filmov
tv
LLMs in Production: Fine-Tuning, Scaling, and Evaluation at Atlassian
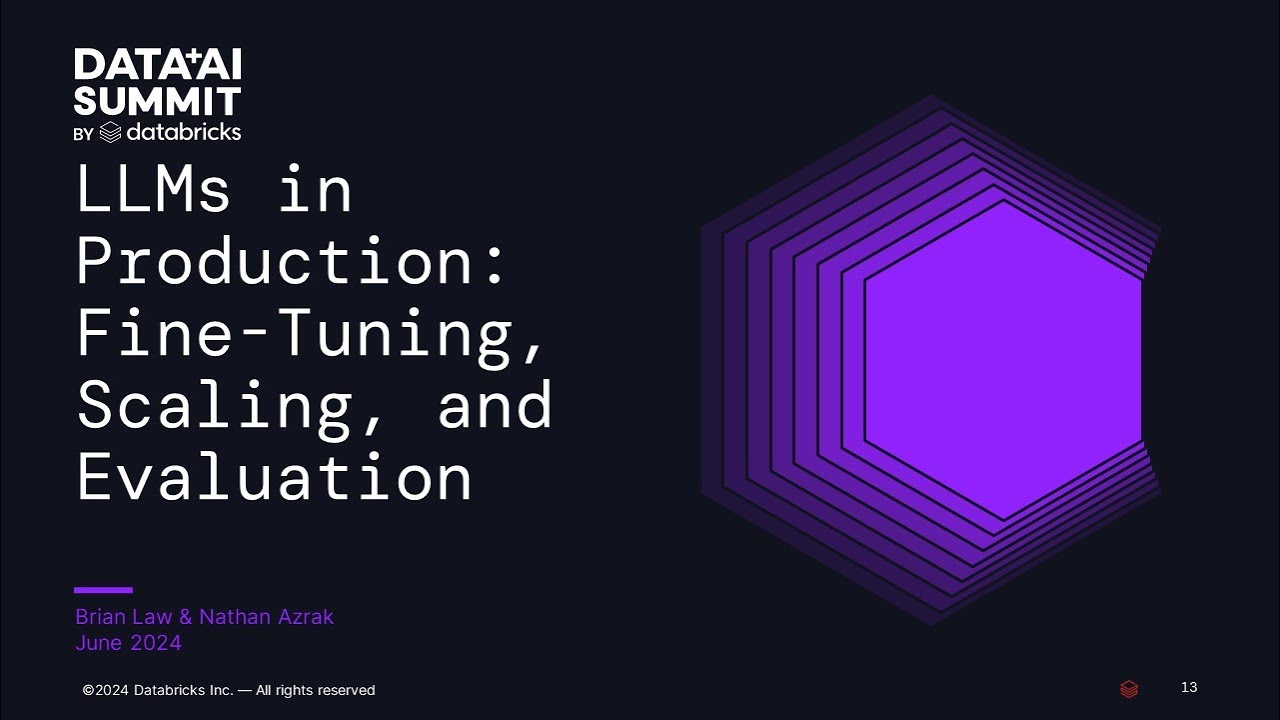
Показать описание
We will dive into the practicalities of deploying LLMs in business settings. We'll explore when to leverage LLMs and address how to minimize the complexity of the problem. Our discussion will guide you through designing an evaluation methodology and detail the circumstances necessitating fine-tuning for optimal performance. We will elaborate on the nuances of training data selection, establishing a flexible training ecosystem, hyperparameter optimization, scalable training, and finetuning workflows. As part of the practical session, we will go through the ETL process, how to format and structure data for finetuning, and how to organize, save, and manage these datasets. We will demonstrate a few finetuning configurations, show you how to monitor and evaluate your finetuned LLMs, and collect further datasets to improve your finetuned LLM over time.
Talk By: Brian Law, Sr Specialist Solutions Architect, Databricks ; Nathan Azrak, Senior Machine Learning Engineer, Atlassian
Here's more to explore:
Talk By: Brian Law, Sr Specialist Solutions Architect, Databricks ; Nathan Azrak, Senior Machine Learning Engineer, Atlassian
Here's more to explore:
LLMs in Production: Fine-Tuning, Scaling, and Evaluation at Atlassian
Efficiently Scaling and Deploying LLMs // Hanlin Tang // LLM's in Production Conference
How Do You Scale to Billions of Fine-Tuned LLMs
How Large Language Models Work
Building Production-Ready RAG Applications: Jerry Liu
Finetuning Open-Source LLMs // Sebastian Raschka // LLMs in Production Conference 3 Keynote 1
How to Fine-Tune your Large Language Models (LLMs)
Finetuning LLMs // Greg Diamos // LLMs in Production Conference III Lightning Talk
Train & Fine-Tune Language Models for Production Course by Activeloop, Towards AI & Intel Di...
A Survey of Techniques for Maximizing LLM Performance
Fine-Tuning LLMs: Best Practices and When to Go Small // Mark Kim-Huang // MLOps Meetup #124
One Billion Times Faster Finetuning with Lamini PEFT #llm #chatgpt
When Do You Use Fine-Tuning Vs. Retrieval Augmented Generation (RAG)? (Guest: Harpreet Sahota)
Pitfalls and Best Practices — 5 lessons from LLMs in Production // Raza Habib // LLMs in Prod Con 2...
What is Prompt Tuning?
Practical Fine-Tuning of LLMs
Demo: LLM Serverless Fine-Tuning With Snowflake Cortex AI | Summit 2024
fine tuning LLMs - the 6 stages
[1hr Talk] Intro to Large Language Models
LLM Module 4: Fine-tuning and Evaluating LLMs | 4.9 Evaluating LLMs
From Idea to Production AI Infra for Scaling LLM Apps
What is Retrieval-Augmented Generation (RAG)?
From Idea to Production: AI Infra for Scaling LLM Apps
Fine-Tuning LLMs Without Expensive GPUs
Комментарии