filmov
tv
Handling imbalanced dataset in machine learning | Deep Learning Tutorial 21 (Tensorflow2.0 & Python)
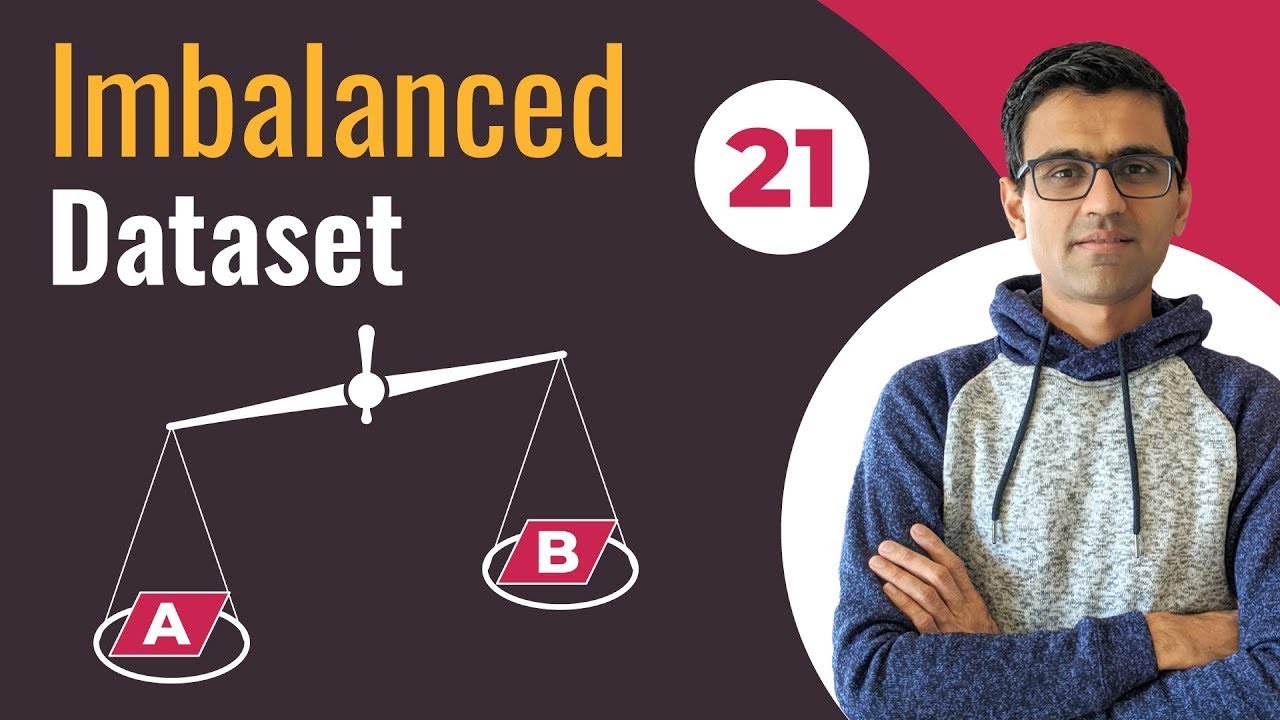
Показать описание
Credit card fraud detection, cancer prediction, customer churn prediction are some of the examples where you might get an imbalanced dataset. Training a model on imbalanced dataset requires making certain adjustments otherwise the model will not perform as per your expectations. In this video I am discussing various techniques to handle imbalanced dataset in machine learning. I also have a python code that demonstrates these different techniques. In the end there is an exercise for you to solve along with a solution link.
#imbalanceddataset #imbalanceddatasetinmachinelearning #smotetechnique #deeplearning #imbalanceddatamachinelearning
Topics
00:00 Overview
00:01 Handle imbalance using under sampling
02:05 Oversampling (blind copy)
02:35 Oversampling (SMOTE)
03:00 Ensemble
03:39 Focal loss
04:47 Python coding starts
07:56 Code - undersamping
14:31 Code - oversampling (blind copy)
19:47 Code - oversampling (SMOTE)
24:26 Code - Ensemble
35:48 Exercise
#️⃣ Social Media #️⃣
DISCLAIMER: All opinions expressed in this video are of my own and not that of my employers'.
#imbalanceddataset #imbalanceddatasetinmachinelearning #smotetechnique #deeplearning #imbalanceddatamachinelearning
Topics
00:00 Overview
00:01 Handle imbalance using under sampling
02:05 Oversampling (blind copy)
02:35 Oversampling (SMOTE)
03:00 Ensemble
03:39 Focal loss
04:47 Python coding starts
07:56 Code - undersamping
14:31 Code - oversampling (blind copy)
19:47 Code - oversampling (SMOTE)
24:26 Code - Ensemble
35:48 Exercise
#️⃣ Social Media #️⃣
DISCLAIMER: All opinions expressed in this video are of my own and not that of my employers'.
Комментарии