filmov
tv
Wavelets-based Feature Extraction - Part2: Wavelet Scattering Transform
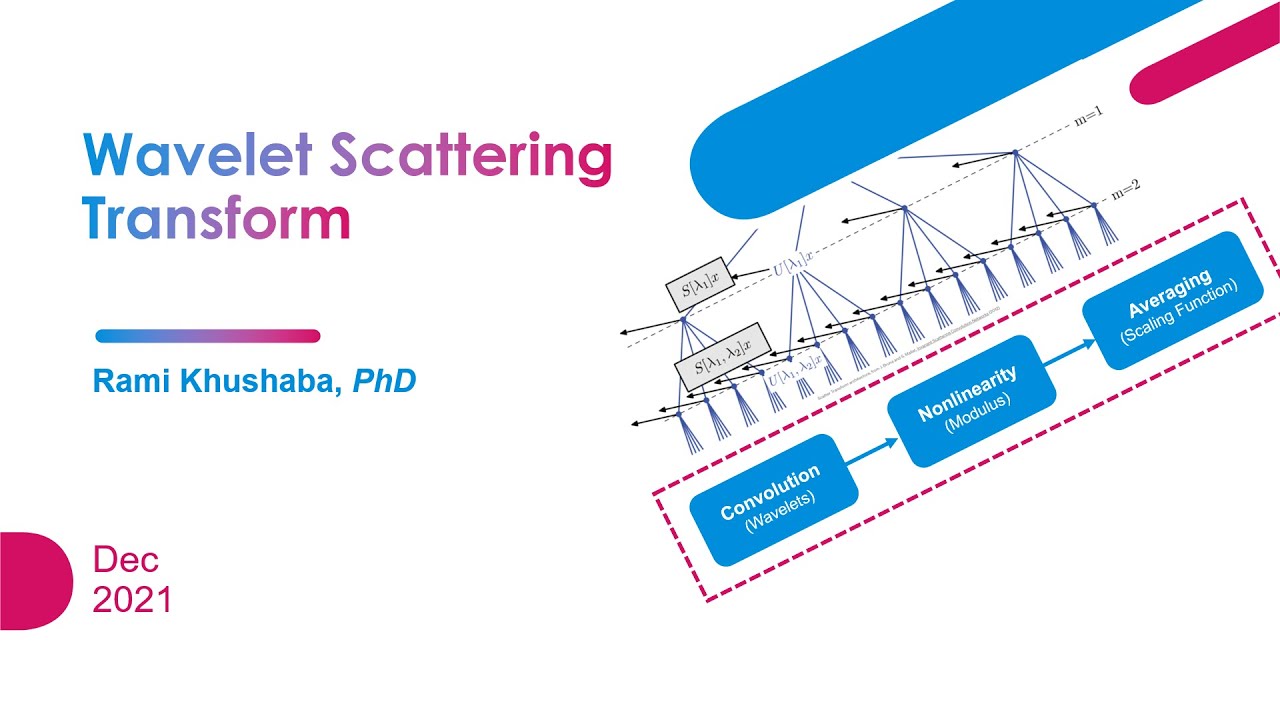
Показать описание
This is the second part of the video that discussed the use of wavelet for feature extraction from signals and images. The focus here is on Wavelet Scattering Transform, this is a deep convolutional network that does not need to iterate to learn, but provides very informative features.
#WaveletScatter #Wavelets #Scattering #ScatteringTransform
#WaveletScatter #Wavelets #Scattering #ScatteringTransform
Wavelets-based Feature Extraction - Part2: Wavelet Scattering Transform
Wavelets-based Feature Extraction
Wavelets: a mathematical microscope
What Are Wavelets | Understanding Wavelets, Part 1
The Wavelet Transform for Beginners
Model Based Design for Predictive Maintenance, Part 2 Feature Extraction
Wavelet Transform based Preprocessing and Features Extraction with MATLAB
Wavelet transform for Images
Haar Features for Face Detection | Face Detection
Introduction to Wavelet Transform - version 2
An introduction to the wavelet transform (and how to draw with them!)
The Wavelet Transform | Introduction & Example Code
Wavelet filtering of undersampled MRI using trainable wavelets and CNN
55 - Feature Extraction Introduction
Rambling about the Wavelet Transform and Fourier Transform
Dorsal hand vein pattern feature extraction with wavelet transforms
Wavelet Time-Frequency Analysis
Image classification using Wavelet transform, PCA and Perceptron model
How to run Object Based Classification with eCognition: - Water features extraction: Part II
2-Minute Tutorial: Exploring Wavelet Layers with the ExtractWaveletLayers Script!
Robotics 3 - Chapter 5 - Feature Extraction (Part 2)
How to visualize filter bank of a wavelet with Python
Tutorial 74 - What are Gabor filters and how to use them to generate features for machine learning?
Spoken Digit Recognition with Wavelet Scattering Transform and Deep Learning Using MATLAB
Комментарии