filmov
tv
Efficiently Compute numpy Arrays with Conditions Using Numpy Functions
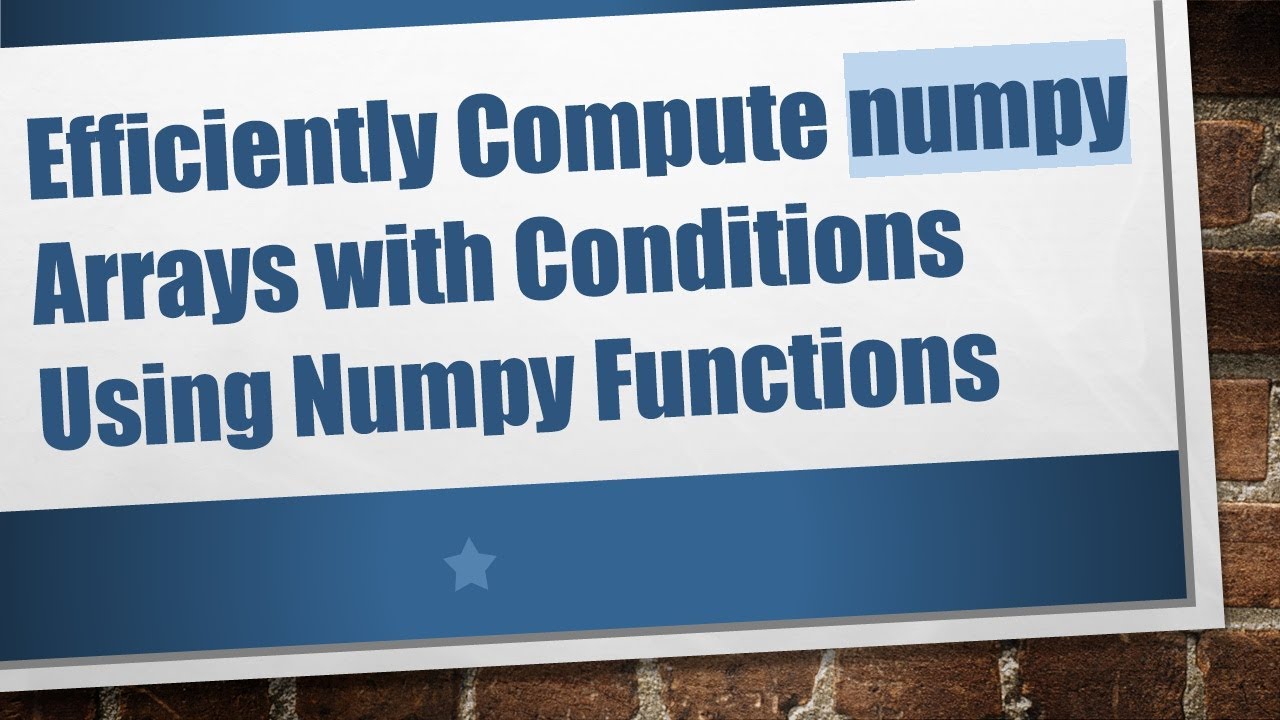
Показать описание
---
Visit these links for original content and any more details, such as alternate solutions, latest updates/developments on topic, comments, revision history etc. For example, the original title of the Question was: Compute numpy arrays based on another numpy array with each other
If anything seems off to you, please feel free to write me at vlogize [AT] gmail [DOT] com.
---
Efficiently Compute Numpy Arrays with Conditions
The Problem
Imagine you have three numpy arrays as follows:
[[See Video to Reveal this Text or Code Snippet]]
Your goal is to create a new array, d, which has the following logic:
If the corresponding value in array a is less than or equal to 3, d should contain the difference of b and c.
If the value in a is greater than 3, d should contain the sum of b and c.
In a scenario where performance is critical, you might start with a loop to handle this, but that can be significantly slower. Therefore, let's look into more efficient approaches using numpy.
Analyzing the Initial Approach
Loop Method
[[See Video to Reveal this Text or Code Snippet]]
Numpy Where Method
[[See Video to Reveal this Text or Code Snippet]]
Both approaches yield similar times for small arrays, but if we increase the size of the dataset, the performance differences become clear. As described:
The loop method took approximately 18.6 ms per loop.
Optimizing with Numpy Where
Benefits of Numpy Where
Vectorized Operations: It utilizes low-level optimizations allowing for broader, efficient operations on entire arrays without explicit loops.
Readability: Code is often cleaner and more straightforward.
Conclusion
Feel free to explore this method with larger datasets and see the performance benefits for yourself!
Visit these links for original content and any more details, such as alternate solutions, latest updates/developments on topic, comments, revision history etc. For example, the original title of the Question was: Compute numpy arrays based on another numpy array with each other
If anything seems off to you, please feel free to write me at vlogize [AT] gmail [DOT] com.
---
Efficiently Compute Numpy Arrays with Conditions
The Problem
Imagine you have three numpy arrays as follows:
[[See Video to Reveal this Text or Code Snippet]]
Your goal is to create a new array, d, which has the following logic:
If the corresponding value in array a is less than or equal to 3, d should contain the difference of b and c.
If the value in a is greater than 3, d should contain the sum of b and c.
In a scenario where performance is critical, you might start with a loop to handle this, but that can be significantly slower. Therefore, let's look into more efficient approaches using numpy.
Analyzing the Initial Approach
Loop Method
[[See Video to Reveal this Text or Code Snippet]]
Numpy Where Method
[[See Video to Reveal this Text or Code Snippet]]
Both approaches yield similar times for small arrays, but if we increase the size of the dataset, the performance differences become clear. As described:
The loop method took approximately 18.6 ms per loop.
Optimizing with Numpy Where
Benefits of Numpy Where
Vectorized Operations: It utilizes low-level optimizations allowing for broader, efficient operations on entire arrays without explicit loops.
Readability: Code is often cleaner and more straightforward.
Conclusion
Feel free to explore this method with larger datasets and see the performance benefits for yourself!