filmov
tv
Demystifying SELF-RAG: A Comprehensive Guide with Examples (Exclusive on LlamaIndex!)
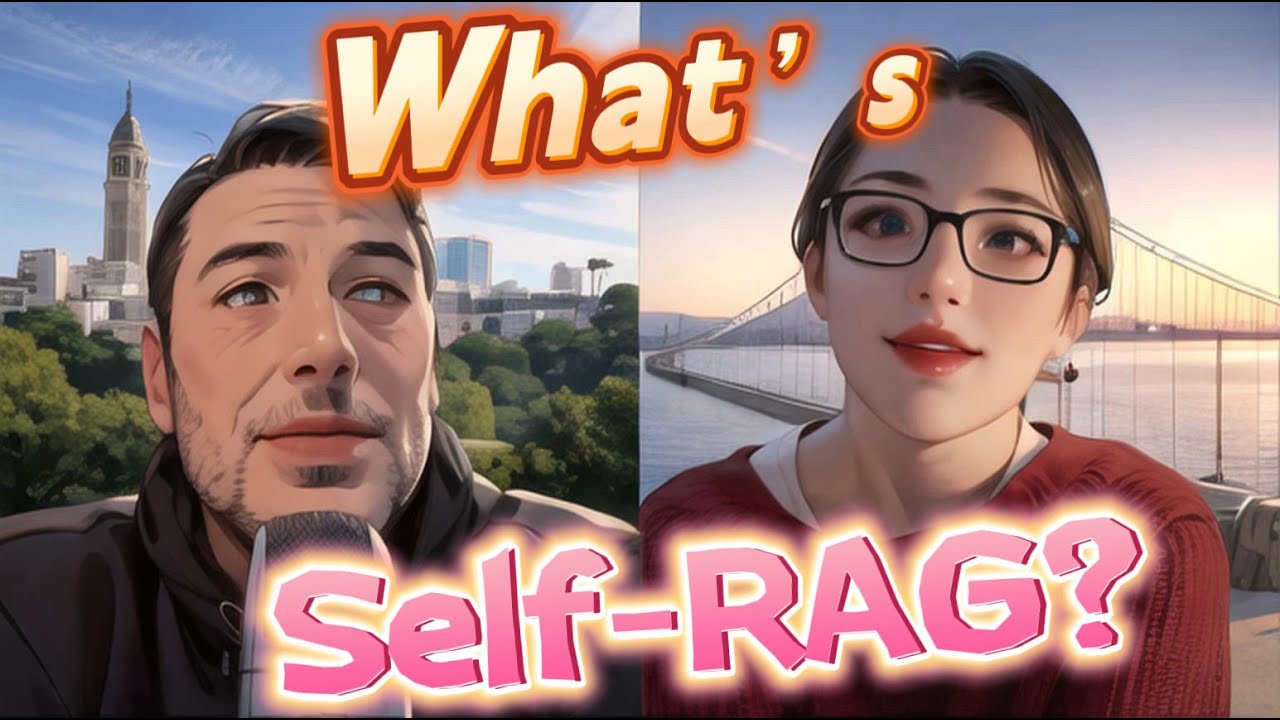
Показать описание
Happy the Year of Dragon, everyone! 🐲
In this episode, join Mehdi and Angelina as they break down SELF-RAG, the powerful new algorithm now available as part of LlamaPack on the cutting-edge LlamaIndex platform. This game-changer takes Retrieval-Augmented Generation (RAG) to the next level, unlocking dynamic capabilities like real-time adaptation and critique.
00:00 Introduction
00:40 What is RAG (Retrieval Augmented Generation)?
02:54 What’s good about RAG comparing with ChatGPT?
03:08 What issues does RAG have?
05:04 What’s the solution?
05:52 What is Query Routing?
06:33 How does Self-RAG work?
07:12 What’s reflective token?
07:49 How does Self-RAG know whether to retrieve or not?
09:11 A concrete example
13:27 How does the algorithm work?
14:31 An example output from the model
17:13 Self-evaluation and critique from retrieval
18:49 Model performance overview
19:35 Benefit for RAG developers - why use this?
🔨 Implementation:
📝 Paper:
Stay tuned for more content! 🎥 Thanks you for watching! 🙌
In this episode, join Mehdi and Angelina as they break down SELF-RAG, the powerful new algorithm now available as part of LlamaPack on the cutting-edge LlamaIndex platform. This game-changer takes Retrieval-Augmented Generation (RAG) to the next level, unlocking dynamic capabilities like real-time adaptation and critique.
00:00 Introduction
00:40 What is RAG (Retrieval Augmented Generation)?
02:54 What’s good about RAG comparing with ChatGPT?
03:08 What issues does RAG have?
05:04 What’s the solution?
05:52 What is Query Routing?
06:33 How does Self-RAG work?
07:12 What’s reflective token?
07:49 How does Self-RAG know whether to retrieve or not?
09:11 A concrete example
13:27 How does the algorithm work?
14:31 An example output from the model
17:13 Self-evaluation and critique from retrieval
18:49 Model performance overview
19:35 Benefit for RAG developers - why use this?
🔨 Implementation:
📝 Paper:
Stay tuned for more content! 🎥 Thanks you for watching! 🙌
Demystifying SELF-RAG: A Comprehensive Guide with Examples (Exclusive on LlamaIndex!)
AI Critique with SELF-REFLECTION 🤯 (powered RAG)!!!
Self-Reflective AI: Self-RAG for Multi-AI-Agents explained
Self-RAG : Self Reflective AI for powerful Retrieval Augmented Generation
Demystifying LLM & AI - A Beginner to Intermediate Guide
Why Everyone is Freaking Out About RAG
RAG (Retrieval-Augmented Generation) | What is Retrieval-Augmented(RAG)? | LLM | Simplilearn
Self-RAG: Learning to Retrieve, Generate and Critique through Self-Reflections
Demystifying LLM & Frameworks: A Comprehensive Guide
Transformer Neural Networks, ChatGPT's foundation, Clearly Explained!!!
Attention in transformers, visually explained | Chapter 6, Deep Learning
InstructRetro: INSTRUCTION TUNING POSTRETRIEVAL-AUGMENTED PRETRAINING
LangChain Explained In 15 Minutes - A MUST Learn For Python Programmers
How To Wash & Dry Your Microfiber Towels Like A Pro!
Attention is all you need (Transformer) - Model explanation (including math), Inference and Training
Demystifying 'Generative AI for Everyone' with Altaf Rehmani
How to Fine-Tune and Train LLMs With Your Own Data EASILY and FAST- GPT-LLM-Trainer
Graph-of-Thoughts (GoT) for AI reasoning Agents
Demystifying Archival Supplies
6 Signs That Your Car Needs A Detail | THE RAG COMPANY
How I'd Learn AI (If I Had to Start Over)
Mastering ChatGPT Prompting with Three Simple Techniques
Don't Forget Your Easel! Quick, Easy Half French Easel Assembly Guide 🎨🌞 #pleinair #outdoorpain...
Demystifying LLMs and Threats My Journey
Комментарии