filmov
tv
Vadim Sokolov (GMU) Deep Learning A Bayesian Perspective
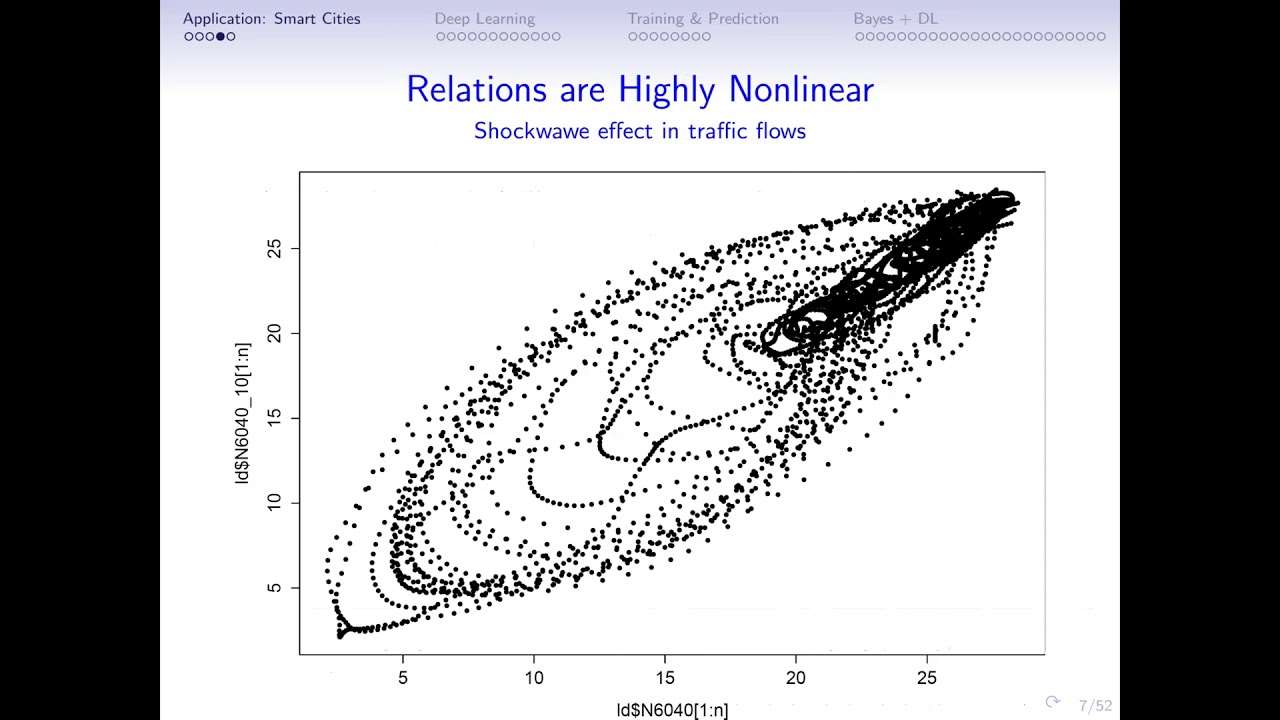
Показать описание
Abstract:
ABSTRACT
Deep learning is a form of machine learning for nonlinear high dimensional pattern matching and prediction. By taking a Bayesian probabilistic perspective, we provide a number of insights into more efficient algorithms for optimisation and hyper-parameter tuning. Traditional high-dimensional data reduction techniques, such as principal component analysis (PCA), partial least squares (PLS), reduced rank regression (RRR), projection pursuit regression (PPR) are all shown to be shallow learners. Their deep learning counterparts exploit multiple deep layers of data reduction which provide predictive performance gains. Stochastic gradient descent (SGD) training optimisation and Dropout (DO) regularization provide estimation and variable selection. Bayesian regularization is central to finding weights and connections in networks to optimize
the predictive bias-variance trade-off.
Bio:
Vadim Sokolov is an assistant professor in the Systems Engineering and Operations Research Department at George Mason University. He works on building robust solutions for large scale complex system analysis, at the interface of simulation-based modeling and statistics. This involves, developing new methodologies that rely on deep learning, Bayesian analysis, design of computational experiments and development of open-source software that implements those methodologies. Inspired by an interest in urban systems he co-developed mobility simulator called Polaris that is currently used for large scale transportation networks analysis by both local and federal governments. Prior to joining GMU he was a principal computational scientist at Argonne National Laboratory, a fellow at the Computation Institute at the University of Chicago and lecturer at the Master of Science in Analytics program at the University of Chicago.
ABSTRACT
Deep learning is a form of machine learning for nonlinear high dimensional pattern matching and prediction. By taking a Bayesian probabilistic perspective, we provide a number of insights into more efficient algorithms for optimisation and hyper-parameter tuning. Traditional high-dimensional data reduction techniques, such as principal component analysis (PCA), partial least squares (PLS), reduced rank regression (RRR), projection pursuit regression (PPR) are all shown to be shallow learners. Their deep learning counterparts exploit multiple deep layers of data reduction which provide predictive performance gains. Stochastic gradient descent (SGD) training optimisation and Dropout (DO) regularization provide estimation and variable selection. Bayesian regularization is central to finding weights and connections in networks to optimize
the predictive bias-variance trade-off.
Bio:
Vadim Sokolov is an assistant professor in the Systems Engineering and Operations Research Department at George Mason University. He works on building robust solutions for large scale complex system analysis, at the interface of simulation-based modeling and statistics. This involves, developing new methodologies that rely on deep learning, Bayesian analysis, design of computational experiments and development of open-source software that implements those methodologies. Inspired by an interest in urban systems he co-developed mobility simulator called Polaris that is currently used for large scale transportation networks analysis by both local and federal governments. Prior to joining GMU he was a principal computational scientist at Argonne National Laboratory, a fellow at the Computation Institute at the University of Chicago and lecturer at the Master of Science in Analytics program at the University of Chicago.