filmov
tv
Deep Learning and Computations of High Dimensional Partial Differential Equations (PDEs)
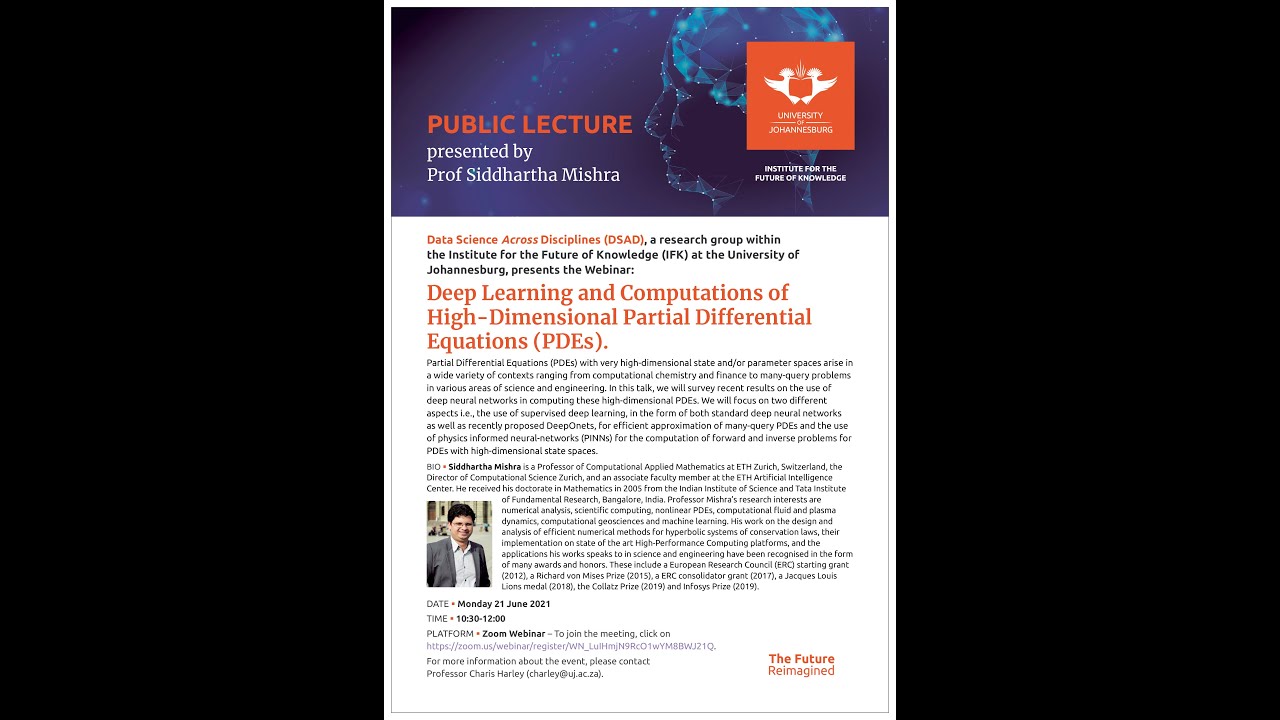
Показать описание
Partial Differential Equations (PDEs) with very high-dimensional state and/or parameter spaces arise in a wide variety of contexts ranging from computational chemistry and finance to many-query problems in various areas of science and engineering. In this talk, we will survey recent results on the use of deep neural networks in computing these high-dimensional PDEs. We will focus on two different aspects i.e., the use of supervised deep learning, in the form of both standard deep neural networks as well as recently proposed DeepOnets, for efficient approximation of many-query PDEs and the use of physics informed neural-networks (PINNs) for the computation of forward and inverse problems for PDEs with high-dimensional state spaces
The speaker is Professor Siddhartha Mishra. He is a Professor of Computational Applied Mathematics at ETH Zurich, Switzerland, the Director of Computational Science Zurich, and an associate faculty member at the ETH Artificial Intelligence Center. He received his doctorate in Mathematics in 2005 from the Indian Institute of Science and Tata Institute of Fundamental Research, Bangalore, India. Professor Mishra’s research interests are numerical analysis, scientific computing, nonlinear PDEs, computational fluid and plasma dynamics, computational geosciences and machine learning. His work on the design and analysis of efficient numerical methods for hyperbolic systems of conservation laws, their implementation on state of the art High-Performance Computing platforms, and the applications his works speaks to in science and engineering have been recognised in the form of many awards and honors. These include a European Research Council (ERC) starting grant (2012), a Richard von Mises Prize (2015), a ERC consolidator grant (2017), a Jacques Louis Lions medal (2018), the Collatz Prize (2019) and Infosys Prize (2019).
The speaker is Professor Siddhartha Mishra. He is a Professor of Computational Applied Mathematics at ETH Zurich, Switzerland, the Director of Computational Science Zurich, and an associate faculty member at the ETH Artificial Intelligence Center. He received his doctorate in Mathematics in 2005 from the Indian Institute of Science and Tata Institute of Fundamental Research, Bangalore, India. Professor Mishra’s research interests are numerical analysis, scientific computing, nonlinear PDEs, computational fluid and plasma dynamics, computational geosciences and machine learning. His work on the design and analysis of efficient numerical methods for hyperbolic systems of conservation laws, their implementation on state of the art High-Performance Computing platforms, and the applications his works speaks to in science and engineering have been recognised in the form of many awards and honors. These include a European Research Council (ERC) starting grant (2012), a Richard von Mises Prize (2015), a ERC consolidator grant (2017), a Jacques Louis Lions medal (2018), the Collatz Prize (2019) and Infosys Prize (2019).