filmov
tv
QSI Seminar: Guillaume Verdon, X, Quantum-probabilistic Generative Models, 19/06/2020
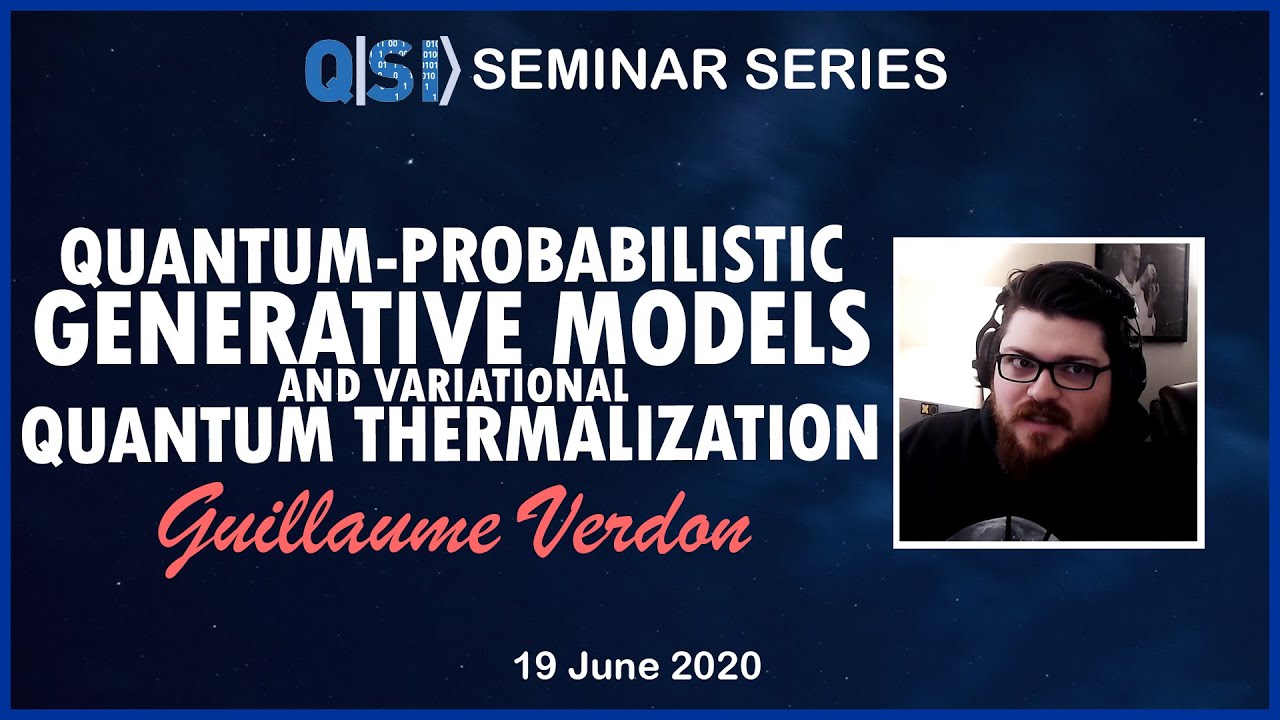
Показать описание
Building better deep learning representations for quantum mixed states by adding quantum layers to classical probabilistic models.
TITLE: Quantum-probabilistic Generative Models and Variational Quantum Thermalization
SPEAKER: Guillaume Verdon
AFFILIATION: X (formerly Google X), California, USA
HOSTED BY: A/Prof. Chris Ferrie, Centre for Quantum Software and Information
ABSTRACT:
We introduce a new class of generative quantum-neural-network-based models called Quantum Hamiltonian-Based Models (QHBMs). In doing so, we establish a paradigmatic approach for quantum-probabilistic hybrid variational learning of quantum mixed states, where we efficiently decompose the tasks of learning classical and quantum correlations in a way which maximizes the utility of both classical and quantum processors. In addition, we introduce the Variational Quantum Thermalizer (VQT) algorithm for generating the thermal state of a given Hamiltonian and target temperature, a task for which QHBMs are naturally well-suited. The VQT can be seen as a generalization of the Variational Quantum Eigensolver (VQE) to thermal states: we show that the VQT converges to the VQE in the zero temperature limit. We provide numerical results demonstrating the efficacy of these techniques in several illustrative examples. In addition to the introduction to the theory and applications behind these models, we will briefly walk through their numerical implementation in TensorFlow Quantum.
RELATED ARTICLES:
OTHER LINKS:
TITLE: Quantum-probabilistic Generative Models and Variational Quantum Thermalization
SPEAKER: Guillaume Verdon
AFFILIATION: X (formerly Google X), California, USA
HOSTED BY: A/Prof. Chris Ferrie, Centre for Quantum Software and Information
ABSTRACT:
We introduce a new class of generative quantum-neural-network-based models called Quantum Hamiltonian-Based Models (QHBMs). In doing so, we establish a paradigmatic approach for quantum-probabilistic hybrid variational learning of quantum mixed states, where we efficiently decompose the tasks of learning classical and quantum correlations in a way which maximizes the utility of both classical and quantum processors. In addition, we introduce the Variational Quantum Thermalizer (VQT) algorithm for generating the thermal state of a given Hamiltonian and target temperature, a task for which QHBMs are naturally well-suited. The VQT can be seen as a generalization of the Variational Quantum Eigensolver (VQE) to thermal states: we show that the VQT converges to the VQE in the zero temperature limit. We provide numerical results demonstrating the efficacy of these techniques in several illustrative examples. In addition to the introduction to the theory and applications behind these models, we will briefly walk through their numerical implementation in TensorFlow Quantum.
RELATED ARTICLES:
OTHER LINKS: