filmov
tv
Sofia Vallecorsa: Quantum Machine Learning in High Energy Physics
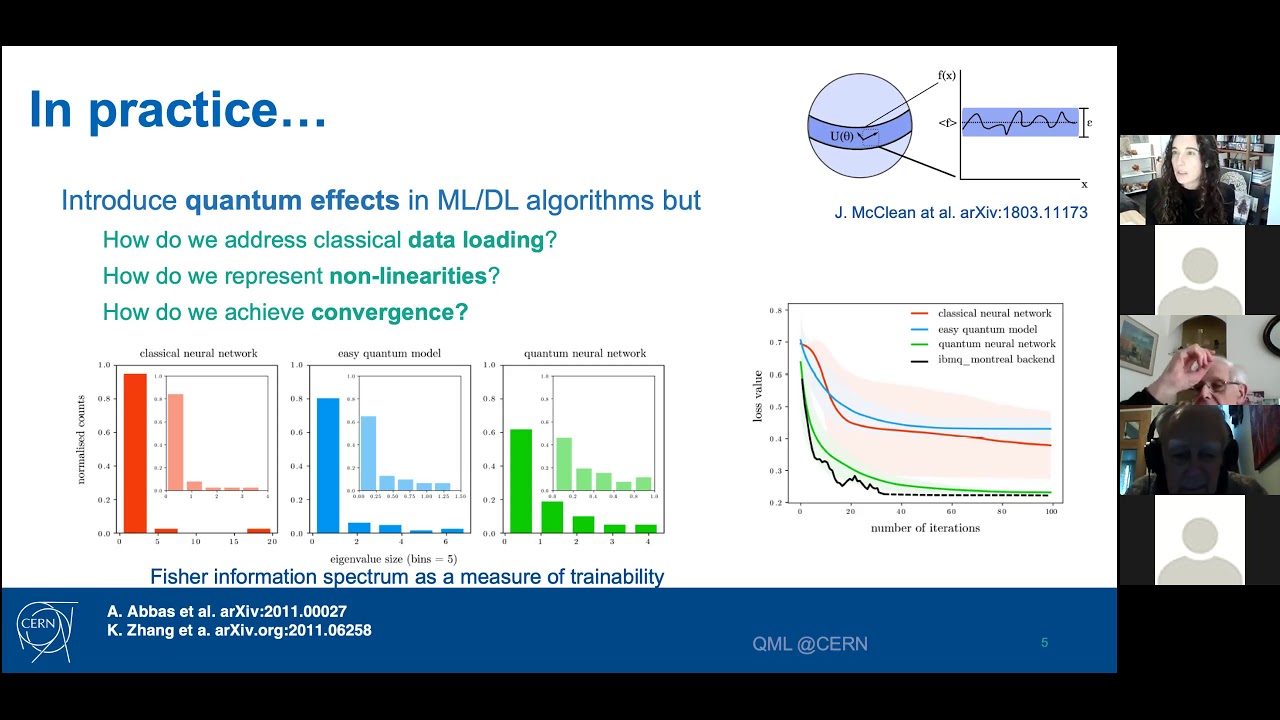
Показать описание
The High Energy Physics community has a long tradition of using machine learning to solve specific tasks, in particular related to a more efficient selection of interesting events over the overwhelming background produced at colliders such as the LHC. In the recent years, several studies have demonstrated the benefit of using deep learning techniques and building on these examples, many High Energy Physics experiments are now working on integrating deep learning into their workflows for different applications: from pattern recognition, to real-time selection of interesting collision events, to simulation and data analysis. At the same time, quantum computing represents a most promising breakthrough in computing technology. Today’s hardware has not yet reached the level at which it could be put into production but the number of R&D activities in terms of hardware, programming platforms, simulators and applications increases both in the academic world and industry. Given both the potential and the uncertainty surrounding this domain, it is important to understand which of our activities could most benefit from quantum computing algorithms. In this context, CERN has created a Quantum Technology Initiative to understand the potential of quantum technologies for High Energy Physics. This talk highlights the first generation of ideas that use quantum machine learning on problems in High Energy Physics and provide an outlook on future applications.