filmov
tv
(Large) Language models for accelerated chemical discovery and synthesis
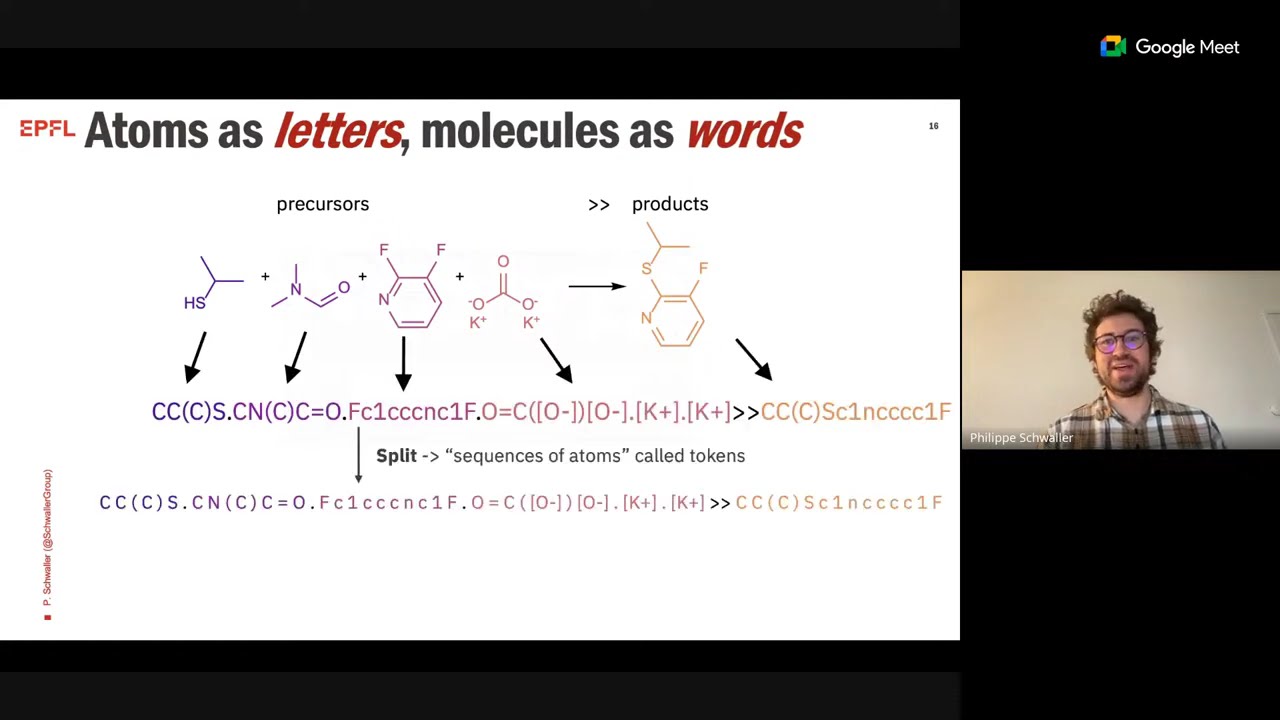
Показать описание
Philippe Schwaller, EPFL
Abstract:
AI-accelerated synthesis is an emerging field that uses machine learning algorithms to improve the efficiency and productivity of chemical and materials synthesis. Modern machine learning models, such as (large) language models, can capture the knowledge hidden in large chemical databases to rapidly design and discover new compounds, predict the outcome of reactions, and help optimize chemical reactions. One of the key advantages of AI-accelerated synthesis is its ability to make vast chemical data accessible and predict promising candidate synthesis paths, potentially leading to breakthrough discoveries. Overall, AI is poised to revolutionize the field of organic synthesis, enabling faster and more efficient drug development, catalysis, and other applications.
Bio:
Philippe Schwaller joined EPFL as a tenure-track assistant professor in the Institute of Chemical Sciences and Engineering in February 2022. He leads the Laboratory of Artificial Chemical Intelligence, which works on AI-accelerated discovery and synthesis of molecules. Philippe is a core PI of the NCCR Catalysis, a Swiss centre for sustainable chemistry research, education, and innovation, and a co-lead of the foundation models for sciences pillar in the Swiss AI initiative. He belongs to a new generation of scientists with a broad set of skills – in his case, a combination of chemistry, materials science, computer science, and experimental research.
Before EPFL, Philippe worked for five years at IBM Research. He simultaneously completed an MPhil in Physics (University of Cambridge) and a PhD in Chemistry and Molecular Sciences (University of Bern). He also holds a BSc and MSc degree in Materials Science and Engineering (EPFL).
#modeling #simulation #ai #ml #research #llm #chemistry #sciml #organicchemistry #chemicalsynthesis #materialsscience #materialdesign #drugdevelopment
Abstract:
AI-accelerated synthesis is an emerging field that uses machine learning algorithms to improve the efficiency and productivity of chemical and materials synthesis. Modern machine learning models, such as (large) language models, can capture the knowledge hidden in large chemical databases to rapidly design and discover new compounds, predict the outcome of reactions, and help optimize chemical reactions. One of the key advantages of AI-accelerated synthesis is its ability to make vast chemical data accessible and predict promising candidate synthesis paths, potentially leading to breakthrough discoveries. Overall, AI is poised to revolutionize the field of organic synthesis, enabling faster and more efficient drug development, catalysis, and other applications.
Bio:
Philippe Schwaller joined EPFL as a tenure-track assistant professor in the Institute of Chemical Sciences and Engineering in February 2022. He leads the Laboratory of Artificial Chemical Intelligence, which works on AI-accelerated discovery and synthesis of molecules. Philippe is a core PI of the NCCR Catalysis, a Swiss centre for sustainable chemistry research, education, and innovation, and a co-lead of the foundation models for sciences pillar in the Swiss AI initiative. He belongs to a new generation of scientists with a broad set of skills – in his case, a combination of chemistry, materials science, computer science, and experimental research.
Before EPFL, Philippe worked for five years at IBM Research. He simultaneously completed an MPhil in Physics (University of Cambridge) and a PhD in Chemistry and Molecular Sciences (University of Bern). He also holds a BSc and MSc degree in Materials Science and Engineering (EPFL).
#modeling #simulation #ai #ml #research #llm #chemistry #sciml #organicchemistry #chemicalsynthesis #materialsscience #materialdesign #drugdevelopment