filmov
tv
Jo Kristian Bergum – AI-powered Semantic Search; A story of broken promises?
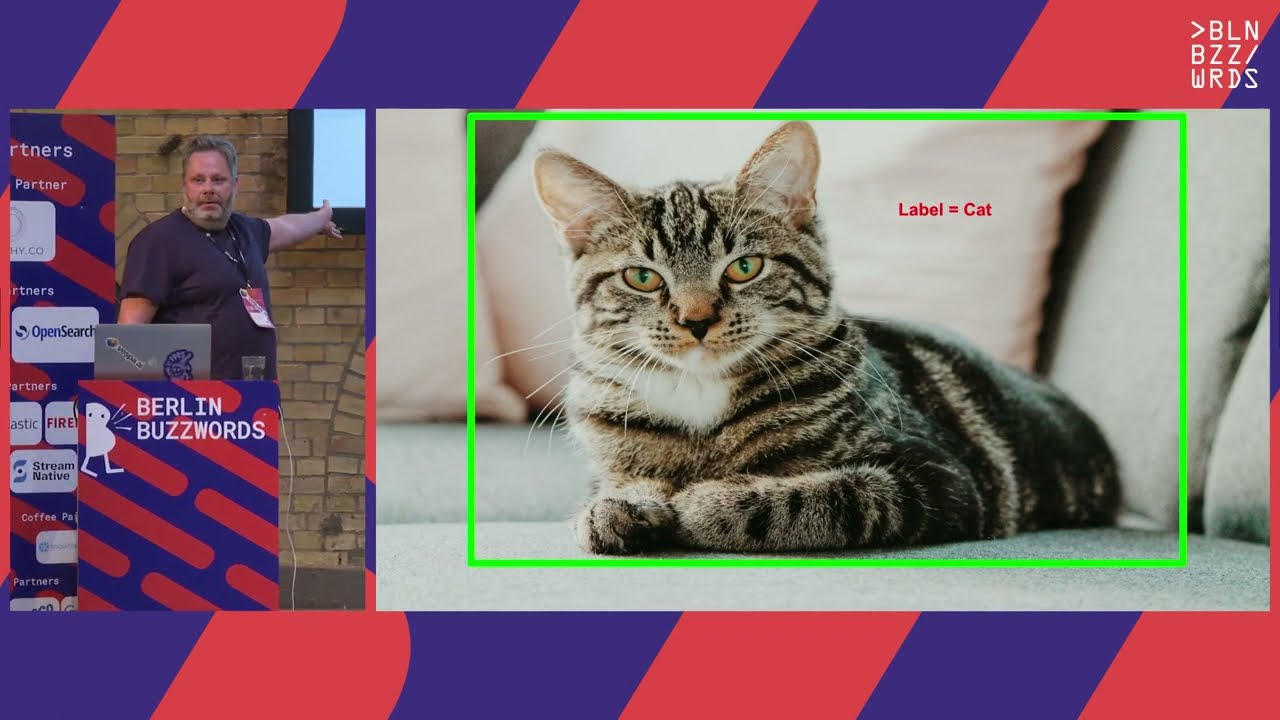
Показать описание
Semantic search using AI-powered vector embeddings of text, where relevancy is measured using a vector similarity function, has been a hot topic for the last few years. As a result, platforms and solutions for vector search have been springing up like mushrooms. Even traditional search engines like Elasticsearch and Apache Solr ride the semantic vector search wave and now support fast but approximative vector search, a building block for supporting AI-powered semantic search at scale.
Undoublty, sizeable pre-trained language models like BERT have revolutionized the state-of-the-art on data-rich text search relevancy datasets. However, the question search practitioners are asking themself is, do these models deliver on their promise of an improved search experience when applied to their domain? Furthermore, is semantic search the silver bullet which outcompetes traditional keyword-based search across many search use cases? This talk delves into these questions and demonstrates how these semantic models can dramatically fail to deliver their promise when used on unseen data in new domains.
Undoublty, sizeable pre-trained language models like BERT have revolutionized the state-of-the-art on data-rich text search relevancy datasets. However, the question search practitioners are asking themself is, do these models deliver on their promise of an improved search experience when applied to their domain? Furthermore, is semantic search the silver bullet which outcompetes traditional keyword-based search across many search use cases? This talk delves into these questions and demonstrates how these semantic models can dramatically fail to deliver their promise when used on unseen data in new domains.
Jo Kristian Bergum – AI-powered Semantic Search; A story of broken promises?
The state of Neural Search and LLMs, interview with Jo Kristian Bergum - Berlin Buzzwords 2023
Jo Bergum - Distinguished Engineer, Yahoo! Vespa - Journey of Vespa from Sparse into Neural Search
Jo Kristian Bergum - Boosting Ranking Performance with Minimal Supervision
Haystack EU 2023 - Jo Kristian Bergum: Navigating Neural Search: Avoiding Common Pitfalls
Haystack EU 2024 - Jo Kristian Bergum:What You See Is What You Search: Vision Language Models & ...
Jo Kristian Bergum – Search and Sushi; Freshness Counts
Back to Basics for RAG w/ Jo Bergum
Jo Kristian Bergum, Anshum Gupta, Josh Devins & Charlie Hull – The Debate: Which Search Engine?...
Berlin Buzzwords 2023: The Debate Returns (with more vectors): Which Search Engine?
Taking the neural search paradigm shift to production
preparing for semantic Search in AI | Artificial Intelligence
AI and Discovery: Semantic Search, Vector Search, Neural Search and Beyond - Niraj Aswani CTO Klevu
Haystack LIVE! The Great Search Engine Debate - Elasticsearch, Solr or Vespa?
Vespa - Open Source Big Data Serving Engine, Jon Bratseth, Distinguished Architect, Verizon Media
Jennifer Ding - What defines the 'open' in 'open AI'?
Vespa ai: Debugging a Vespa Searcher
What Goes Around Comes Around... And Around... - Andy Pavlo (Dijkstra Award 2024)
Custom RAG Evaluations w/ Vespa.ai
Dainius Jocas – Tips and Tricks to Scale Elasticsearch for 1M RPMs and Beyond
Getting Started with Vespa (open source big data serving engine) using Docker
Dmitry Kan on Neural Search Frameworks - Weaviate Podcast #34
Fokko Driesprong - Tip of the Iceberg
Umesh Dangat – NrtSearch: Yelp’s fast, scalable, and cost-effective open source search engine
Комментарии