filmov
tv
AI Agents: Looping vs Planning
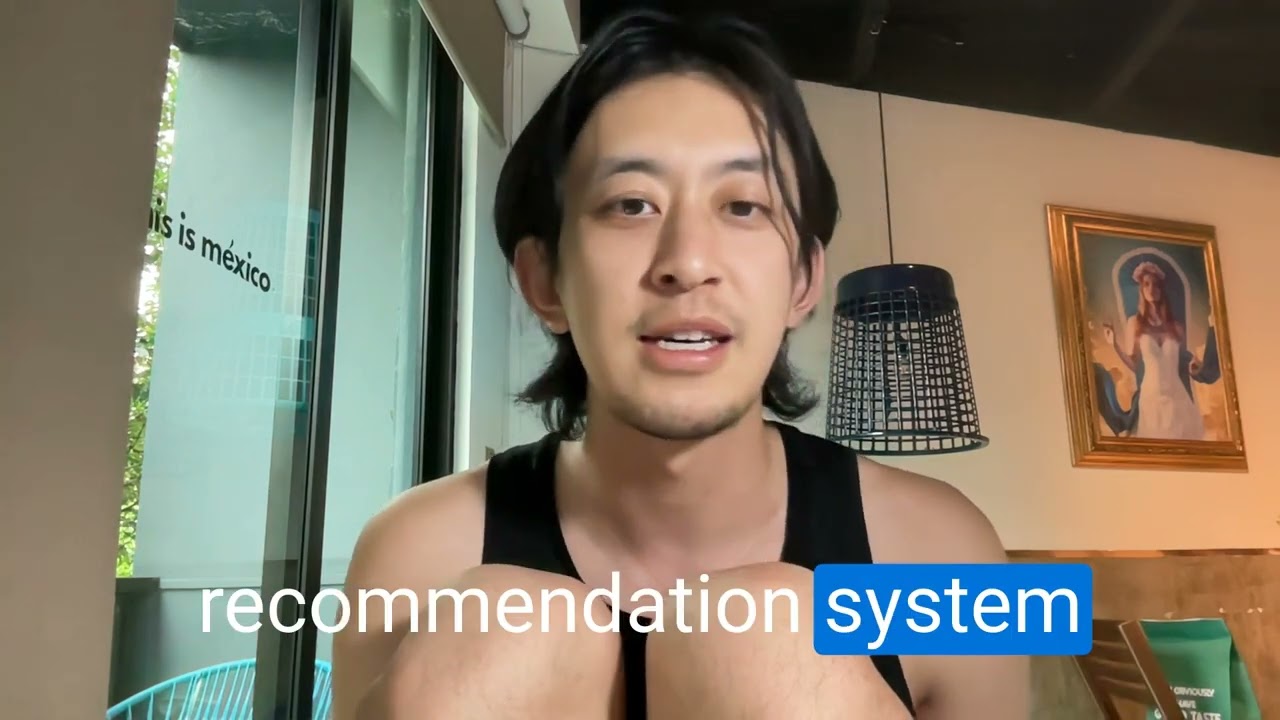
Показать описание
Here is a cleaned up version of the text, maintaining the original tone and key points:
Today, I want to discuss the ideas around looping versus planning agents. The react paper is well-known, where you have a train of thought, access to tools, and loop through thinking, using tools, and reasoning about next steps. While this is cool, it becomes more complicated and less reproducible for real-world applications beyond simple academic examples. Few-shot examples are hard to capture as inbound requests, number of tools, and feedback for improvement are unclear.
Looping may not be perfect, so the goal is to propose solutions that use more plans and tags while still reasoning about them in a fuzzy way. I think in terms of inputs and outputs, even with instructor models. With an agent, the output data structure should be a deterministically executable plan. We can fine-tune a model that takes requests and produces the correct plan.
Here's how we can do it:
1. Predict all necessary tools given a request, possibly using multiple hops and a recommendation system based on similar and complementary tools. There will be precision and recall trade-offs.
2. Given the request, retrieved tools, and their descriptions/instructions, generate an execution plan (DAG). The conversation iteratively changes the plan.
3. Fine-tune a model that takes inputs and tools to predict the final plan, assuming modifications don't change much.
4. Retrieve examples of successfully running plans given the request and tools to hydrate the prompt with few-shot examples of sophisticated plans.
5. If the plan is too complex to generate with fully implemented edges, implement individual edges by transitioning from one node to another's inputs using a react loop and few-shot examples.
The idea is to produce the entire plan separately from its execution, with the plan's construction being probabilistic rather than its execution. The goal is to produce artifacts for retrieval to create more few-shot examples, leaving a single artifact at the end of each conversation. This allows fine-tuning models to predict the output correctly in a single shot, essentially compiling the system.
Today, I want to discuss the ideas around looping versus planning agents. The react paper is well-known, where you have a train of thought, access to tools, and loop through thinking, using tools, and reasoning about next steps. While this is cool, it becomes more complicated and less reproducible for real-world applications beyond simple academic examples. Few-shot examples are hard to capture as inbound requests, number of tools, and feedback for improvement are unclear.
Looping may not be perfect, so the goal is to propose solutions that use more plans and tags while still reasoning about them in a fuzzy way. I think in terms of inputs and outputs, even with instructor models. With an agent, the output data structure should be a deterministically executable plan. We can fine-tune a model that takes requests and produces the correct plan.
Here's how we can do it:
1. Predict all necessary tools given a request, possibly using multiple hops and a recommendation system based on similar and complementary tools. There will be precision and recall trade-offs.
2. Given the request, retrieved tools, and their descriptions/instructions, generate an execution plan (DAG). The conversation iteratively changes the plan.
3. Fine-tune a model that takes inputs and tools to predict the final plan, assuming modifications don't change much.
4. Retrieve examples of successfully running plans given the request and tools to hydrate the prompt with few-shot examples of sophisticated plans.
5. If the plan is too complex to generate with fully implemented edges, implement individual edges by transitioning from one node to another's inputs using a react loop and few-shot examples.
The idea is to produce the entire plan separately from its execution, with the plan's construction being probabilistic rather than its execution. The goal is to produce artifacts for retrieval to create more few-shot examples, leaving a single artifact at the end of each conversation. This allows fine-tuning models to predict the output correctly in a single shot, essentially compiling the system.
Комментарии