filmov
tv
Complete Machine Learning In 6 Hours| Krish Naik
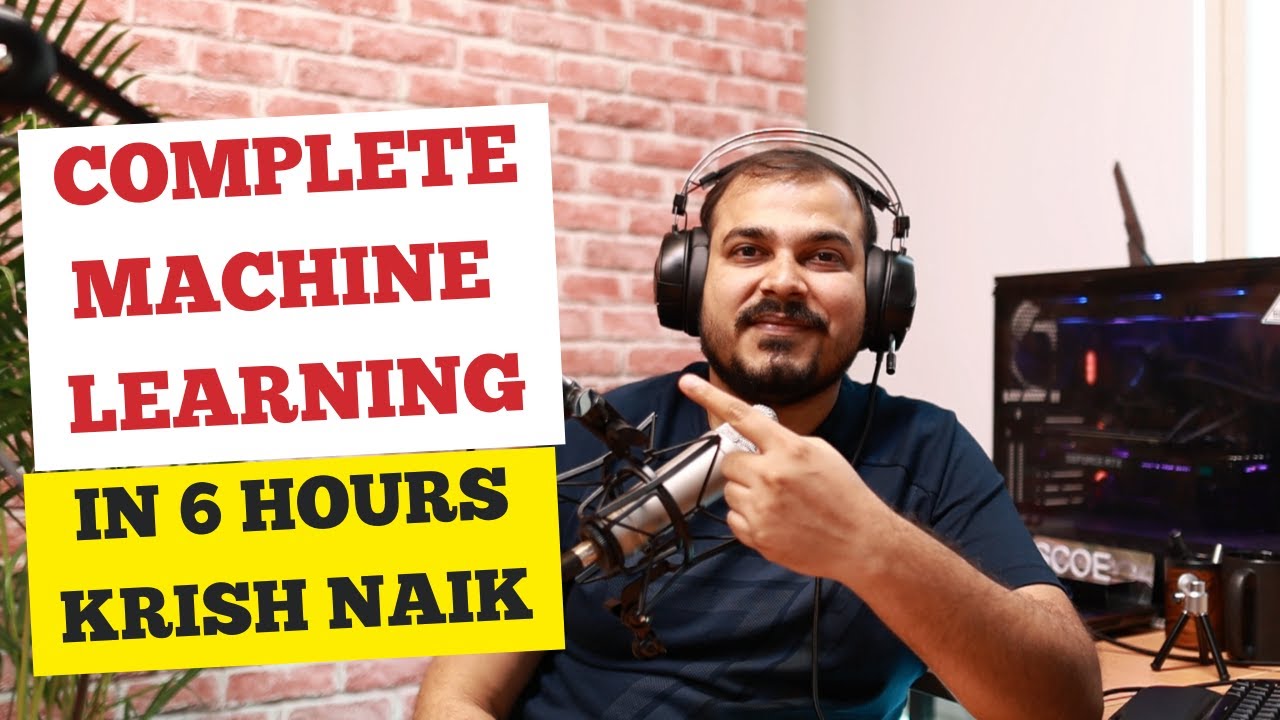
Показать описание
All the materials are available in the below link
Time Stamp:
00:00:00 Introduction
00:01:25 AI Vs ML vs DL vs Data Science
00:07:56 Machine LEarning and Deep Learning
00:09:05 Regression And Classification
00:18:14 Linear Regression Algorithm
01:07:14 Ridge And Lasso Regression Algorithms
01:33:08 Logistic Regression Algorithm
02:13:52 Linear Regression Practical Implementation
02:28:30 Ridge And Lasso Regression Practical Implementation
02:54:21 Naive Baye's Algorithms
03:16:02 KNN Algorithm Intuition
03:23:47 Decision Tree Classification Algorithms
03:57:05 Decision Tree Regression Algorithms
04:02:57 Practical Implementation Of Deicsion Tree Classifier
04:09:14 Ensemble Bagging And Bossting Techniques
04:21:29 Random Forest Classifier And Regressor
04:29:58 Boosting, Adaboost Machine Learning Algorithms
04:47:30 K Means Clustering Algorithm
05:01:54 Hierarichal Clustering Algorithms
05:11:28 Silhoutte Clustering- Validating Clusters
05:17:46 Dbscan Clustering Algorithms
05:25:57 Clustering Practical Examples
05:35:51 Bias And Variance Algorithms
05:43:44 Xgboost Classifier Algorithms
06:00:00 Xgboost Regressor Algorithms
06:19:04 SVM Algorithm Machine LEarning Algorithm
---------------------------------------------------------------------------------------------------------------------
►Data Science Projects:
►Learn In One Tutorials
►Learn In a Week Playlist
►Detailed Playlist:
Time Stamp:
00:00:00 Introduction
00:01:25 AI Vs ML vs DL vs Data Science
00:07:56 Machine LEarning and Deep Learning
00:09:05 Regression And Classification
00:18:14 Linear Regression Algorithm
01:07:14 Ridge And Lasso Regression Algorithms
01:33:08 Logistic Regression Algorithm
02:13:52 Linear Regression Practical Implementation
02:28:30 Ridge And Lasso Regression Practical Implementation
02:54:21 Naive Baye's Algorithms
03:16:02 KNN Algorithm Intuition
03:23:47 Decision Tree Classification Algorithms
03:57:05 Decision Tree Regression Algorithms
04:02:57 Practical Implementation Of Deicsion Tree Classifier
04:09:14 Ensemble Bagging And Bossting Techniques
04:21:29 Random Forest Classifier And Regressor
04:29:58 Boosting, Adaboost Machine Learning Algorithms
04:47:30 K Means Clustering Algorithm
05:01:54 Hierarichal Clustering Algorithms
05:11:28 Silhoutte Clustering- Validating Clusters
05:17:46 Dbscan Clustering Algorithms
05:25:57 Clustering Practical Examples
05:35:51 Bias And Variance Algorithms
05:43:44 Xgboost Classifier Algorithms
06:00:00 Xgboost Regressor Algorithms
06:19:04 SVM Algorithm Machine LEarning Algorithm
---------------------------------------------------------------------------------------------------------------------
►Data Science Projects:
►Learn In One Tutorials
►Learn In a Week Playlist
►Detailed Playlist:
Комментарии