filmov
tv
Accelerating open shell transition metal catalyst discovery with machine learning
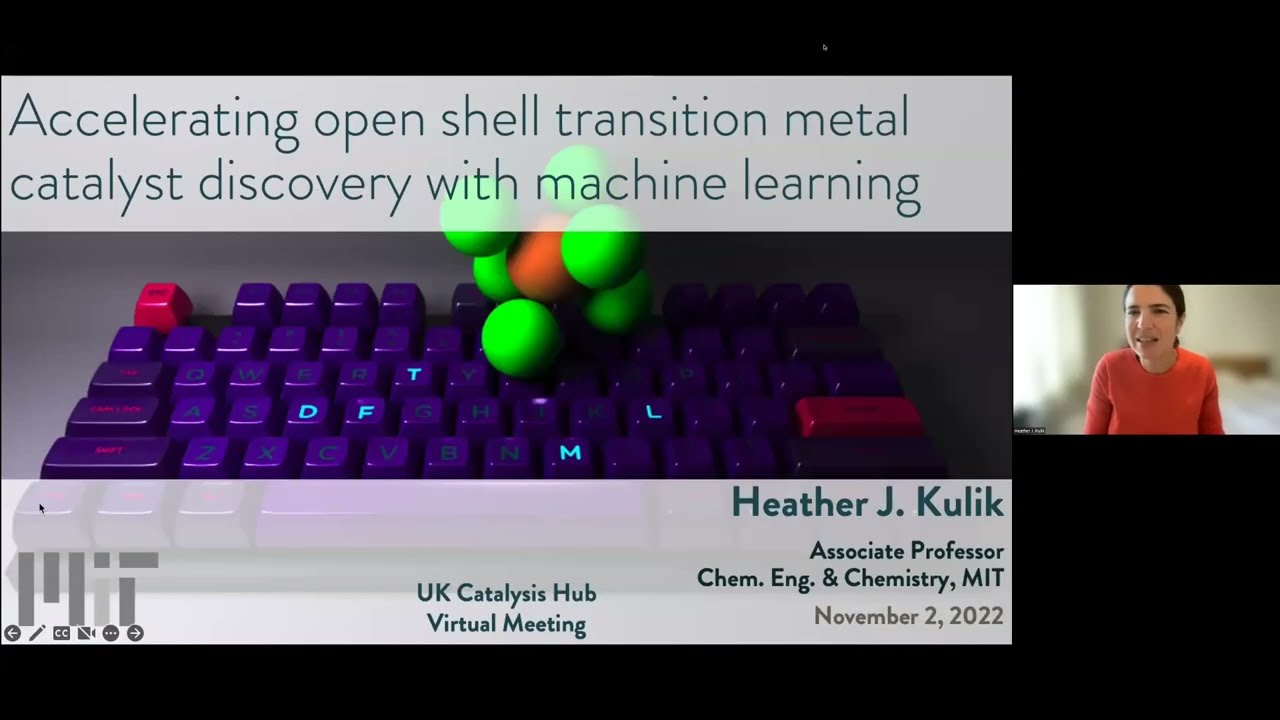
Показать описание
Talk on Accelerating open shell transition metal catalyst discovery with machine learning with Prof. Heather J. Kulik, MIT given at The UK Catalysis Hub and Centre for Rapid Online Analysis of Reactions (ROAR) meeting to discuss the impact of automation and digital chemistry on catalysis.
The vast space of all theoretical open shell transition metal catalysts challenges conventional experimentation or computation (i.e., with density functional theory, DFT). I will describe how we have built tailored representations for accelerating the prediction of open shell transition metal complex properties and subsequently extended this effort to prediction of metal-organic framework (MOF) propetrties. I will describe how we have used both high-throughput computational screening and machine learning with active learning to uncover new design principles in a space of 16 M candidate catalysts for direct methane-to-methanol conversion. With this approach, we demonstrate the determination of design principles in weeks instead of decades that traditional calculations would have required. I will describe how we have endeavored to overcome challenges of data quality (i.e., from DFT) either by learning the difference between lower and higher levels of theory or by learning directly on experimental data.
The vast space of all theoretical open shell transition metal catalysts challenges conventional experimentation or computation (i.e., with density functional theory, DFT). I will describe how we have built tailored representations for accelerating the prediction of open shell transition metal complex properties and subsequently extended this effort to prediction of metal-organic framework (MOF) propetrties. I will describe how we have used both high-throughput computational screening and machine learning with active learning to uncover new design principles in a space of 16 M candidate catalysts for direct methane-to-methanol conversion. With this approach, we demonstrate the determination of design principles in weeks instead of decades that traditional calculations would have required. I will describe how we have endeavored to overcome challenges of data quality (i.e., from DFT) either by learning the difference between lower and higher levels of theory or by learning directly on experimental data.