filmov
tv
Michael Stonebraker, TAMR | MIT CDOIQ 2019
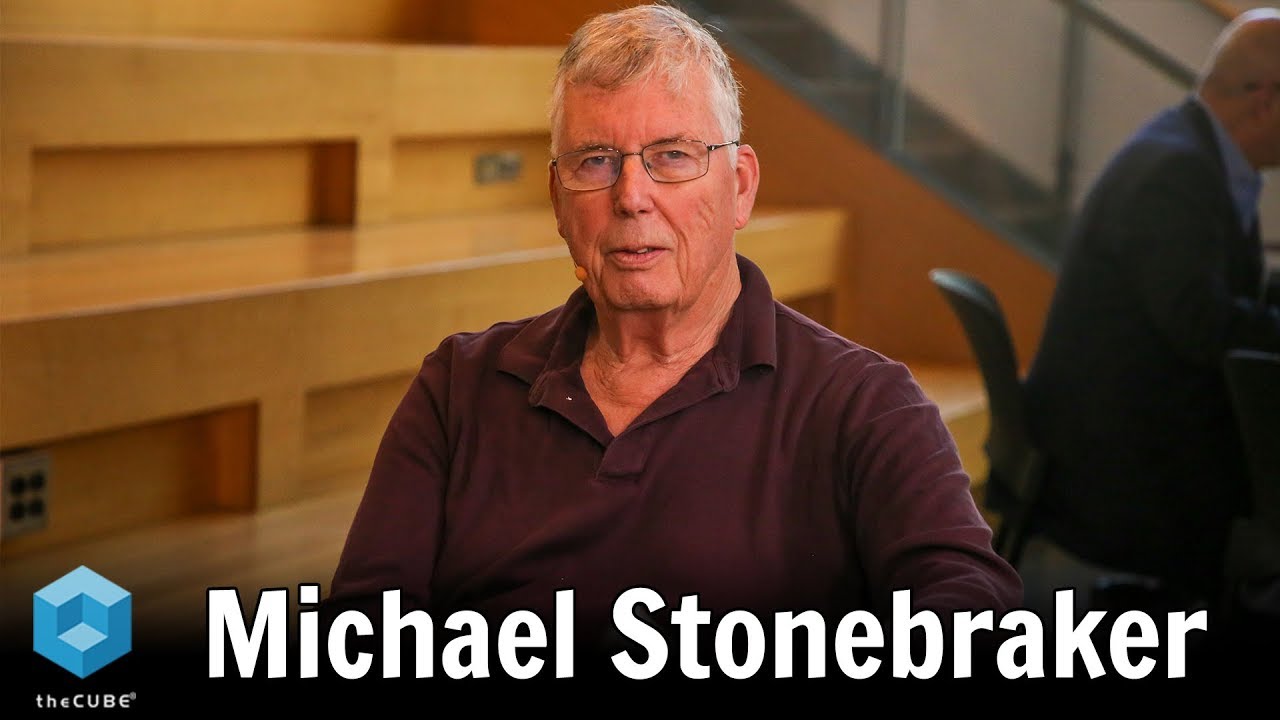
Показать описание
#theCUBE #MITCDOIQ
Machine learning tips the scale
The market is not exactly lacking proposed solutions to the data-swamp problem. Plenty of tech companies are bringing them out or updating their original offerings. The main technologies typically used in these systems, however, have a key deficiency, Stonebraker pointed out. These traditional technologies include extract, transform, load systems and master data management systems.
“A dirty, little secret is that technology does not scale,” Stonebraker said.
ETL is based on the premise that someone really bright will come up with a global data model for all data sources a user wants. Then a human interviews each business unit to see what data they’ve got, how to get it in the global data model, load it into the data warehouse and so on. Processes that are that human intensive tend to not scale, according to Stonebraker. They typically wind up with 10 or 20 sources integrated in the data warehouse, he added.
Is that a sufficient number? Let’s look at a real-world company. Tamr customer Toyota Motor Europe has distributors in different countries (sometimes cantons). If someone buys a Toyota in Spain and then moves to France, the French company knows nothing about the car owner.
In total, TME has 250 separate customer databases with 40 million total records in 50 languages. The company is in the process of integrating them into a single customer database to solve this customer-servicing issue. Machine learning provides a plausible means to do this. “I’ve never seen an ETL system capable of dealing with that kind of scale,” Stonebraker said.
The reason MDM doesn’t scale is basically because it’s rules-based, Stonebraker explained. Another Tamr customer, General Electric Co., wants to do spend analytics. It had 20 million spend transactions from the year before last. It tried to classify all of those into a rules-based hierarchy.
“So GE wrote 500 rules, which is about the most any single human can get their arms around,” he said. “That classified 2 million of the 20 million transactions. You’ve now got 18 to go. And another 500 rules is not going to give you 2 million more.
That, he noted, is the law of diminishing returns. “You’re going to have to write a huge number of rules that no one can possibly understand,” Stonebraker said. “If you don’t use machine learning, you’re absolutely toast.”
Комментарии