filmov
tv
Modeling at Scale in Algorithmic Trading with SigOpt
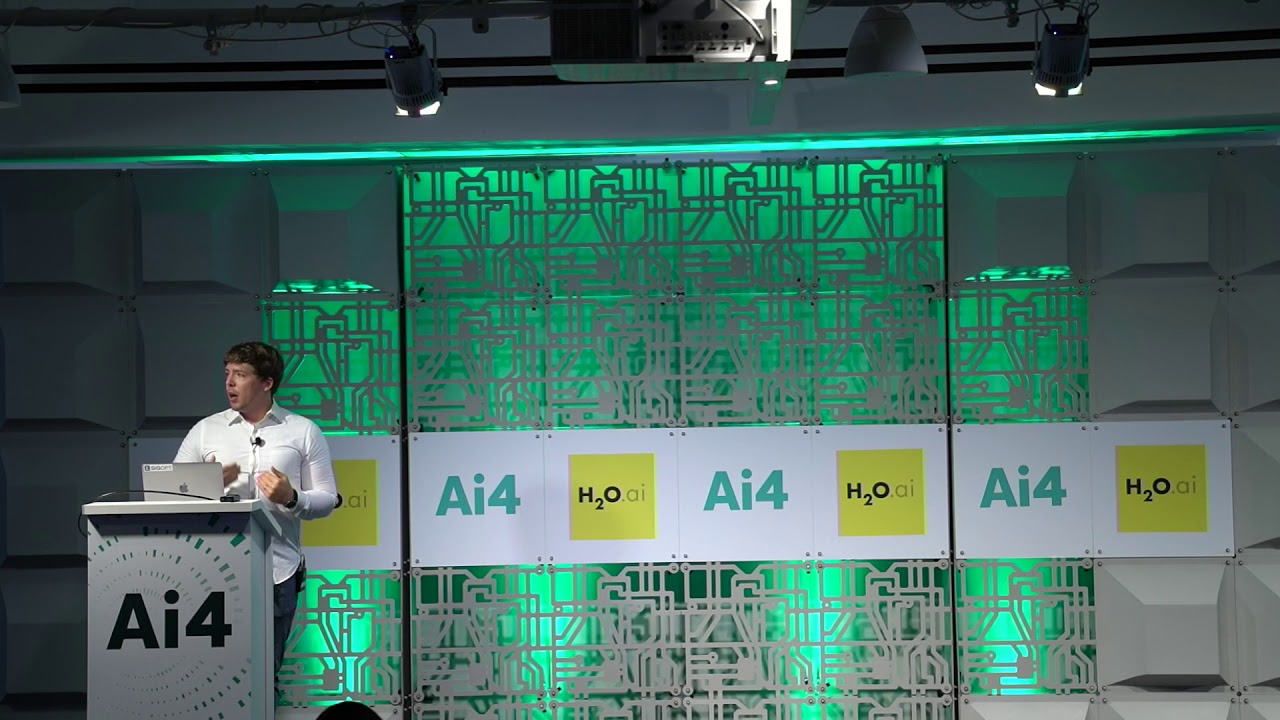
Показать описание
Scott Clark - Cofounder & CEO, SigOpt
Abstract:
Systematic trading is a trailblazer in modeling at scale. Yet even these machine learning leaders often struggle to scale their modeling processes. Far too often their most valuable asset - their researchers - waste time on modeling tasks that do not benefit from their domain expertise. Trading leaders who represent over $300 billion in assets under management rely on SigOpt to overcome this challenge by automating experimentation and optimization to build the best models. SigOpt CEO Scott Clark draws on this experience to share a set of actionable insights for teams investing in a reproducible, iterative and high-performing approach to modeling in systematic trading.
Bio:
Scott Clark, CEO & Co-Founder of SigOpt, is passionate about empowering experts to achieve their full potential with optimization solutions. He conceived of the idea for SigOpt while completing his Applied Mathematics Ph.D. at Cornell and proceeded to build an open source the Metric Optimization Engine at Yelp to help solve this problem. This process taught him that optimization needed to be productized to be effective for enterprises, which led him to found SigOpt in 2014, which has subsequently been funded by Y-Combinator, Andreessen Horowitz, Blumberg Capital, DCVC, In-Q-Tel, and others. SigOpt now helps firms and academics around the world accelerate and amplify their research through optimization in fields from machine learning to algorithmic trading and beyond. Scott holds a Ph.D. in Applied Mathematics and an MS in Computer Science from Cornell University, and BS degrees in Mathematics, Physics, and Computational Physics from Oregon State University. He was chosen as one of Forbes’ 30 under 30 in 2016.