filmov
tv
1st webinar | A. Giovannucci: Neuro-image segmentation and real-time data processing
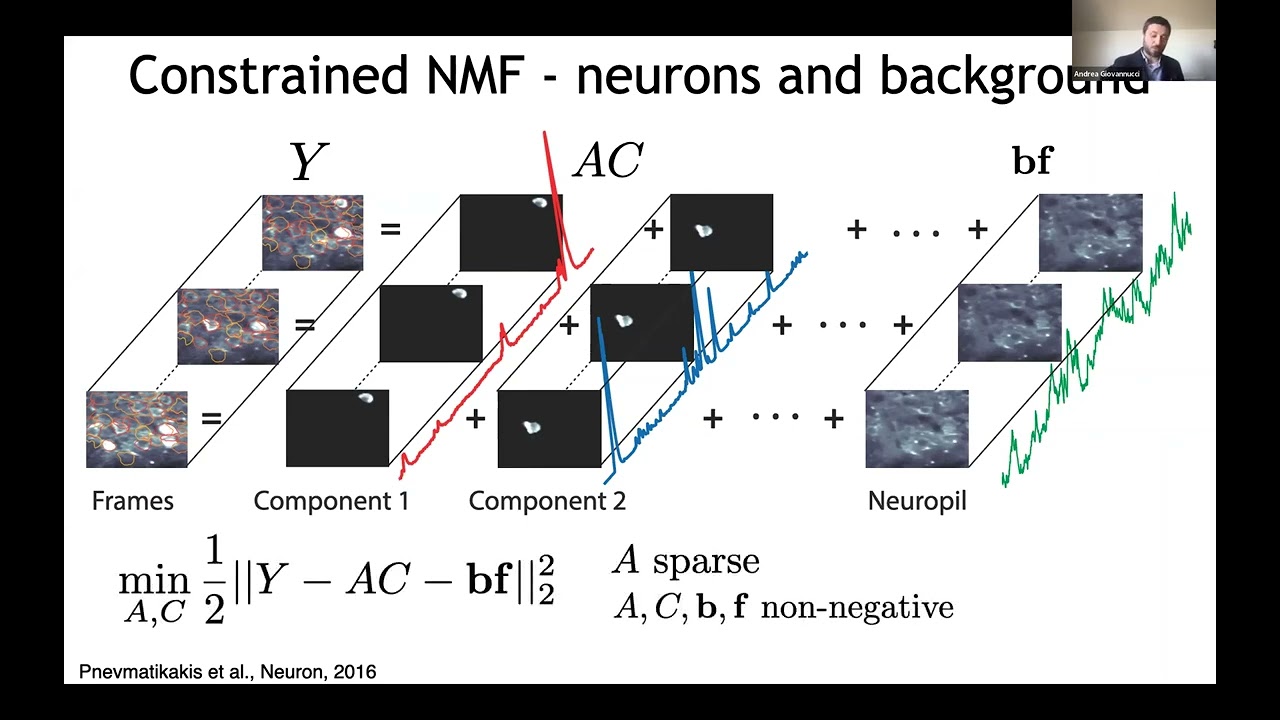
Показать описание
BIO
Dr. Giovannucci obtained his PhD from the Artificial Intelligence Research Institute of Barcelona. His postdoctoral work spanned computational neuroscience, neuroprosthetics, and experimental neuroscience at Princeton University and Pompeu Fabra University. Dr. Giovannucci subsequently took on a data scientist position at the Flatiron Institute (Simons Foundation) to develop machine learning algorithms and open-source software for the analysis of calcium imaging data. Dr. Giovannucci is currently an Assistant Professor in Neural Engineering at the UNC/NCSU department of Bioengineering, the Closed-loop Engineering for Advanced Rehabilitation center (CLEAR) and the UNC Neuroscience Center. The current research interests of the laboratory he leads focus on the analysis of biomedical and neuroscience imaging data, including calcium and voltage imaging at cellular level. Dr. Giovannucci is a Beckman Young Investigator, and formerly was a New Jersey Commission on Brain Injury Research and a Juan de la Cierva postdoctoral fellow. His research is funded by the Chan Zuckerberg Initiative, The Kavli foundation, NIH and the Beckman Foundation.
ABSTRACT
Optical microscopy methods such as calcium and voltage imaging already enable fast activity readout (30-1000Hz) of large neuronal populations using light. However, the lack of corresponding advances in online algorithms has slowed progress in retrieving information about neural activity during or shortly after an experiment. This technological gap not only prevents the execution of novel real-time closed-loop experiments, but also hampers fast experiment-analysis-theory turnover for high-throughput imaging modalities. The fundamental challenge is to reliably extract neural activity from fluorescence imaging frames at speeds compatible with new indicator dynamics and imaging modalities. To meet these challenges and requirements, we propose a framework for Fluorescence Imaging OnLine Analysis (FIOLA). FIOLA exploits computational graphs and accelerated hardware to preprocess fluorescence imaging movies and extract fluorescence traces at speeds in excess of 300Hz on calcium imaging datasets and at speeds over 400Hz on voltage imaging datasets. Additionally, we present the first real-time spike extraction algorithm for voltage imaging data. We evaluate FIOLA on both simulated data and real data, demonstrating reliable and scalable performance. Our methods provide the computational substrate required to precisely interface large neuronal populations and machines in real-time, enabling new applications in neuroprosthetics, brain-machine interfaces, and experimental neuroscience. Moreover, this new set of tools is poised to dramatically shorten the experiment-data-theory cycle by providing immediate feedback on the activity of large neuronal populations at experimental time.
Dr. Giovannucci obtained his PhD from the Artificial Intelligence Research Institute of Barcelona. His postdoctoral work spanned computational neuroscience, neuroprosthetics, and experimental neuroscience at Princeton University and Pompeu Fabra University. Dr. Giovannucci subsequently took on a data scientist position at the Flatiron Institute (Simons Foundation) to develop machine learning algorithms and open-source software for the analysis of calcium imaging data. Dr. Giovannucci is currently an Assistant Professor in Neural Engineering at the UNC/NCSU department of Bioengineering, the Closed-loop Engineering for Advanced Rehabilitation center (CLEAR) and the UNC Neuroscience Center. The current research interests of the laboratory he leads focus on the analysis of biomedical and neuroscience imaging data, including calcium and voltage imaging at cellular level. Dr. Giovannucci is a Beckman Young Investigator, and formerly was a New Jersey Commission on Brain Injury Research and a Juan de la Cierva postdoctoral fellow. His research is funded by the Chan Zuckerberg Initiative, The Kavli foundation, NIH and the Beckman Foundation.
ABSTRACT
Optical microscopy methods such as calcium and voltage imaging already enable fast activity readout (30-1000Hz) of large neuronal populations using light. However, the lack of corresponding advances in online algorithms has slowed progress in retrieving information about neural activity during or shortly after an experiment. This technological gap not only prevents the execution of novel real-time closed-loop experiments, but also hampers fast experiment-analysis-theory turnover for high-throughput imaging modalities. The fundamental challenge is to reliably extract neural activity from fluorescence imaging frames at speeds compatible with new indicator dynamics and imaging modalities. To meet these challenges and requirements, we propose a framework for Fluorescence Imaging OnLine Analysis (FIOLA). FIOLA exploits computational graphs and accelerated hardware to preprocess fluorescence imaging movies and extract fluorescence traces at speeds in excess of 300Hz on calcium imaging datasets and at speeds over 400Hz on voltage imaging datasets. Additionally, we present the first real-time spike extraction algorithm for voltage imaging data. We evaluate FIOLA on both simulated data and real data, demonstrating reliable and scalable performance. Our methods provide the computational substrate required to precisely interface large neuronal populations and machines in real-time, enabling new applications in neuroprosthetics, brain-machine interfaces, and experimental neuroscience. Moreover, this new set of tools is poised to dramatically shorten the experiment-data-theory cycle by providing immediate feedback on the activity of large neuronal populations at experimental time.