filmov
tv
Interpretable Latent Variable Models Demonstrate Flexible Neural Control of Spinal Motor Units
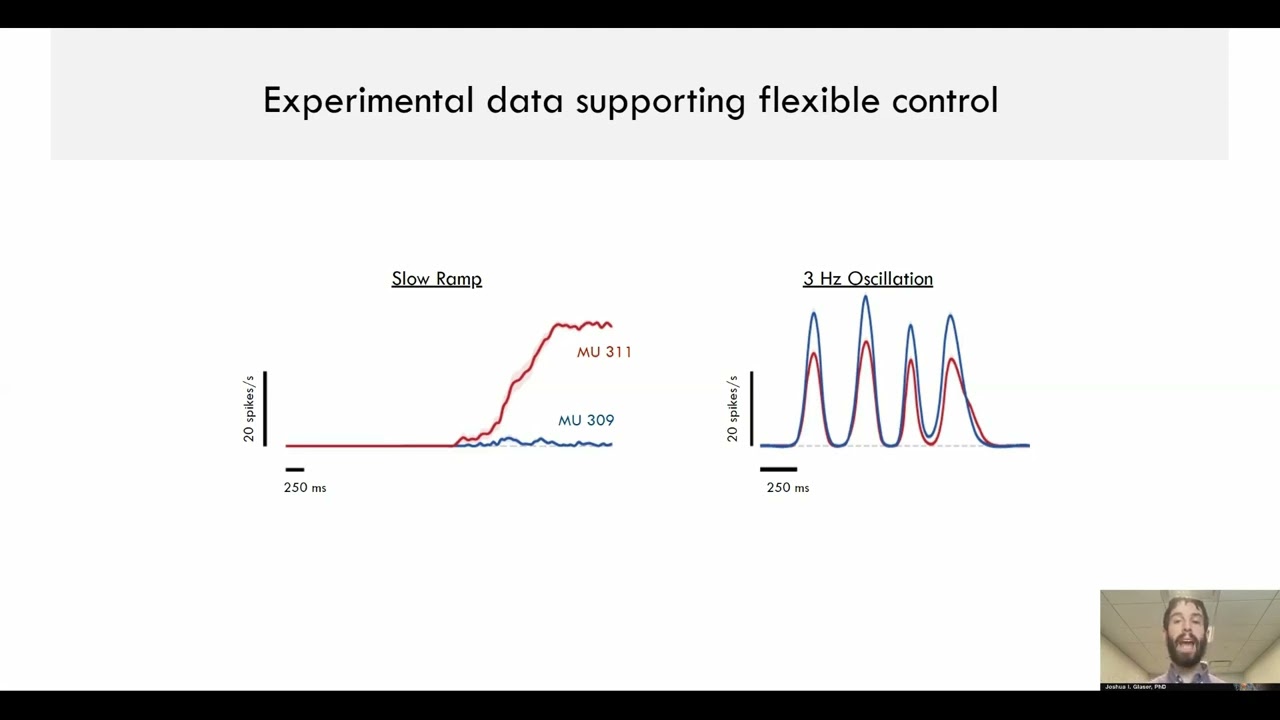
Показать описание
Presented By: Joshua I. Glaser, PhD
Speaker Biography: Dr. Joshua Glaser is an Assistant Professor at Northwestern University in the Departments of Neurology and Computer Science. He previously received his BS in Mathematics and Physics from the University of Illinois Urbana-Champaign, completed his PhD in Neuroscience at Northwestern University, and did postdoctoral research at Columbia University's Center for Theoretical Neuroscience. His lab's research focuses on developing more interpretable machine learning tools to analyze neural activity data.
Webinar: Interpretable Latent Variable Models Demonstrate Flexible Neural Control of Spinal Motor Units
Webinar Abstract: In this talk, I will discuss the development of interpretable machine learning models to test scientific hypotheses, with a specific focus on spinal motor control. Voluntary movement requires communication from cortex to the spinal cord, where a dedicated pool of motor units (MUs) activates each muscle. The canonical description of MU function states that cortex cannot flexibly control MUs independently, but rigidly supplies each pool with a single common drive. Here, we test this “rigid control” theory through both 1) novel experiments in which many MUs were stably recorded while macaques generated widely varying forces, and 2) the development of a latent variable model that represents the rigid control theory. By testing whether this latent variable model could accurately fit the recorded MU data, we were able to quantitatively test the rigid control theory. We found that a single latent variable is unable to describe MU activity across widely ranging behaviors, demonstrating that, contrary to canonical beliefs, MU activity is flexibly controlled to meet task demands.
Earn PACE Credits:
Labroots on Social:
SnapChat: labroots_inc
Speaker Biography: Dr. Joshua Glaser is an Assistant Professor at Northwestern University in the Departments of Neurology and Computer Science. He previously received his BS in Mathematics and Physics from the University of Illinois Urbana-Champaign, completed his PhD in Neuroscience at Northwestern University, and did postdoctoral research at Columbia University's Center for Theoretical Neuroscience. His lab's research focuses on developing more interpretable machine learning tools to analyze neural activity data.
Webinar: Interpretable Latent Variable Models Demonstrate Flexible Neural Control of Spinal Motor Units
Webinar Abstract: In this talk, I will discuss the development of interpretable machine learning models to test scientific hypotheses, with a specific focus on spinal motor control. Voluntary movement requires communication from cortex to the spinal cord, where a dedicated pool of motor units (MUs) activates each muscle. The canonical description of MU function states that cortex cannot flexibly control MUs independently, but rigidly supplies each pool with a single common drive. Here, we test this “rigid control” theory through both 1) novel experiments in which many MUs were stably recorded while macaques generated widely varying forces, and 2) the development of a latent variable model that represents the rigid control theory. By testing whether this latent variable model could accurately fit the recorded MU data, we were able to quantitatively test the rigid control theory. We found that a single latent variable is unable to describe MU activity across widely ranging behaviors, demonstrating that, contrary to canonical beliefs, MU activity is flexibly controlled to meet task demands.
Earn PACE Credits:
Labroots on Social:
SnapChat: labroots_inc