filmov
tv
Empirically Measuring, & Reducing, C++’s Accidental Complexity - Herb Sutter - CppCon 2020
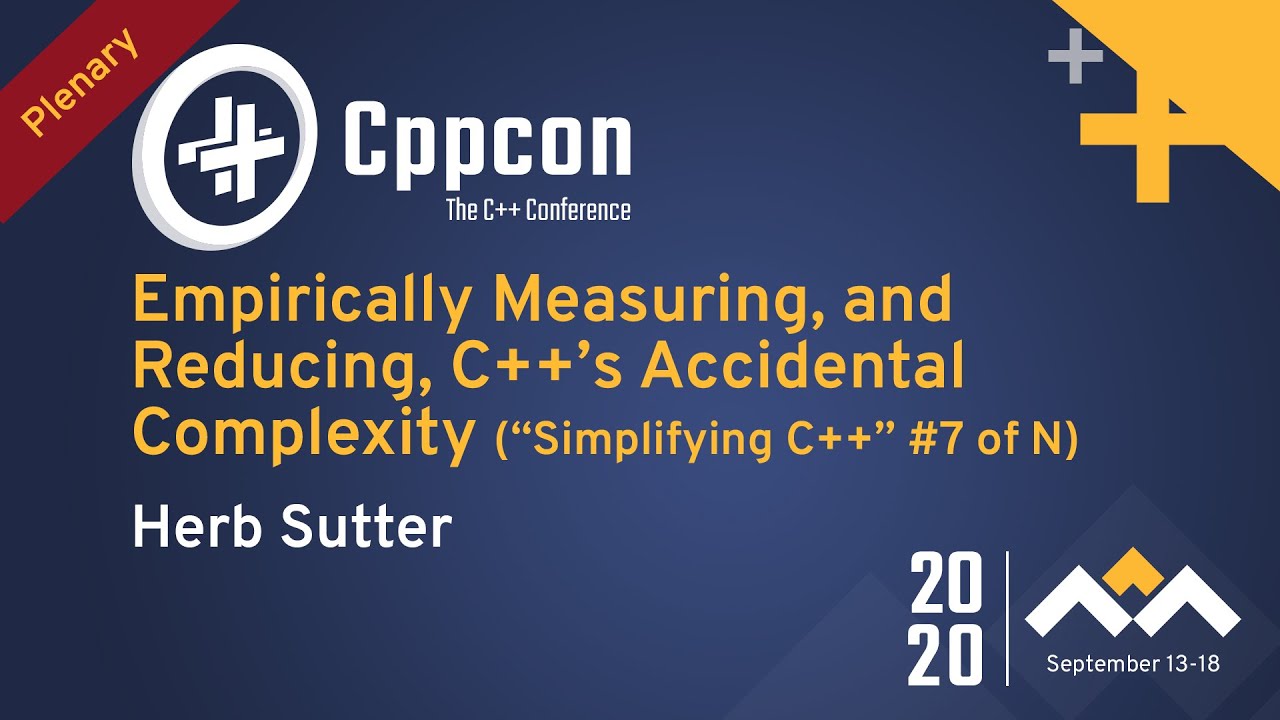
Показать описание
---
We often hear “C++ is more complex than it needs to be,” typically demonstrated using anecdotes and “gotcha” examples. Those can be valid and demonstrate real pain points, but it would be nice to have more quantifiable data that we could analyze to measure sources of complexity. This talk reports work to systematically catalog and measure C++’s unneeded complexity, how some current evolution proposals may address its major sources, and presents specific suggestions on what we might be able to do about it in the context of a future-evolution proposal to simplify parameter passing and provide meaningful initialization guarantees in C++.
---
Herb is the chair of the ISO C++ standards committee, a programming language architect at Microsoft, and the author of over 200 articles and 4 books about C++ and related topics.
---
*-----*
*-----*
We often hear “C++ is more complex than it needs to be,” typically demonstrated using anecdotes and “gotcha” examples. Those can be valid and demonstrate real pain points, but it would be nice to have more quantifiable data that we could analyze to measure sources of complexity. This talk reports work to systematically catalog and measure C++’s unneeded complexity, how some current evolution proposals may address its major sources, and presents specific suggestions on what we might be able to do about it in the context of a future-evolution proposal to simplify parameter passing and provide meaningful initialization guarantees in C++.
---
Herb is the chair of the ISO C++ standards committee, a programming language architect at Microsoft, and the author of over 200 articles and 4 books about C++ and related topics.
---
*-----*
*-----*
Empirically Measuring, & Reducing, C++’s Accidental Complexity - Herb Sutter - CppCon 2020
Empirical Risk Minimization
CS-C3240. Empirical Risk Minimization.
Empirical Risk Minimization
Percentiles (1.7)
Metacognitive calibration: a methodological expansion and empirical application - ISLS 2023
a defense of the imperial measurement system
An empirical approach to measuring and calibrating for error in observational analyses
Quantifying Bliss: Empirically Testable Hypotheses For Valence
Some bounds for the Wasserstein distance between the empirical measure
GCSE Chemistry - Moles, Concentration & Volume Calculations #29
Why No One Has Measured The Speed Of Light
Confidence Interval [Simply explained]
Statistics - How to use the Empirical Rule
There’s No Wave Function? | Jacob Barandes
The Code Quality Advantage: How Empirical Data Shatters the Speed vs Quality Myth by Adam Tornhill
1.10 Measures of Position and the Empirical Rule
Support Vector Machine (SVM) in 2 minutes
Fractals are typically not self-similar
Empirical Modeling of Variance in Medium Frequency R-Mode Time-of-Arrival Measurements -
Empirical Formula & Molecular Formula Determination From Percent Composition
10 - Model Order Reduction - (Discrete) Empirical Interpolation Method (DEIM)
Empirical Evap. Eq., Analytical Methods of Evap. Est., Reservoir Evap. and Methods for its Reduction
2.5a Empirical Rule and Chebyshev's Rule revised
Комментарии