filmov
tv
Wiener Process - Statistics Perspective
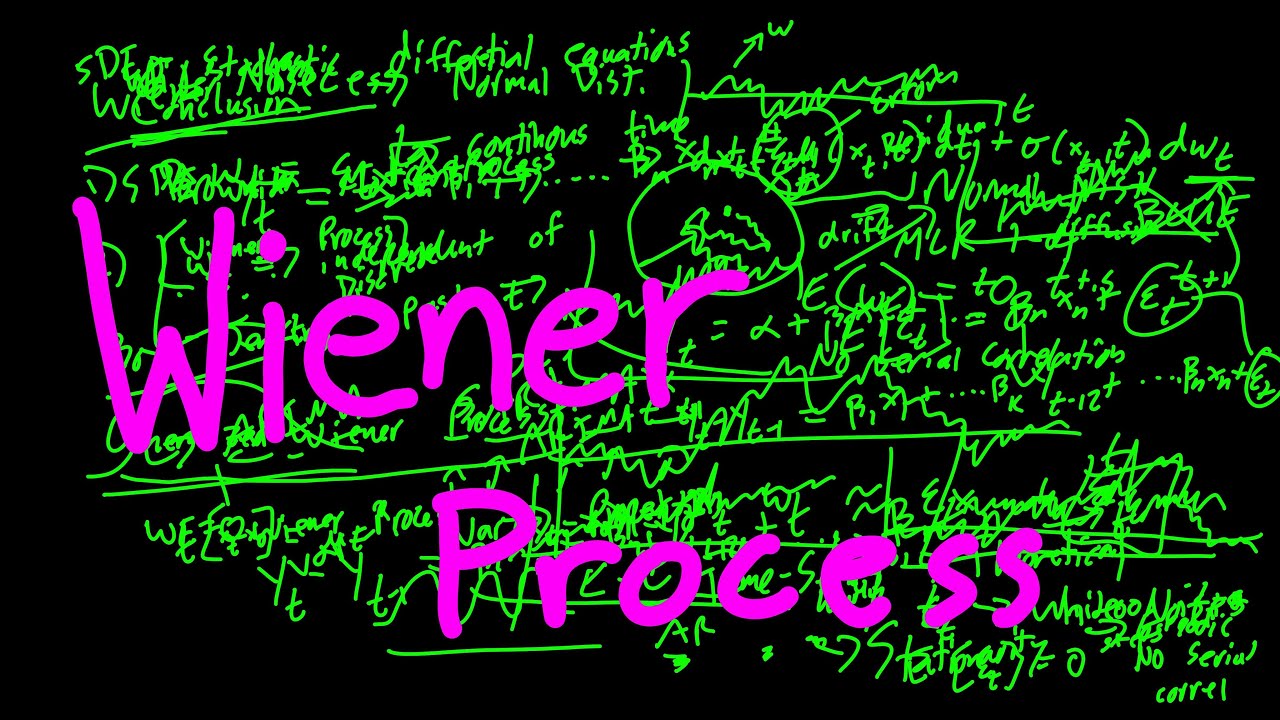
Показать описание
Quantitative finance can be a confusing area of study and the mix of math, statistics, finance, and programming makes it harder as everyone comes from a different background. In this video I explain the Wiener Process which is also known as Brownian Motion from a statistical model perspective. The residuals of a model in traditional discrete time-series models such as OLS and ARIMA have specific properties which are a zero mean and no serial correlation. These properties are known as White Noise. This white noise can be found in nature as Brownian Motion (Wiener Process). A botanist named Robert Brown discovered this as he watch a particle of pollen float around water.
In continuous time-series (stochastic models) we have a Wiener Process denoted as w(t) at the end of the equation. In many math operations it will cancel out because the mean is zero however the properties of White Noise is what makes the specification of the formulas correct. If it was just ignored and the assumptions not tested, they would be incomplete and often wrong.
Stationarity Introduction:
Stationarity Complexity Explained Using the Mandelbrot
DF and ADF Stationarity Testing
Stationarity in Finance with Applications
Probability, Stationarity, and Failures in Finance
Website:
Support:
Quant t-shirts, mugs, and hoodies:
Connect with me:
In continuous time-series (stochastic models) we have a Wiener Process denoted as w(t) at the end of the equation. In many math operations it will cancel out because the mean is zero however the properties of White Noise is what makes the specification of the formulas correct. If it was just ignored and the assumptions not tested, they would be incomplete and often wrong.
Stationarity Introduction:
Stationarity Complexity Explained Using the Mandelbrot
DF and ADF Stationarity Testing
Stationarity in Finance with Applications
Probability, Stationarity, and Failures in Finance
Website:
Support:
Quant t-shirts, mugs, and hoodies:
Connect with me:
Комментарии