filmov
tv
Lagrangian Neural Network (LNN) [Physics Informed Machine Learning]
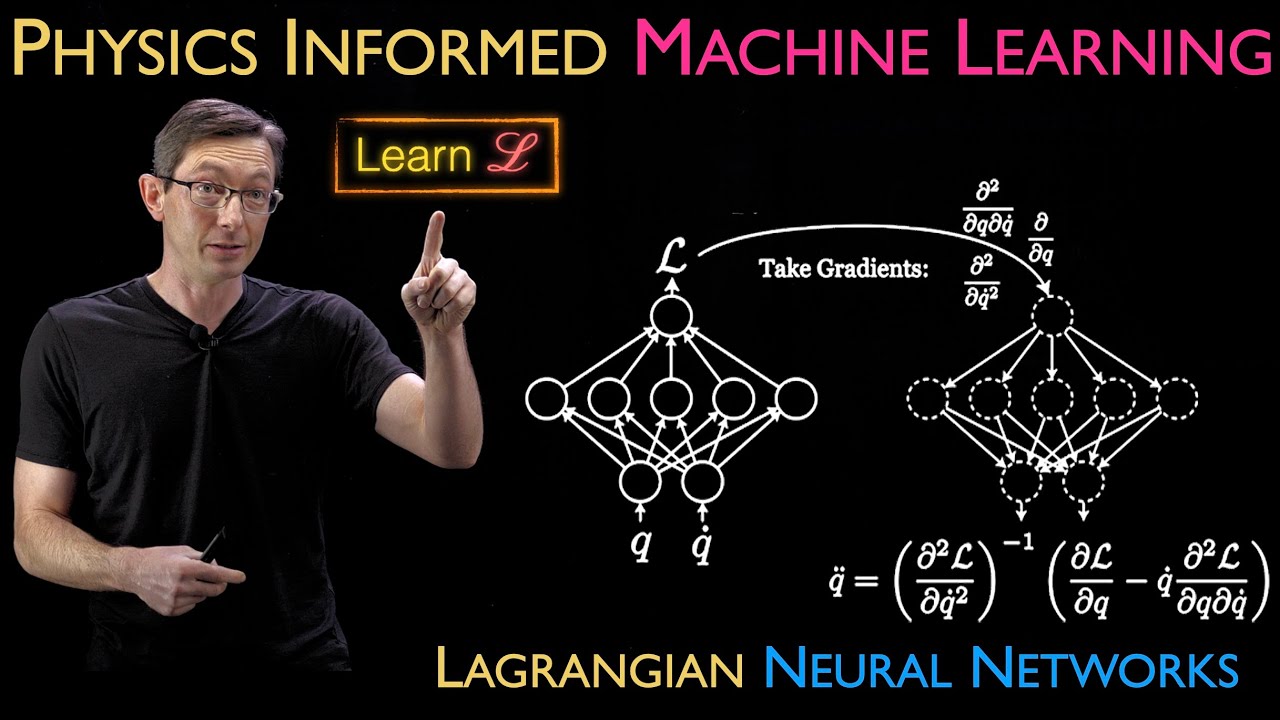
Показать описание
This video was produced at the University of Washington, and we acknowledge funding support from the Boeing Company
%%% CHAPTERS %%%
00:00 Intro
02:14 Background: The Lagrangian Perspective
05:14 Background: Lagrangian Dynamics
06:46 Variational Integrators
10:40 The Parallel to Machine Learning/ Why LNNs
13:22 LNNs: Underlying Concept
16:02 LNNs are ODEs/ LNNs: Implementation
18:21 Outro
%%% CHAPTERS %%%
00:00 Intro
02:14 Background: The Lagrangian Perspective
05:14 Background: Lagrangian Dynamics
06:46 Variational Integrators
10:40 The Parallel to Machine Learning/ Why LNNs
13:22 LNNs: Underlying Concept
16:02 LNNs are ODEs/ LNNs: Implementation
18:21 Outro
Lagrangian Neural Network (LNN) [Physics Informed Machine Learning]
Lagrangian Neural Networks | AISC
Lagrangian Mechanics - A beautiful way to look at the world
Enhancing the Inductive Biases of Graph Neural ODE for Modeling Dynamical Systems
Benchmarking Energy-Conserving Neural Networks for Learning Dynamics from Data | L4DC 2021
Machine Learning for Computational Fluid Dynamics
Logical Neural Networks Pt 1/4: Central Ideas
Доклады ИАД (Hamiltonian & Lagrangian NN, Dataset dynamics, Geometric manifolds)
Logical Neural Networks: Towards Unifying Statistical and Symbolic AI
Fabian Faulstich - pure state v-representability of density matrix embedding - augmented lagrangian
Advanced Machine Learning with Neural Networks 2021 - Class 6 - Machine learning for fluid dynamics
Normalizing Flows for scientific applications
A theory of deep learning: explaining the approximation, optimization and generalization puzzles Pt2
KDD 2020: Lecture Style Tutorials: Physics Inspired Models in Artificial Intelligence-Part 1
The Physics of Self-Replication and Nanotechnology
Shih Chieh Hsu, “GPU or FPGA-accelerated AI Inference as a service for LHC physics”
InvertibleNetworks.jl - Memory efficient deep learning ... | Philip A. Witte et al | JuliaCon2021
Mathematical aspects of neural network approximation and learning by Joan Bruna
Jérôme Darbon: 'Overcoming the curse of dimensionality for some Hamilton-Jacobi partial differe...
This is what Feynman's PhD thesis looks like 👀
Nathaniel Trask:Structure preserving architectures for SciML
Moravec's Paradox - Why are machines so smart, yet so dumb?
Nat Trask: Structure preserving deep learning architectures
Friston's 'Free Energy Principle' | The Most INTENSE Theory of Reality
Комментарии