filmov
tv
Mastering Retrieval for LLMs - BM25, Fine-tuned Embeddings, and Re-Rankers
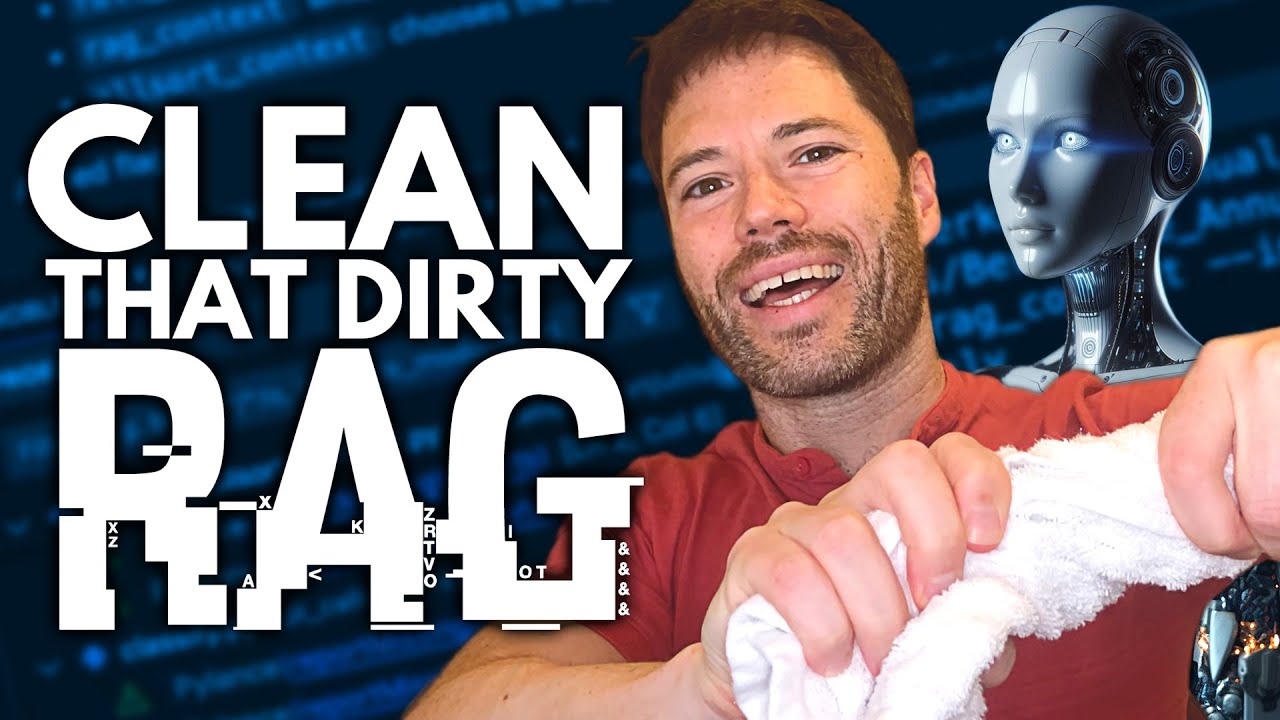
Показать описание
VIDEO RESOURCES:
TIMESTAMPS:
0:00 Mastering Retrieval (RAG) for LLMs
0:44 Video Overview
13:19 Baseline Performance with No Retrieval
17:29 Document Chunking - Naive vs Sentence based
24:34 BM25
33:20 Semantic / Vector / Embeddings Search
39:59 Cosine vs Dot Product Similarity
43:21 Generating Chunks and Embeddings
50:50 Running BM25 and Similarity Retrieval
55:22 Performance with BM25 vs Similarity
58:36 Fine-tuning embeddings / encoders
1:04:00 Preparing fine-tuning datasets
1:14:54 Embeddings Training Continued
1:22:00 Performance after Fine-tuning
1:25:58 Re-rankers
1:27:10: Cross-encoders
1:30:47 LLM re-rankers
1:36:11 Re-ranking performance
1:48:50 Final Tips
Mastering Retrieval for LLMs - BM25, Fine-tuned Embeddings, and Re-Rankers
Building Production-Ready RAG Applications: Jerry Liu
Mastering LLMs with RAG
Generative AI / LLM - Document Retrieval and Question Answering
What is Llama Index? how does it help in building LLM applications? #languagemodels #chatgpt
Different methods of using an LLMs! #llmwithav #learnwithav #llm #datascience #generativeai
Adding Agentic Layers to RAG
Mastering AI Jargon - Your Guide to OpenAI & LLM Terms
LLMs as Planners - Reasoning versus Retrieval
Roadmap to Learn Generative AI(LLM's) In 2024 With Free Videos And Materials- Krish Naik
Advanced Retrieval Augmented Generation (RAG): Build a Powerful llm application using Re-ranker
Mastering LLMs with Shanif Dhanani of Locusive
Unlocking LLM Potential: Mastering Medprompt for Enhanced AI in Healthcare
Build a Retrieval-Augmented Generation Chatbot in 5 Minutes
Prompt Engineering Tutorial – Master ChatGPT and LLM Responses
Mastering the Giants: Tackling the Challenges of LLMs in the Real World | PatternAI | Krupa Galiya
Fine-tuning Large Language Models (LLMs) | w/ Example Code
Mastering ChatGPT: Tips and Tricks for LLMs!
Mastering LLMs: Balancing Power and Unpredictability in AI Solutions
How to prepare for GenAI/LLM Interview? #artificialintelligence
Implement RAG (Retrieval Augmented Generation) #ai #springboot
Mastering RAG Evaluation: Metrics and Methods | Retrieval-Augmented Generation
Mastering LLM Accuracy of ServiceNow LLM Applications with Prompt Engineering
LangChain Master Class For Beginners 2024 [+20 Examples, LangChain V0.2]
Комментарии