filmov
tv
224 - Recurrent and Residual U-net
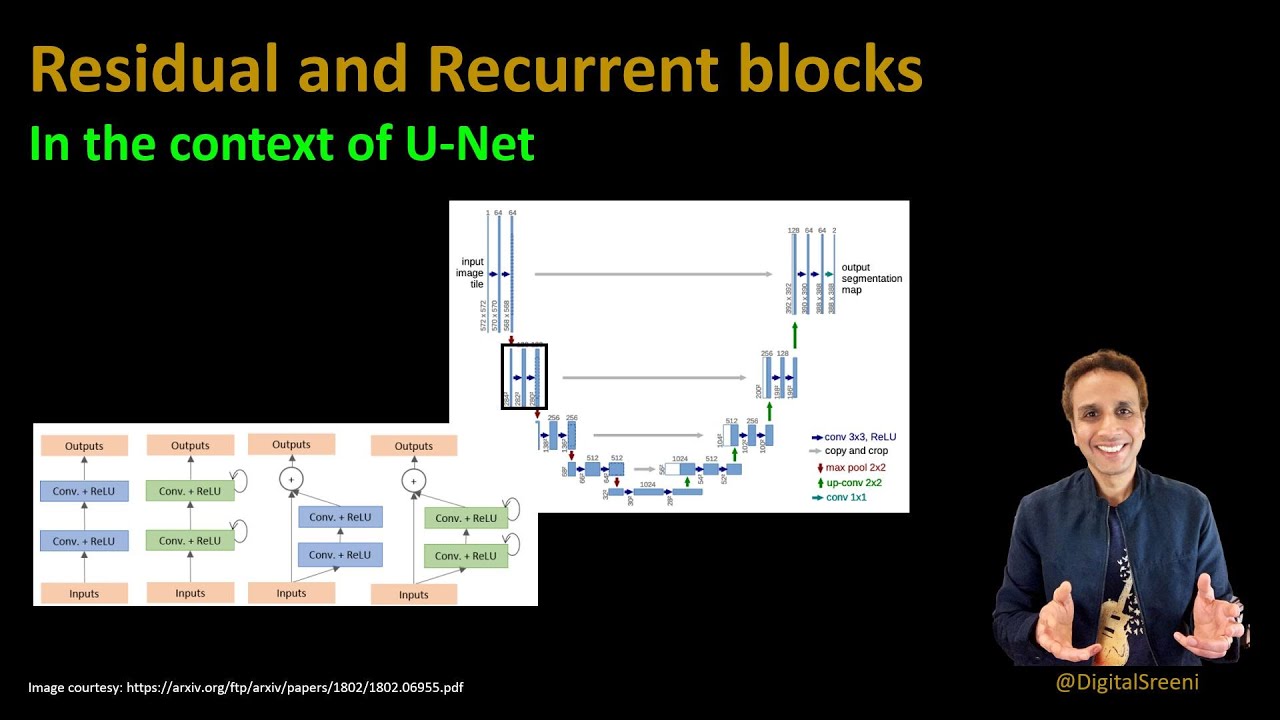
Показать описание
Residual Networks:
Residual networks were proposed to overcome the problems of deep CNNs (e.g., VGG). Stacking convolutional layers and making the model deeper hurts the generalization ability of the network. To address this problem, ResNet architecture was introduced which adds the idea of “skip connections”.
In traditional neural networks, each layer feeds into the next layer. In networks with residual blocks, each layer feeds into the next layer and directly into the layers about 2–3 hops away. Inputs can forward propagate faster through the residual connections (shortcuts) across layers.
Recurrent convolutional networks:
The recurrent network can use the feedback connection to store information over time. Recurrent networks use context information; as time steps increase, the network leverages more and more neighborhood information. Recurrent and CNNs can be combined for image-based applications. With recurrent convolution layers, the network can evolve over time though the input is static. Each unit is influenced by its neighboring units, includes the context information of an image.
U-net can be built using recurrent or residual or a combination block instead of the traditional double-convolutional block.
Residual networks were proposed to overcome the problems of deep CNNs (e.g., VGG). Stacking convolutional layers and making the model deeper hurts the generalization ability of the network. To address this problem, ResNet architecture was introduced which adds the idea of “skip connections”.
In traditional neural networks, each layer feeds into the next layer. In networks with residual blocks, each layer feeds into the next layer and directly into the layers about 2–3 hops away. Inputs can forward propagate faster through the residual connections (shortcuts) across layers.
Recurrent convolutional networks:
The recurrent network can use the feedback connection to store information over time. Recurrent networks use context information; as time steps increase, the network leverages more and more neighborhood information. Recurrent and CNNs can be combined for image-based applications. With recurrent convolution layers, the network can evolve over time though the input is static. Each unit is influenced by its neighboring units, includes the context information of an image.
U-net can be built using recurrent or residual or a combination block instead of the traditional double-convolutional block.
Комментарии