning for interaction in autonomous agents. Animesh actively works on applications of robot manipulation in industrial and healthcare robotics.
===
In this talk I will first through example lay bare the need for structured biases in modern RL algorithms in the context of robotics. This will span state, actions, learning mechanisms and network architectures. Secondly, we will talk about discovery of latent causal structure in dynamics for planning. Finally I will demonstrate how large scale data generation combined with insights from structure learning can enable sample efficient algorithms for practical systems. In this talk I will focus mainly on manipulation, but my work has been applied to surgical robotics and legged locomotion as well.
Speaker bio: Animesh is an Assistant Professor of Computer Science at the University of Toronto and a Faculty Member at the Vector Institute where he leads the Toronto People, AI, and Robotics (PAIR) research group. Animesh is affiliated with Mechanical and Industrial Engineering (courtesy) and UofT Robotics Institute. Animesh also spends time as a research scientist at Nvidia Research in ML for Robotics. Prior to this, Animesh was a postdoc at Stanford AI Lab. Animesh earned a Ph.D. from UC Berkeley, an MS from Georgia Institute of Technology and a BE from the University of Delhi. Animesh’s research focuses on machine learning algorithms for perception and control in robotics. Animesh aim’s to enable Generalizable Autonomy through efficient robot learning for long-term sequential decision making. The principal technical focus lies in understanding representations and algorithms to enable simplicity and generality of learning for interaction in autonomous agents. Animesh actively works on applications of robot manipulation in industrial and healthcare robotics.
===