filmov
tv
Talk: Enhancing classification of autism spectrum disorder using resting-state fMRI with machine le…
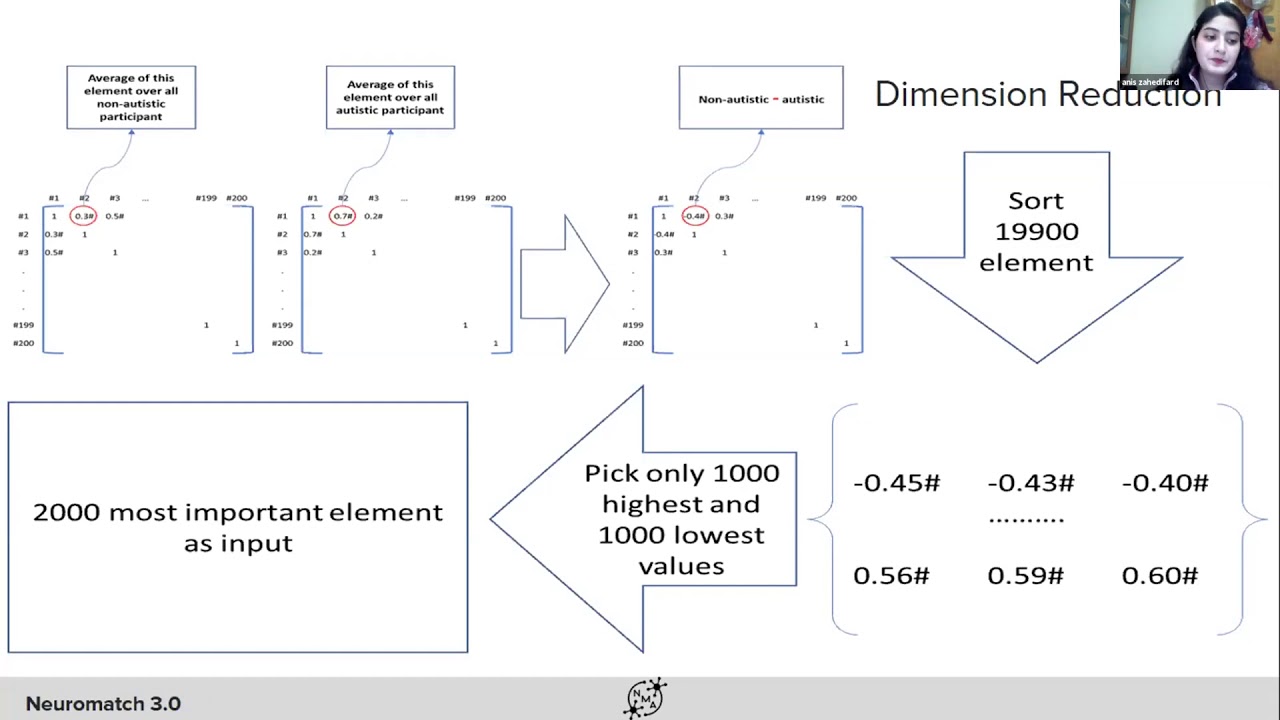
Показать описание
Speaker: Anis Zahedifard, University of Tehran (grid.46072.37)
Title: Enhancing classification of autism spectrum disorder using resting-state fMRI with machine learning approach and dimensionlity reduction
Emcee: Alex Hernandez-Garcia
Backend host: Melvin Selim Atay
Presented during Neuromatch Conference 3.0, Oct 26-30, 2020.
Summary: Autism spectrum disorder is a condition related to brain development that impacts how a person perceives and socializes with others. Early diagnosis of autism disorder involves in-depth behavioral observation and testing, which can be time consuming, expensive, and affected by clinician diagnostic bias. Early diagnosis of ASD using non-behavioral measurements can lead to improved outcomes for children with ASD. We developed algorithms to classify ASD based on publicly available brain-imaging datasets (ABIDE), including brain scans from people with and without ASD diagnosis. Using a multilayer perceptron neural network, we classified preprocessed data from 200 regions of interest (atlas of craddock 200) into binary categories of ASD positive or negative. To overcome overfitting of the training dataset, we used a novel approach to determine the most relevant subset of the preprocessed data. This led to a significant increase in our held-out testing accuracy. Our results indicate that brain imaging data alone, without any behavioral assessments, can be used to classify ASD diagnosis using dimensionality reduction and machine learning techniques. This has the potential to improve early diagnosis of ASD.
Title: Enhancing classification of autism spectrum disorder using resting-state fMRI with machine learning approach and dimensionlity reduction
Emcee: Alex Hernandez-Garcia
Backend host: Melvin Selim Atay
Presented during Neuromatch Conference 3.0, Oct 26-30, 2020.
Summary: Autism spectrum disorder is a condition related to brain development that impacts how a person perceives and socializes with others. Early diagnosis of autism disorder involves in-depth behavioral observation and testing, which can be time consuming, expensive, and affected by clinician diagnostic bias. Early diagnosis of ASD using non-behavioral measurements can lead to improved outcomes for children with ASD. We developed algorithms to classify ASD based on publicly available brain-imaging datasets (ABIDE), including brain scans from people with and without ASD diagnosis. Using a multilayer perceptron neural network, we classified preprocessed data from 200 regions of interest (atlas of craddock 200) into binary categories of ASD positive or negative. To overcome overfitting of the training dataset, we used a novel approach to determine the most relevant subset of the preprocessed data. This led to a significant increase in our held-out testing accuracy. Our results indicate that brain imaging data alone, without any behavioral assessments, can be used to classify ASD diagnosis using dimensionality reduction and machine learning techniques. This has the potential to improve early diagnosis of ASD.