filmov
tv
Etienne Dilocker on Vector Search Engines and Weaviate
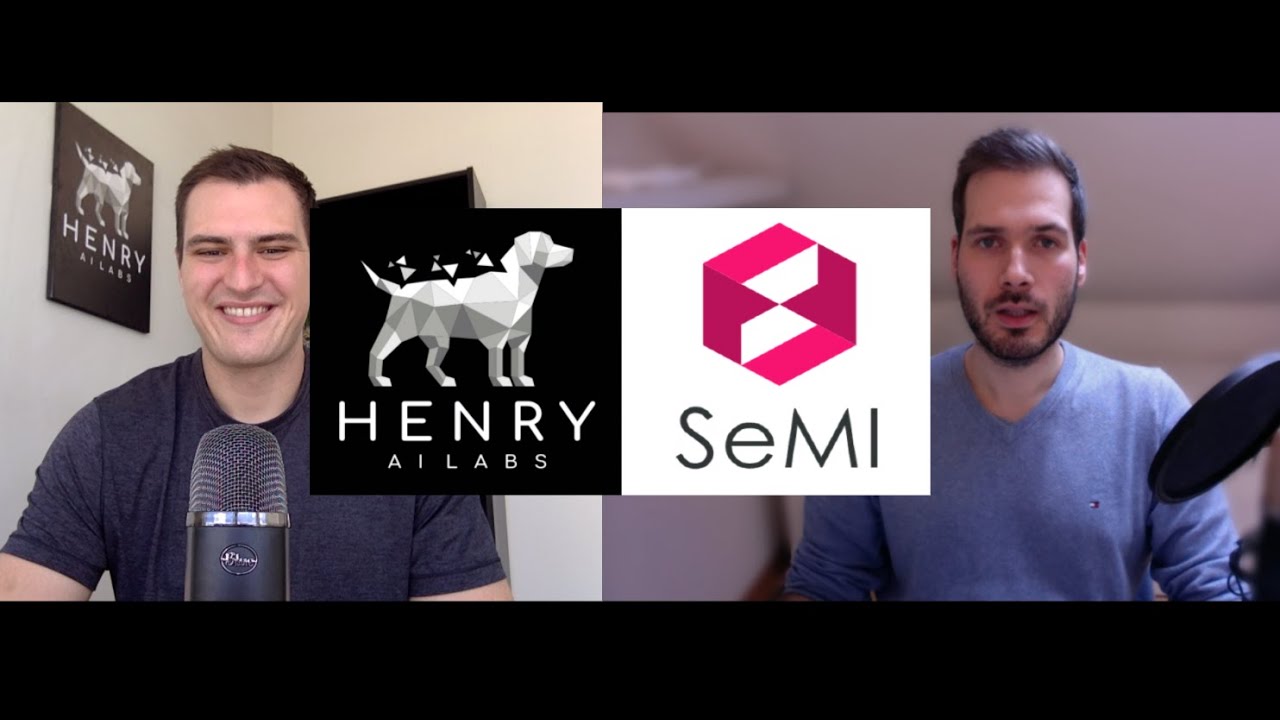
Показать описание
Vector Search Engines are powering the the next generation of search. Instead of relying on things like BM25 or TF-IDF representations, we use neural representations to compare semantic similarity. Dot products to calculate the similarity between all vectors would be very time consuming. Hierarchical Navigable Small World Graphs (HNSW) are a cutting-edge new algorithm used by Weaviate to speed up this search. This podcast explores HNSW, Neurosymbolic search and filtering, and many more!
I hope you find this interesting, happy to answer any questions left on the YouTube video!
Check out this Introduction to Weaviate: Very well organized, no headaches getting started!
Chapters
0:00 Introduction
1:30 Why Vector Search Engines?
3:02 Hierarchical Navigable Small World Graphs and Scaling
9:00 Setup Questions
12:57 What is the compute heavy part?
17:15 Neurosymbolic Databases
23:53 Weaviate for Language Models
26:17 Weaviate for HuggingFace or PapersWithCode Datasets
30:43 Weaviate Modules
33:58 Text-to-Image Vector Search
36:54 What inspired Etienne to work on this?
I hope you find this interesting, happy to answer any questions left on the YouTube video!
Check out this Introduction to Weaviate: Very well organized, no headaches getting started!
Chapters
0:00 Introduction
1:30 Why Vector Search Engines?
3:02 Hierarchical Navigable Small World Graphs and Scaling
9:00 Setup Questions
12:57 What is the compute heavy part?
17:15 Neurosymbolic Databases
23:53 Weaviate for Language Models
26:17 Weaviate for HuggingFace or PapersWithCode Datasets
30:43 Weaviate Modules
33:58 Text-to-Image Vector Search
36:54 What inspired Etienne to work on this?
Etienne Dilocker on Vector Search Engines and Weaviate
Erik Bernhardsson and Etienne Dilocker on Vector Search in Production - Weaviate Podcast #25
Weaviate Podcast #10 - Yury Malkov and Etienne Dilocker about HNSW in Vector Search and Weaviate
Solving Multi-Tenancy In Vector Search Requires A Paradigm Shift, Etienne Dilocker, CTO, Weaviate
Weaviate: An Architectural Deep Dive (Etienne Dilocker)
Etienne Dilocker on ANN Benchmarks - Weaviate podcast #16
Etienne Dilocker on Weaviate 1.20 - Weaviate Podcast #56!
What is a Vector Database?
Etienne Dilocker on Weaviate v1.14 Release! - Weaviate Podcast #19
Weaviate v1.15 Release with Etienne Dilocker and Dirk Kulawiak - Weaviate Podcast #24
Vector Search and Embeddings in Organizations: Harnessing Language Models and Vector Indexing
Weaviate 1.17 Release with Etienne Dilocker and Parker Duckworth - Weaviate Podcast #31
Weaviate Podcast #1 • Etienne Dilocker of SeMI • Community and Weaviate core update
Farshad Farahbakhshian and Etienne Dilocker on Weaviate and AWS - Weaviate Podcast #67!
Presenting... The Weaviate Podcast!
Weaviate 1.23 Release Podcast with Etienne Dilocker!
Our Mad Journey of Building a Vector Database in Go - Weaviate at FOSDEM 2023
Weaviate 1.19 Release with Etienne Dilocker - Weaviate Podcast #44!
Guest Lecture: Vector Quantization Techniques with Etienne | Brown University CSCI
Approximate Nearest Neighbor Benchmarks - Weaviate Podcast Recap
Weaviate Meetup June – Architecture Deep Dive, how to build a vector database
Q&A module for the Weaviate Vector Database - Introduction
Live from Berlin Buzzwords 2022 - with developers from Weaviate, Jina AI and Apache Solr
Binary Passage Retrieval in Weaviate (32x Memory Savings)
Комментарии