filmov
tv
Graph Language Models EXPLAINED in 5 Minutes! [Author explanation 🔴 at ACL 2024]
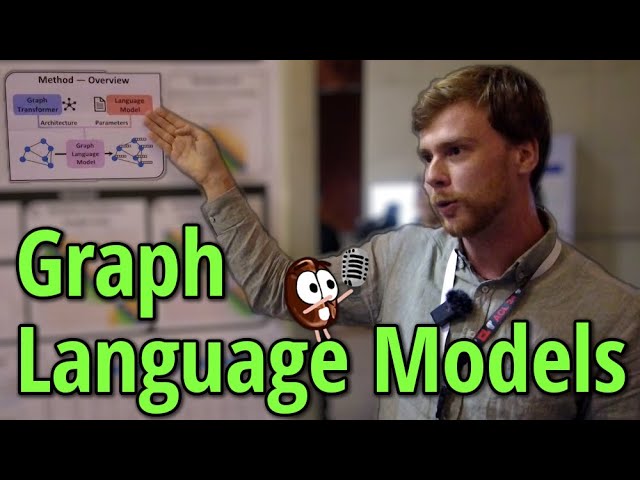
Показать описание
How to make powerful LLMs understand graphs and their structure? 🕸️ With Graph Language Models! They take a pre-trained language model and fit it with the ability to process graphs. Watch if you're curious about how this works (hint: choose the right positional embeddings)!
Follow Moritz Plenz (first author) on:
Thanks to our Patrons who support us in Tier 2, 3, 4: 🙏
Dres. Trost GbR, Siltax, Vignesh Valliappan, Michael, Sunny Dhiana, Andy Ma
Outline:
00:00 LLM for graphs
01:08 Motivation
02:02 Key idea of Graph LLMs
02:25 Relative Positional Encodings
03:00 Method (Graph LLMs)
04:04 Experiments and Evaluation
04:49 Results
06:07 Outro
▀▀▀▀▀▀▀▀▀▀▀▀▀▀▀▀▀▀▀▀▀▀▀▀▀▀
🔥 Optionally, pay us a coffee to help with our Coffee Bean production! ☕
Join this channel as a Bean Member to get access to perks:
▀▀▀▀▀▀▀▀▀▀▀▀▀▀▀▀▀▀▀▀▀▀▀▀▀▀
🔗 Links:
#AICoffeeBreak #MsCoffeeBean #MachineLearning #AI #research
Video editing: Nils Trost
Music 🎵 : Space Navigator – Sarah, the Illstrumentalist
Follow Moritz Plenz (first author) on:
Thanks to our Patrons who support us in Tier 2, 3, 4: 🙏
Dres. Trost GbR, Siltax, Vignesh Valliappan, Michael, Sunny Dhiana, Andy Ma
Outline:
00:00 LLM for graphs
01:08 Motivation
02:02 Key idea of Graph LLMs
02:25 Relative Positional Encodings
03:00 Method (Graph LLMs)
04:04 Experiments and Evaluation
04:49 Results
06:07 Outro
▀▀▀▀▀▀▀▀▀▀▀▀▀▀▀▀▀▀▀▀▀▀▀▀▀▀
🔥 Optionally, pay us a coffee to help with our Coffee Bean production! ☕
Join this channel as a Bean Member to get access to perks:
▀▀▀▀▀▀▀▀▀▀▀▀▀▀▀▀▀▀▀▀▀▀▀▀▀▀
🔗 Links:
#AICoffeeBreak #MsCoffeeBean #MachineLearning #AI #research
Video editing: Nils Trost
Music 🎵 : Space Navigator – Sarah, the Illstrumentalist
Graph Language Models EXPLAINED in 5 Minutes! [Author explanation 🔴 at ACL 2024]
Language Models are Open Knowledge Graphs (Paper Explained)
How Large Language Models Work
How large language models work, a visual intro to transformers | Chapter 5, Deep Learning
What is a Knowledge Graph?
GraphRAG: LLM-Derived Knowledge Graphs for RAG
Fusing Knowledge Graphs and Large Language Models
Large Language Models from scratch
🔵 Embed the latest from SAP HANA’s AI functions into your SAP BTP Application
What is Retrieval-Augmented Generation (RAG)?
Knowledge Graph Construction Demo from raw text using an LLM
Graph-of-Thoughts (GoT) for AI reasoning Agents
Attention in transformers, visually explained | Chapter 6, Deep Learning
The Future of Knowledge Graphs in a World of Large Language Models
Large Language Models and Knowledge Graphs: Merging Flexibility and Structure
Transformer Neural Networks, ChatGPT's foundation, Clearly Explained!!!
What are Large Language Models (LLMs)?
“What's wrong with LLMs and what we should be building instead” - Tom Dietterich - #VSCF2023...
Graphs, Vectors and Machine Learning - Computerphile
Build a Large Language Model AI Chatbot using Retrieval Augmented Generation
Large Language Models - Explained via Readable Video
Transformers with Knowledge Graph | K-BERT: Enabling Language Representation with Knowledge Graph
Foundation Models: An Explainer for Non-Experts
[1hr Talk] Intro to Large Language Models
Комментарии