filmov
tv
Dmitry Krotov | Modern Hopfield Networks for Novel Transformer Architectures
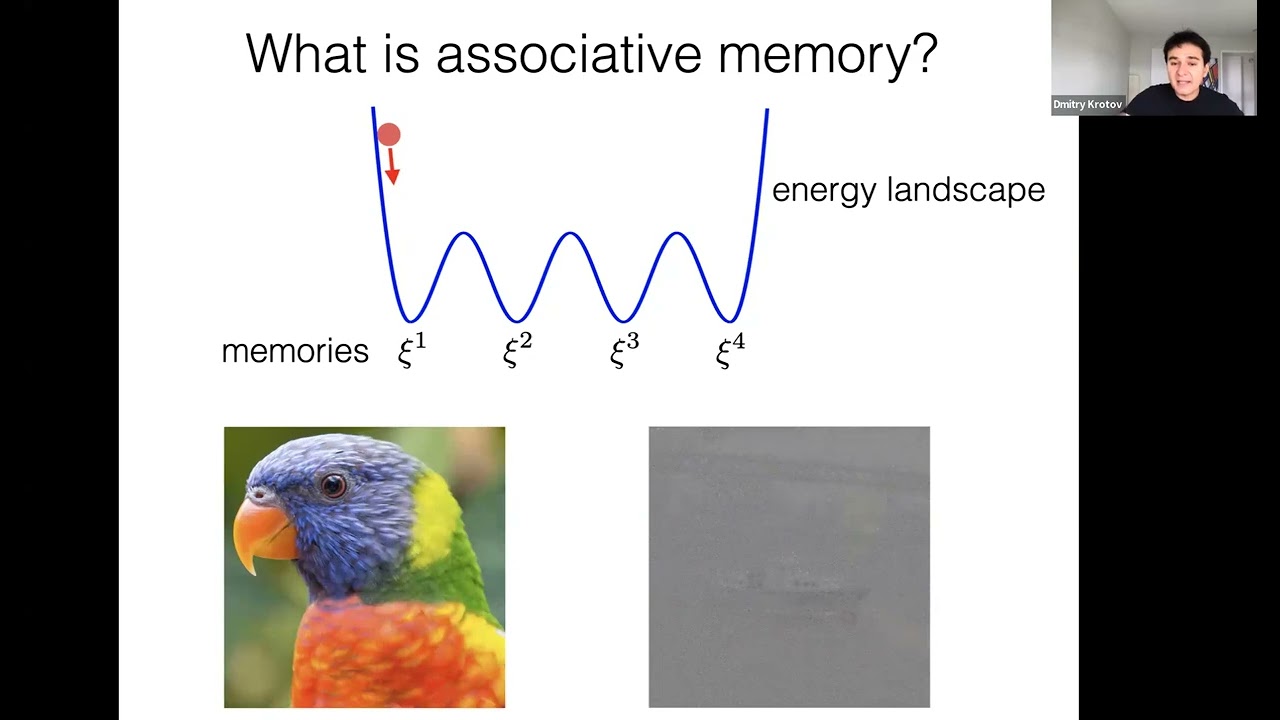
Показать описание
New Technologies in Mathematics Seminar
Speaker: Dmitry Krotov, IBM Research – Cambridge
Title: Modern Hopfield Networks for Novel Transformer Architectures
Abstract: Modern Hopfield Networks or Dense Associative Memories are recurrent neural networks with fixed point attractor states that are described by an energy function. In contrast to conventional Hopfield Networks, which were popular in the 1980s, their modern versions have a very large memory storage capacity, which makes them appealing tools for many problems in machine learning and cognitive and neurosciences. In this talk, I will introduce an intuition and a mathematical formulation of this class of models and will give examples of problems in AI that can be tackled using these new ideas. Particularly, I will introduce an architecture called Energy Transformer, which replaces the conventional attention mechanism with a recurrent Dense Associative Memory model. I will explain the theoretical principles behind this architectural choice and show promising empirical results on challenging computer vision and graph network tasks.
Speaker: Dmitry Krotov, IBM Research – Cambridge
Title: Modern Hopfield Networks for Novel Transformer Architectures
Abstract: Modern Hopfield Networks or Dense Associative Memories are recurrent neural networks with fixed point attractor states that are described by an energy function. In contrast to conventional Hopfield Networks, which were popular in the 1980s, their modern versions have a very large memory storage capacity, which makes them appealing tools for many problems in machine learning and cognitive and neurosciences. In this talk, I will introduce an intuition and a mathematical formulation of this class of models and will give examples of problems in AI that can be tackled using these new ideas. Particularly, I will introduce an architecture called Energy Transformer, which replaces the conventional attention mechanism with a recurrent Dense Associative Memory model. I will explain the theoretical principles behind this architectural choice and show promising empirical results on challenging computer vision and graph network tasks.
Dmitry Krotov | Modern Hopfield Networks for Novel Transformer Architectures
Hopfield Networks in 2021 - Fireside chat between Sepp Hochreiter and Dmitry Krotov | NeurIPS 2020
Dense Associative Memory in Machine Learning
Dmitry Krotov, IBM | Machine Learning Workshop
Large Associative Memory Problem in Neurobiology and Machine Learning - Dmitry Krotov, PhD
T22.3: Biological Softmax Demonstrated in Modern Hopfield Networks
Modern Hopfield Networks for Sample-Efficient Return Decomposition from Demonstrations
How are memories stored in neural networks? | The Hopfield Network #SoME2
ICML 2021 | Modern Hopfield Networks - Dr Sepp Hochreiter
Dense Associative Memories and Deep Learning
Modern Hopfield Networks // Computer Vision Meetup // March 2021
NIPS 2016 Spotlight video Dense Associative Memory for Pattern Recognition
What is Hopfield Networks in Machine Learning?
Modern Hopfield Networks - Dr Sepp Hochreiter
NDC1.4 - Hopfield Model
Introducing Hopfield Networks 2.0
NIPS: Oral Session 7 - John J. Hopfield
Lecture 25: Hopfield Nets and Auto Associators
Week 08c: Hopfield Demonstration
Simplicial Hopfield networks - Neuro Seminar 2
20: Hopfield Networks - Intro to Neural Computation
Understanding Transformers as Hopfield Networks #machinelearning #transformers
Day 14 - 2023 Telluride Neuromorphic Workshop
Modern Hopfield Networks in DL Architectures II - Dr Sepp Hochreiter
Комментарии