filmov
tv
Shortest Path Finder - GA - Genetic Algorithms - Travelling Salesman's Problem
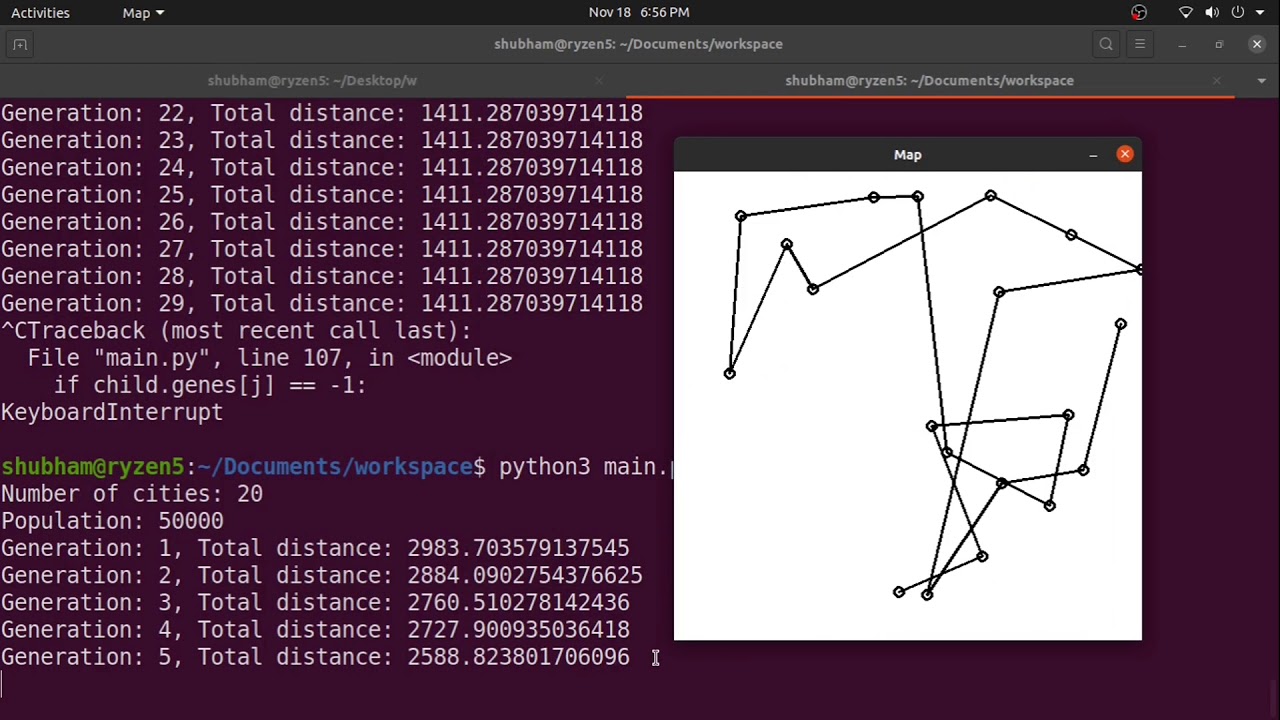
Показать описание
Subscribe for more.
Series of tutorials Is coming.
A new blog website will be launched soon.
Shortest Path Finder - GA - Genetic Algorithms - Travelling Salesman's Problem
Dijkstra's algorithm in 3 minutes
Shortest Pathfinder using Genetic Algorithm
Find the Shortest Path - Intro to Algorithms
Dijkstra's Shortest Path Algorithm - Intro to Algorithms
Find the Shortest Path - Intro to Algorithms
Genetic Algorithm to Find Shortest Path
Shortest Path Substructure - GT - Computability, Complexity, Theory: Algorithms
All Pairs Shortest Path - Georgia Tech - Computability, Complexity, Theory: Algorithms
Finding the shortest path using Dijkstra's Algorithm
Two Techniques for Fast Computation of Constrained Shortest Paths
How to Find the Shortest Path - Intro to Algorithms
Hierarchical Multiobjective Shortest Path Problems
How to find shortest path in network using numerical method - shortest path problem (example 3)
Net 2 Shortest Path
Shortest Path using The Genetics Algorithm
KaVe 201 Lecture4-GA part 5/5 Finding Shortest Path with Genetic Algorithm
Longest Simple Path - Intro to Algorithms
Finding the k-shortest path between two nodes
MAPV101 Algorithms Shortest Path Moores Breadth First Search Matrix Method
Social Network Analysis: Shortest Path Comparison of Dijkstra and Bellman ford Algorithm
Find the Shortest Path for Multiple OD Pairs: Closest Facility & OD Cost Matrix
Using genetic algorithm to find the shortest path of TSP
Application of Genetic Algorithm in modeling of Shortest Path problem
Комментарии