filmov
tv
Local RAG LLM with Ollama
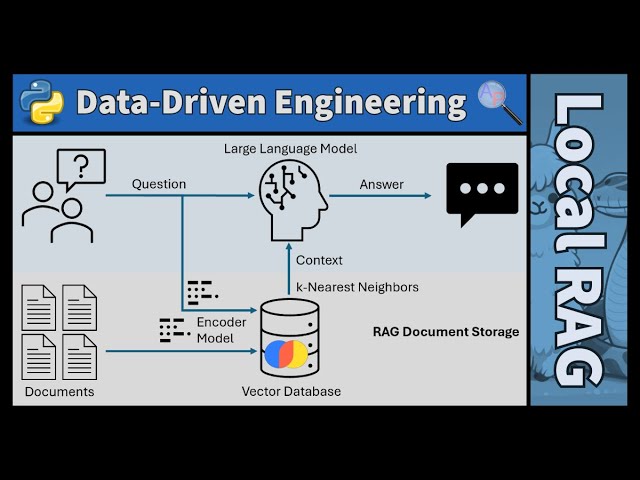
Показать описание
Applications of Retrieval-Augmented Generation (RAG) and Large Language Models (LLMs) give context-aware solutions for complex Natural Language Processing (NLP) tasks. Natural language processing (NLP) is a machine learning technology that gives computers the ability to interpret, manipulate, and interact with human language. Combining RAG and LLMs enables personalized, multilingual, and context-aware systems. The objective of this tutorial is to implement RAG for user-specific data handling, develop multilingual RAG systems, use LLMs for content generation, and integrate LLMs in code development.
Retrieval-Augmented Generation (RAG)
LLM with Ollama Python Library
LLM with Ollama Python Library is a tutorial on Large Language Models (LLMs) with Python with the ollama library for chatbot and text generation. It covers the installation of the ollama server and ollama python package and uses different LLM models like mistral, gemma, phi, and mixtral that vary in parameter size and computational requirements.
RAG and LLM Integration
Combining Retrieval-Augmented Generation (RAG) with Large Language Models (LLMs) leads to context-aware systems. RAG optimizes the output of a large language model by referencing an external authoritative knowledge base outside of initial training data sources. These external references generate a response to provide more accurate, contextually relevant, and up-to-date information. In this architecture, the LLM is the reasoning engine while the RAG context provides relevant data. This is different than fine-tuning where the LLM parameters are augmented based on a specific knowledge database.
The synergy of RAG enhances the LLM ability to generate responses that are not only coherent and contextually appropriate but also enriched with the latest information and data, making it valuable for applications that require higher levels of accuracy and specificity, such as customer support, research assistance, and specialized chatbots. This combines the depth and dynamic nature of external data with the intuitive understanding and response generation of LLMs for more intelligent and responsive AI systems.
RAG with LLM (Local)
Retrieval-Augmented Generation (RAG)
LLM with Ollama Python Library
LLM with Ollama Python Library is a tutorial on Large Language Models (LLMs) with Python with the ollama library for chatbot and text generation. It covers the installation of the ollama server and ollama python package and uses different LLM models like mistral, gemma, phi, and mixtral that vary in parameter size and computational requirements.
RAG and LLM Integration
Combining Retrieval-Augmented Generation (RAG) with Large Language Models (LLMs) leads to context-aware systems. RAG optimizes the output of a large language model by referencing an external authoritative knowledge base outside of initial training data sources. These external references generate a response to provide more accurate, contextually relevant, and up-to-date information. In this architecture, the LLM is the reasoning engine while the RAG context provides relevant data. This is different than fine-tuning where the LLM parameters are augmented based on a specific knowledge database.
The synergy of RAG enhances the LLM ability to generate responses that are not only coherent and contextually appropriate but also enriched with the latest information and data, making it valuable for applications that require higher levels of accuracy and specificity, such as customer support, research assistance, and specialized chatbots. This combines the depth and dynamic nature of external data with the intuitive understanding and response generation of LLMs for more intelligent and responsive AI systems.
RAG with LLM (Local)
Комментарии