filmov
tv
Mikhail Belkin: From classical statistics to modern deep learning
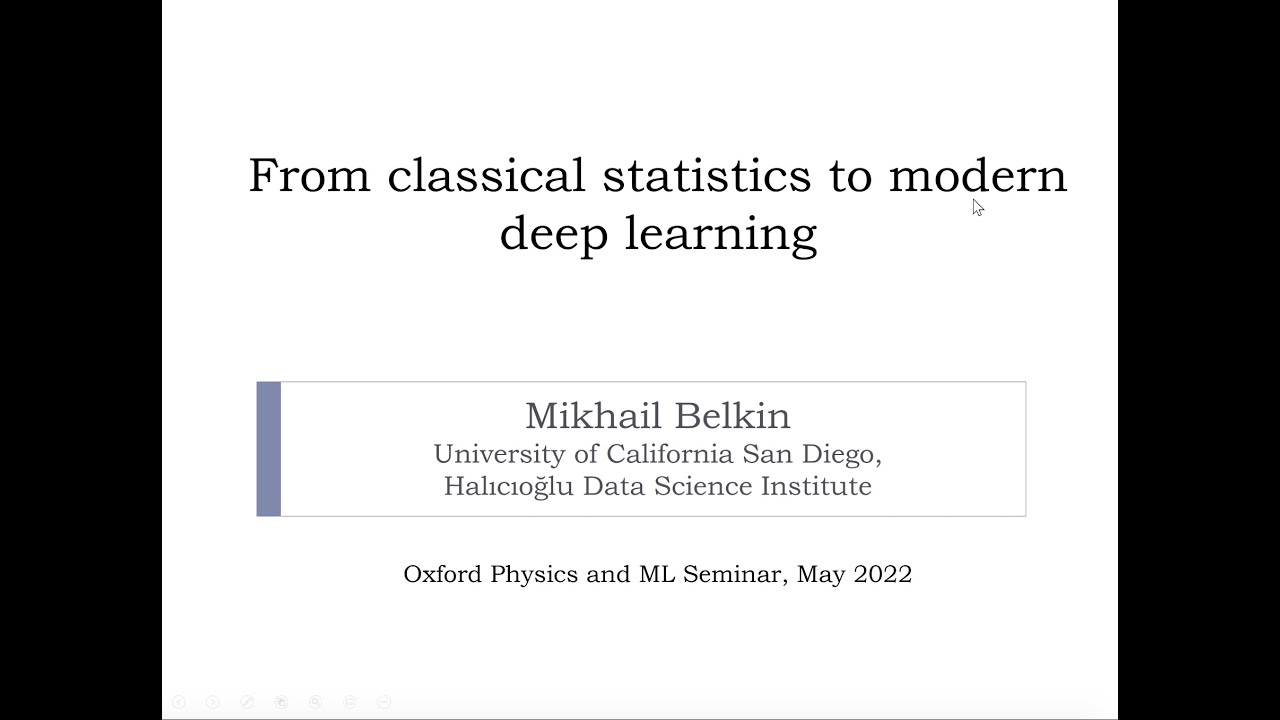
Показать описание
Recent empirical successes of deep learning have exposed significant gaps in our fundamental understanding of learning and optimization mechanisms. Modern best practices for model selection are in direct contradiction to the methodologies suggested by classical analyses. Similarly, the efficiency of SGD-based local methods used in training modern models, appeared at odds with the standard intuitions on optimization. In this talk, Mikhail Belkin (UC San Diego) presents evidence, empirical and mathematical, that necessitates revisiting classical statistical notions, such as over-fitting. He discusses the emerging understanding of generalization, and, in particular, the "double descent" risk curve, which extends the classical U-shaped generalization curve beyond the point of interpolation.
Mikhail Belkin: From classical statistics to modern deep learning
From Classical Statistics to Modern ML: the Lessons of Deep Learning - Mikhail Belkin
Data Science Week 2022: Prof. Mikhail Belkin - From classical statistics to modern deep learning
Mikhail Belkin - From classical bias-variance trade-off to double descent
1W-MINDS: Mikhail Belkin, Feb 18, 2021, A theory of optimization and transition to linearity in...
Mikhail Belkin: 'Optimization for over-parameterized systems of non-linear equations'
Fit Without Fear: An Over-Fitting Perspective on Modern Deep and Shallow Learning
Day 5: Lecture - Theory of DL with Mikhail Belkin
From Classical Statistics to Modern Machine Learning
Distinguished Seminar in Optimization and Data: Mikhail Belkin (UCSD)
From Classical Statistics to Modern Deep Learning [in Russian]
CLIMB talk with Mikhail Belkin: Toward a Practical Theory of Deep Learning: Feature Learning...
Mikhail BELKIN, What can we learn from deep learning?
Stability of overparametrized learning models
IDSS Distinguished Speaker Seminar Series - Mikhail Belkin, UC San Diego
A conversation with Prof. Mikhail Belkin
The Power and Limitations of Kernel Learning
ML Basics and Kernel Methods (Tutorial) by Mikhail Belkin
Misha Belkin - The elusive generalization and easy optimization, Pt. 1 of 2 - IPAM at UCLA
EWSC: The challenges of training infinitely large neural networks, Mikhail Belkin
FDS Virtual Talk by Mikhail Belkin
Beyond Empirical Risk Minimization: the lessons of deep learning
ICAVS 8 | Mikhail Belkin
Learning a Hidden Basis through Imperfect Measurements: Why and How
Комментарии