filmov
tv
Andrew Ng’s Tips for the Data-Centric AI Future
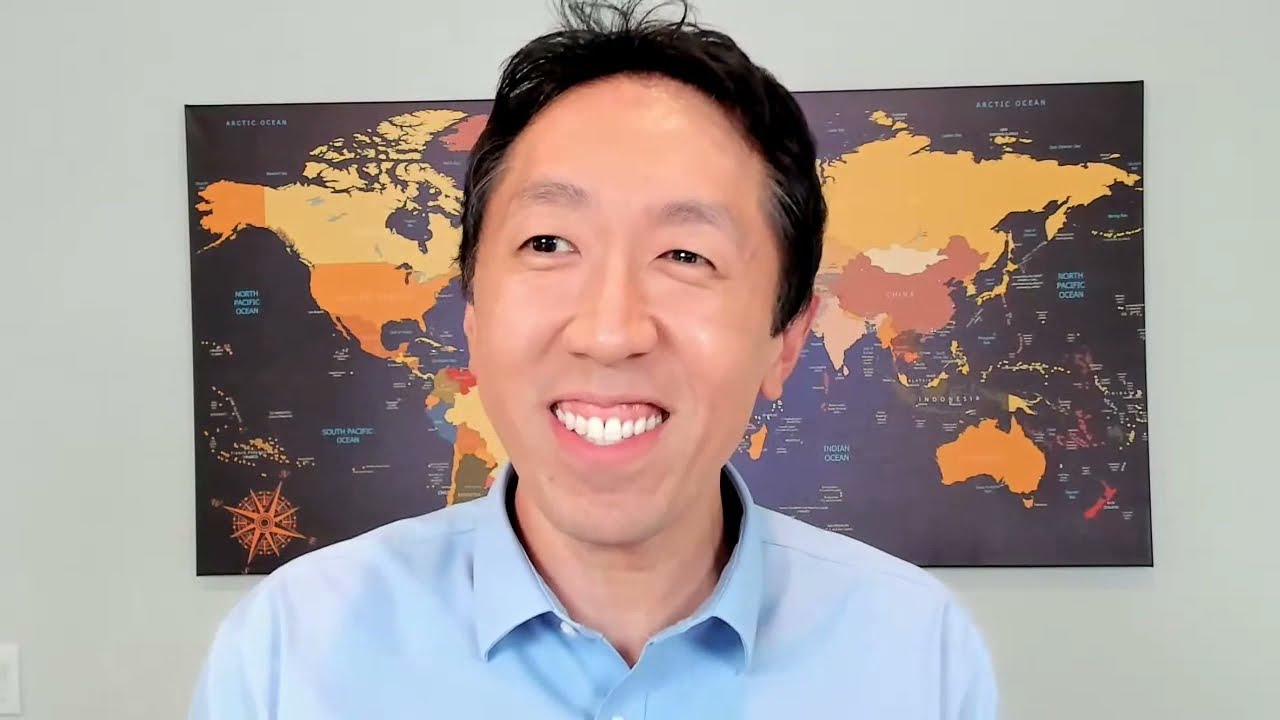
Показать описание
Embark on a journey into the future of AI with Andrew Ng, the renowned pioneer in machine learning and online education, as he explains the paradigm shift towards data-centric AI development and its implications for the industry. Learn about the evolution of AI and the crucial role of data engineering in enhancing machine learning systems. Discover practical tips for optimizing data quality, improving model performance, and accelerating the AI development process.
Timestamps:
00:00 Introduction
00:40 Acknowledgment of Previous Collaborations
01:07 Overview of Data Centric AI
02:32 Discussion on the Rise of Data Centric AI
05:08 Top Five Tips for Data Centric AI Development
05:54 Tip 1: Ensure Consistent Labels
08:13 Tip 2: Use Multiple Labelers
10:30 Tip 3: Analyze Bad Examples
11:23 Tip 4: More Data is Not Always Better
11:29 Tip 5: Focus on Error Analysis
13:19 Clarifications on Data Centric AI
14:01 Summary of Key Points
15:20 Shifts in AI Development
16:30 Invitation for Questions
17:02 Audience Q&A
20:00 Addressing Noisy Examples
22:11 Practicality of Data Centric AI in Resource-Limited Scenarios
24:22 Trade-Offs in Label Cleaning vs. Data Gathering
26:01 Tips for Structured Data
27:40 Conclusion and Further Engagement
#andrewng #datacentricai #machinelearning
Timestamps:
00:00 Introduction
00:40 Acknowledgment of Previous Collaborations
01:07 Overview of Data Centric AI
02:32 Discussion on the Rise of Data Centric AI
05:08 Top Five Tips for Data Centric AI Development
05:54 Tip 1: Ensure Consistent Labels
08:13 Tip 2: Use Multiple Labelers
10:30 Tip 3: Analyze Bad Examples
11:23 Tip 4: More Data is Not Always Better
11:29 Tip 5: Focus on Error Analysis
13:19 Clarifications on Data Centric AI
14:01 Summary of Key Points
15:20 Shifts in AI Development
16:30 Invitation for Questions
17:02 Audience Q&A
20:00 Addressing Noisy Examples
22:11 Practicality of Data Centric AI in Resource-Limited Scenarios
24:22 Trade-Offs in Label Cleaning vs. Data Gathering
26:01 Tips for Structured Data
27:40 Conclusion and Further Engagement
#andrewng #datacentricai #machinelearning
Комментарии