filmov
tv
Brain Machine Interface used by monkey to drive in virtual reality: task specific neural coding
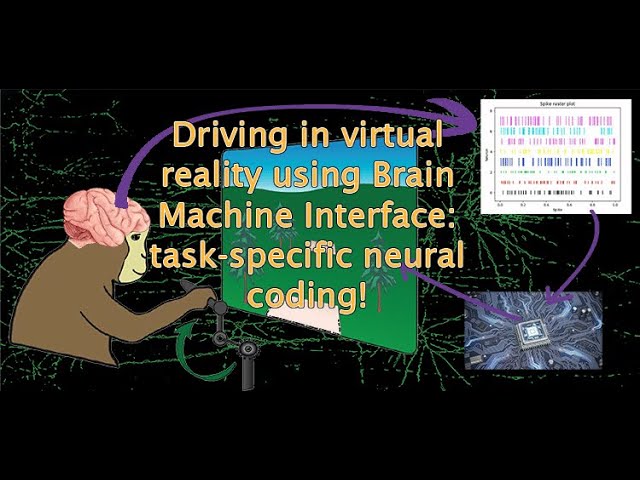
Показать описание
Elon Musk seeks to make us all super human cyborgs using brain machine interfaces (BMIs) developed by his company Neuralink. Science fiction is becoming reality, but neuroscience keeps revealing new complexity in the brain's signals. In this video, I tell you about an exciting new finding that the brain uses different neural coding for each different task it engages in. This means that to make humans into cyborgs with super human abilities, Musk and Neuralink will need to program a different algorithm for every task that a person might want to use their brain machine interface to achieve.
More specifically, in this video, I describe a recent paper published in the Journal of Neuroscience by Karen Shroeder, Sean Perkins, Qi Wang, and Mark Churchland. In the paper, they describe how they taught monkeys to navigate a simple virtual reality environment using the rotation of a hand pedal. Then, once the monkeys had learned to be successful drivers, the researchers trained a computer to decode the monkeys' brain signals and use those to control the virtual reality game. The decoding was so good that the monkeys barely noticed they weren't driving with their hands anymore.
How did the researchers manage to do such a flawless decoding of the brain's signal? In past research, other scientists have used simple linear decoders. This is the type of decoder that Elon Musk's company Neuralink is planning on using (as revealed in their blog and video publications). The basic idea is that each neuron in the hand area of the motor cortex codes for some aspect of hand motion. If scientists record from a bunch of neurons, then they can look for correlations between neuron firing rates and variables of hand motion (like x-direction position or y-direction speed). Then, the combined information from many neurons can be used to build up an idea of where the brain is trying to make the hand move. This style of decoder didn't work very well to let the monkey drive in virtual reality.
It turned out that far better decoding could be achieved if the researchers designed a bespoke algorithm that utilized as much of the variance in the neural signal as possible. The variance in a signal is where the information is contained. If you walk up to someone and just say, "ahhhhhhhhhhhhhhhhhhhhh....", then you won't communicate much. It's in the variance of your voice that you encode information. The same is true of neural signals. What the researchers here figured out is that the variance in neural signals doesn't always follow the variables we think it will. For example, they found that their decoder worked best when it had separate elements decoding backward versus forward motion. The actual position of the hand wasn't directly represented anywhere. This was very different from the type of linear 1-1 mapping of neural signal to arm motion that's been used in past research or that is being used by Neuralink. This difference implies that the brain changes its neural code for every task it's faced with!
It's wild to think that the brain can change with every task, but it makes some sense. It helps avoid distraction. It's like having a dedicated communication channel for every specific need. It's going to be a nightmare for Musk and Neuralink and anyone else who wants to develop general purpose brain machine interfaces, but Shroeder et al. have shown us a way forward: you've got to follow the brain's variance rather than assuming you know what it's coding for a priori.
paper described in video: 10.1523/JNEUROSCI.2687-20.2021
Connect with me
_____________________________
Twitter:
_____________________________
Patreon:
_____________________________
More specifically, in this video, I describe a recent paper published in the Journal of Neuroscience by Karen Shroeder, Sean Perkins, Qi Wang, and Mark Churchland. In the paper, they describe how they taught monkeys to navigate a simple virtual reality environment using the rotation of a hand pedal. Then, once the monkeys had learned to be successful drivers, the researchers trained a computer to decode the monkeys' brain signals and use those to control the virtual reality game. The decoding was so good that the monkeys barely noticed they weren't driving with their hands anymore.
How did the researchers manage to do such a flawless decoding of the brain's signal? In past research, other scientists have used simple linear decoders. This is the type of decoder that Elon Musk's company Neuralink is planning on using (as revealed in their blog and video publications). The basic idea is that each neuron in the hand area of the motor cortex codes for some aspect of hand motion. If scientists record from a bunch of neurons, then they can look for correlations between neuron firing rates and variables of hand motion (like x-direction position or y-direction speed). Then, the combined information from many neurons can be used to build up an idea of where the brain is trying to make the hand move. This style of decoder didn't work very well to let the monkey drive in virtual reality.
It turned out that far better decoding could be achieved if the researchers designed a bespoke algorithm that utilized as much of the variance in the neural signal as possible. The variance in a signal is where the information is contained. If you walk up to someone and just say, "ahhhhhhhhhhhhhhhhhhhhh....", then you won't communicate much. It's in the variance of your voice that you encode information. The same is true of neural signals. What the researchers here figured out is that the variance in neural signals doesn't always follow the variables we think it will. For example, they found that their decoder worked best when it had separate elements decoding backward versus forward motion. The actual position of the hand wasn't directly represented anywhere. This was very different from the type of linear 1-1 mapping of neural signal to arm motion that's been used in past research or that is being used by Neuralink. This difference implies that the brain changes its neural code for every task it's faced with!
It's wild to think that the brain can change with every task, but it makes some sense. It helps avoid distraction. It's like having a dedicated communication channel for every specific need. It's going to be a nightmare for Musk and Neuralink and anyone else who wants to develop general purpose brain machine interfaces, but Shroeder et al. have shown us a way forward: you've got to follow the brain's variance rather than assuming you know what it's coding for a priori.
paper described in video: 10.1523/JNEUROSCI.2687-20.2021
Connect with me
_____________________________
Twitter:
_____________________________
Patreon:
_____________________________
Комментарии