filmov
tv
[MLOps-02] Create SageMaker Pipeline for Model Serving
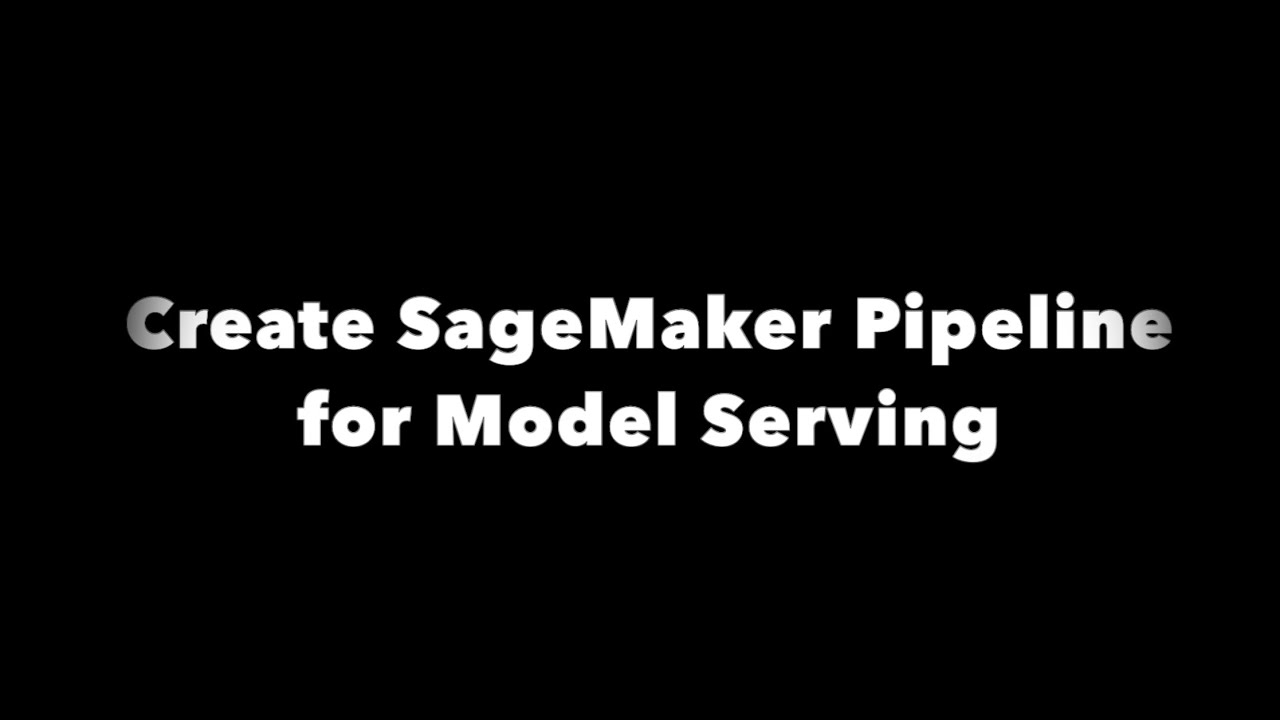
Показать описание
레고 블럭을 구성 하여 완성품 (예: 탑, 비행기, 배) 을 만들듯이, 데이터 과학자가 ML Ops 를 직접 구성하고 단계별로 만들어서 실행 할수 있을까요? 이 워크샵 에서는 (1) Amazon SageMaker Pipeline 으로 모델 훈련 및 서빙 파이프라인을 생성하는 방법, (2) CI/CD 연결을 위한 AWS CodePipeline 의 튜토리얼 실습, (3) CodePipeline 을 SageMaker Pipeline 으로 연결 하는 방법, 마지막으로 (4) 이벤트 스케줄러 생성 및 전체 과정 실행하기 를 배우게 됩니다. 이 모든 과정은 데이터 과학자에게 친숙한 쥬피터 노트북에서 진행이 됩니다. 제공된 소스 코드를 다운로드하여 본인의 모델로 레고 블럭 쌓듯이 본인의 ML Ops 를 구성해보세요
* [MLOps-00] Setup Environment for Role, SageMaker Notebook and Studio
* [MLOps-01] Create SageMaker Pipeline for Model Training
* This Step → [MLOps-02] Create SageMaker Pipeline for Model Serving
* [MLOps-03] AWS Code Series Hello World - CodeCommit, CodeBuild and CodePipeline
* [MLOps-04] Create CodePipeline Calling SageMaker Pipeline for Model Training
* [MLOps-05] Create CodePipeline Calling SageMaker Pipeline for Model Serving
* [MLOps-06] Create EventBridge Rules and Re-train as well as Re-deploy a model
* [MLOps-07] Cleanup All Resources Including SageMaker Notebook, Endpoint, and Studio Domain
(English) Just like building lego blocks to build a finished product (e.g. tower, plane, ship), can you directly construct ML Ops and build them step-by-step to streamline execution? In this session, (1),(2) how to create a model training and serving pipeline with Amazon SageMaker Pipeline, (3) a tutorial on AWS CodePipeline for CI/CD connectivity, (4),(5) CodePipeline to SageMaker You will learn how to pipeline it, and finally (6) create an event bridge rules and run the entire process. All of this process is carried out in the Jupyter notebook. After learning this workshop, please try to apply your data and model to this workshop code which is as above.
* [MLOps-00] Setup Environment for Role, SageMaker Notebook and Studio
* [MLOps-01] Create SageMaker Pipeline for Model Training
* This Step → [MLOps-02] Create SageMaker Pipeline for Model Serving
* [MLOps-03] AWS Code Series Hello World - CodeCommit, CodeBuild and CodePipeline
* [MLOps-04] Create CodePipeline Calling SageMaker Pipeline for Model Training
* [MLOps-05] Create CodePipeline Calling SageMaker Pipeline for Model Serving
* [MLOps-06] Create EventBridge Rules and Re-train as well as Re-deploy a model
* [MLOps-07] Cleanup All Resources Including SageMaker Notebook, Endpoint, and Studio Domain
(English) Just like building lego blocks to build a finished product (e.g. tower, plane, ship), can you directly construct ML Ops and build them step-by-step to streamline execution? In this session, (1),(2) how to create a model training and serving pipeline with Amazon SageMaker Pipeline, (3) a tutorial on AWS CodePipeline for CI/CD connectivity, (4),(5) CodePipeline to SageMaker You will learn how to pipeline it, and finally (6) create an event bridge rules and run the entire process. All of this process is carried out in the Jupyter notebook. After learning this workshop, please try to apply your data and model to this workshop code which is as above.