filmov
tv
Building an Optimized ML Pipeline: The builders behind Superbet’s profanity detection use case
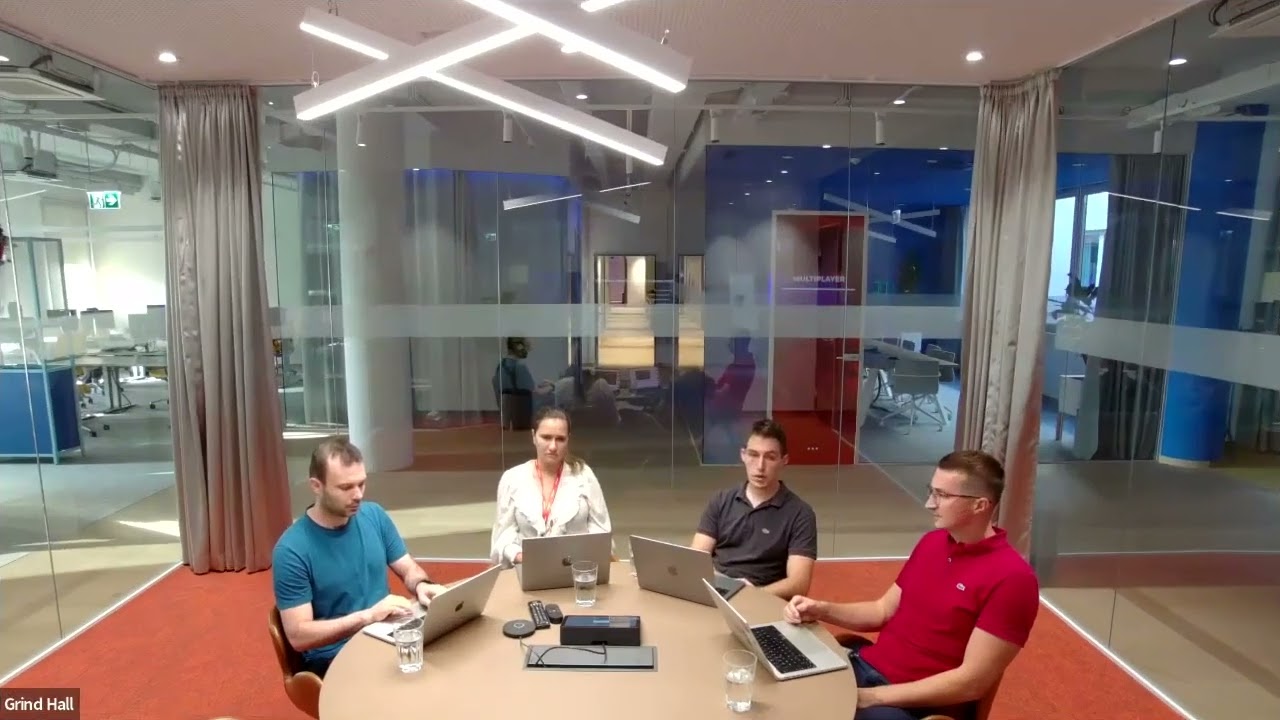
Показать описание
Join us for a live webinar hosted by Qwak and featuring members of Happening's data science and ML engineering teams discussing how they built Superbet's profanity detection model.
During this session, we will discuss:
✔️ How the team ensured fallback variations for multi-language models without duplicating efforts
✔️ What best practices have been implemented for optimizing workflows and reducing operational efforts when re-deploying ML models.
✔️ How all this was implemented in Superbest's profanity model architecture, designed to identify profane messages in chat messages.
This will give you valuable insights into how Happening manages their ML pipeline and how you can optimize your own.
If you're a data scientist, ML engineer, or anyone interested in learning how to optimize ML pipelines for multiple audiences, this webinar is for you. Don't miss this opportunity to learn from the experts at Happening and improve your ML pipeline.
During this session, we will discuss:
✔️ How the team ensured fallback variations for multi-language models without duplicating efforts
✔️ What best practices have been implemented for optimizing workflows and reducing operational efforts when re-deploying ML models.
✔️ How all this was implemented in Superbest's profanity model architecture, designed to identify profane messages in chat messages.
This will give you valuable insights into how Happening manages their ML pipeline and how you can optimize your own.
If you're a data scientist, ML engineer, or anyone interested in learning how to optimize ML pipelines for multiple audiences, this webinar is for you. Don't miss this opportunity to learn from the experts at Happening and improve your ML pipeline.
Building an Optimized ML Pipeline: The builders behind Superbet’s profanity detection use case
What is Data Pipeline? | Why Is It So Popular?
Building an Optimized ML Pipeline: The builders behind Superbet’s profanity detection use case
How to build more efficient ML Pipelines
Creating an Optimized Data Pipeline for Data-Heavy Applications // Subsurface Summer 2020
AutoMLPipeline: A ToolBox for Building ML Pipelines | Paulito Palmes | JuliaCon 2020
Festo.IO_2023 - Automation and Optimization of ML Pipelines
Building a Secure and Transparent ML Pipeline Using Open Source Technologies
Making Generative AI and Enterprise Agents Work: Strategies for Success
Mastering Machine Learning – Ep.2: Build and Run a Machine Learning Pipeline
Deploying Optimized Deep Learning Pipelines
Data Pipelines Explained
Building a Machine Learning Pipeline with Python and Scikit-Learn | Step-by-Step Tutorial
Build unified batch and streaming pipelines on popular ML frameworks
Building an optimization pipeline in Parallel AI
@IndeedEng: Building an Integrated Machine Learning Pipeline
Accelerate Your ML Pipeline with AutoML and MLflow
Build Efficient, High-performance Data Pipelines with IICS
Optimizing Terascale Machine Learning Pipelines With KeystoneML
How to Design and Build a Recommendation System Pipeline in Python (Jill Cates)
Build your machine learning pipeline with Kubeflow
Building Large Scale Machine Learning Applications with Pipelines - Evan Sparks (UC Berkeley AMPLAB)
Webinar recording: Optimizing Machine Learning Pipelines with JSON-AI and MindsDB
2024 LLVM Dev Mtg - What we learned from building Mojo’s optimization pipeline
Комментарии