filmov
tv
Python Integration, Interpolation, and Curve Fitting
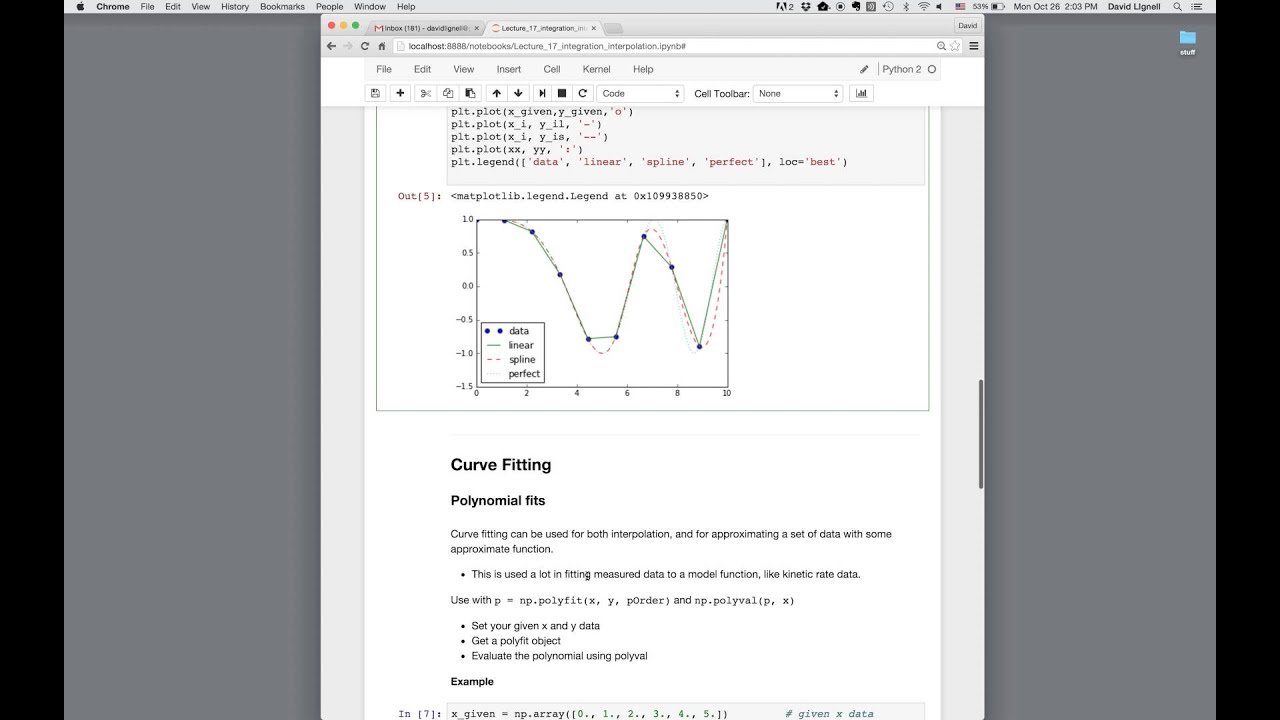
Показать описание
iPython Notebook, using numpy and scipy interpolation, integration, and curve fitting functions
Python Integration, Interpolation, and Curve Fitting
How To Interpolate Data In Python
Spline Interpolation In Python (Linear, Quadratic, Cubic, etc…) | Numerical Methods
Curve Fitting in Python (2022)
Nonlinear Equations, Integration, Derivatives, Interpolation in Python
PYTHON + MATPLOTLIB - Polynomial interpolation
5.1 Numerical Differentiation using Interpolation or Curve fitting with Example in Jupyter Notebook
Python Interpolation 2 of 4: 1d interpolation with Rbf
Python Tutorial #32 - Scipy Interpolation
SciPy Tutorial (2022): For Physicists, Engineers, and Mathematicians
Python Interpolation 1 of 4: 1d interpolation with interp1d
Interpolation in 5 minutes
Non-Linear CURVE FITTING using PYTHON
Exact Areas and Definite Integral with Python
Python 17- Numerical methods- Spline interpolation 1
How to: Import, Plot, Fit, and Integrate Data in Python
Introduction to Interpolation with SymPy
Python interpolation using scipy
Interpolation in Python
Linear interpolation using Python | GitHub Copilot
Python 🐍 Nonlinear Regression Curve Fit
Cubic Spline with Python GEKKO
Interpolation with Python 4 of 4: 2d interpolation with ndimage
3.5 - Interpolation using Newton's Method - Quadratic Equation (n=2) Case Example w/ Graph &...
Комментарии