filmov
tv
The Images in-between | Before Diffusion: Variational Autoencoder VAE explained w/ KL Divergence
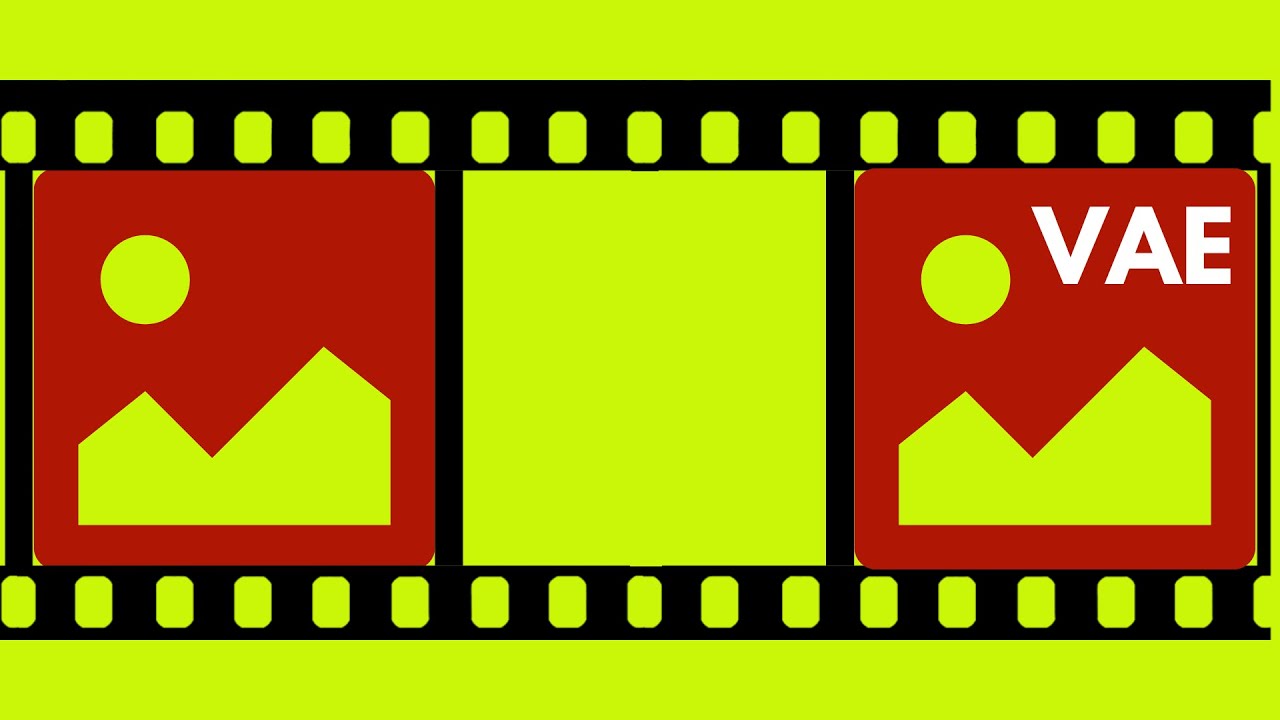
Показать описание
On the road to Diffusion theory for text-to-image and text-to-video. Generative AI.
The answers to all your questions:
1. enforcing a probabilistic prior on the latent space
2. restrict our posterior approximation to Gaussian distribution.
3. KL-Div as a regularization of the posterior
4. keep thee latent distribution compact around a subspace.
5. smooth transitions of data points in latent space.
6. how can a generative system create such unseen images?
Further reading
Outlook to Vector-Quantized Variational Autoencoder for text-to-image AI tools.
#ai
#datascience
#stablediffusion
The Images in-between | Before Diffusion: Variational Autoencoder VAE explained w/ KL Divergence
Before, After and In between | Learn Pre-School Concepts For Kids with Siya
Before, After and In Between | Mathematics Book A | Periwinkle
Wayanad Landslide: Before & After Satellite Images Revealed | ISRO Pictures Out
Before After Between
Random PREGNANT Battle Between Alpha Hero #shorts #spiderman #alphahero
Random REVERSE SPIDER-GIRL Battle Between Alpha Hero #shorts #spiderman #alphahero
In Between Before and After Photos
Leaked?: Imane Khelif's Childhood Images Emerge As Gender Debate Intensifies At Paris Olympics ...
Random REVERSE SPIDER-MAN Battle Between Alpha Hero #shorts #spiderman #alphahero
How to teach before , after and between numbers for kids.
Can you spot the difference between this image and the one before this short.#art #minecraft #song.
#Before and after #2020🙄 | #90s vs 2k trends | difference between 90s and 2k
Random REVERSE SPIDER-GIRL Battle Between Alpha Hero #shorts #spiderman #alphahero
check the difference between in the pic after and before
What is the difference between before and after ? 🤔🤔 #shorts
Random PREGNANT Battle Between Alpha Hero #3 #shorts #spiderman #alphahero
'Rare Historical Photos That Will Blow Your Mind! 📸✨ #HistoryUnveiled #VintagePics' #histo...
Random Equipment - THOR battle between alpha heroes🔥 #alphahero #spiderman #shorts
Berlin, Germany Before and Between the Wars
After Before & Between Numbers || Maths Concept For Kids
The contrast between the before and after photos of brother and sister is huge. Netizens are so rea
Before, After and Between Number
Big fight between V and Jin backstage before their concert 😭😭💔
Комментарии