filmov
tv
Stanford CS25: V4 I Jason Wei & Hyung Won Chung of OpenAI
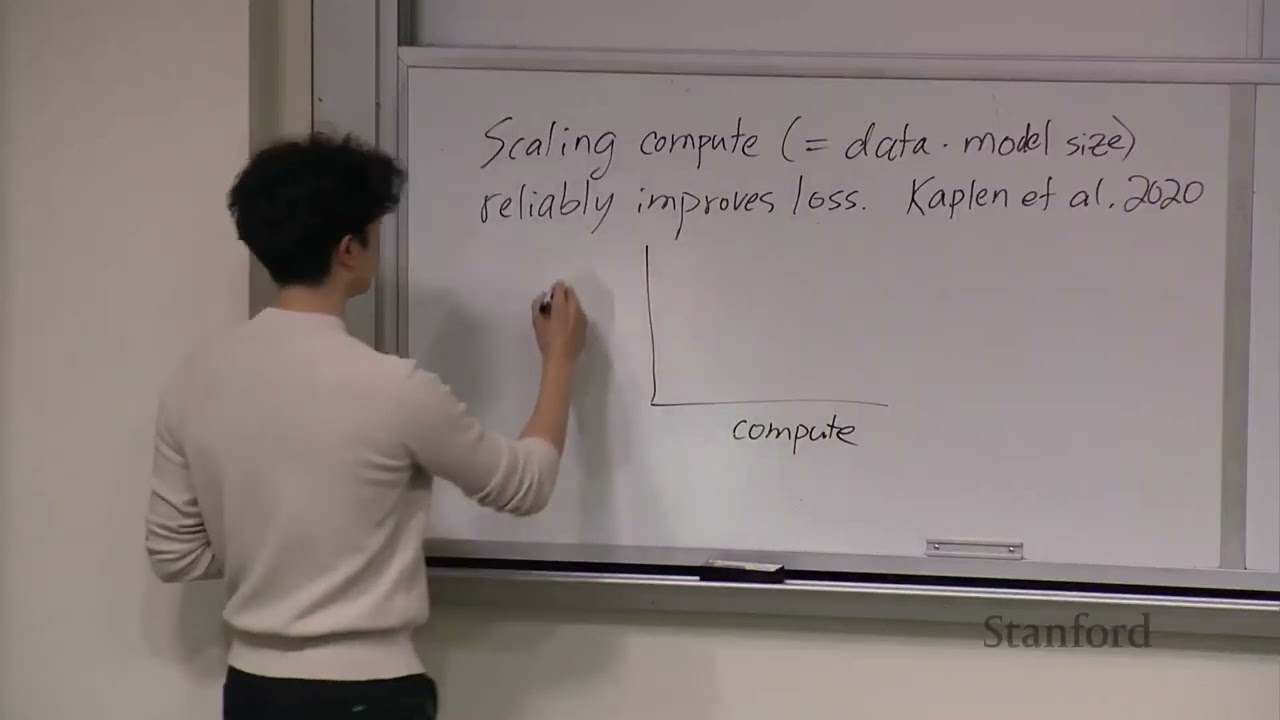
Показать описание
April 11, 2024
Speakers: Jason Wei & Hyung Won Chung, OpenAI
Intuitions on Language Models (Jason)
Shaping the Future of AI from the History of Transformer (Hyung Won)
About the speakers:
Jason Wei is an AI researcher based in San Francisco. He is currently working at OpenAI. He was previously a research scientist at Google Brain, where he popularized key ideas in large language models such as chain-of-thought prompting, instruction tuning, and emergent phenomena.
Hyung Won Chung is a research scientist at OpenAI ChatGPT team. He has worked on various aspects of Large Language Models: pre-training, instruction fine-tuning, reinforcement learning with human feedback, reasoning, multilinguality, parallelism strategies, etc. Some of the notable work includes scaling Flan paper (Flan-T5, Flan-PaLM) and T5X, the training framework used to train the PaLM language model. Before OpenAI, he was at Google Brain and before that he received a PhD from MIT.
Speakers: Jason Wei & Hyung Won Chung, OpenAI
Intuitions on Language Models (Jason)
Shaping the Future of AI from the History of Transformer (Hyung Won)
About the speakers:
Jason Wei is an AI researcher based in San Francisco. He is currently working at OpenAI. He was previously a research scientist at Google Brain, where he popularized key ideas in large language models such as chain-of-thought prompting, instruction tuning, and emergent phenomena.
Hyung Won Chung is a research scientist at OpenAI ChatGPT team. He has worked on various aspects of Large Language Models: pre-training, instruction fine-tuning, reinforcement learning with human feedback, reasoning, multilinguality, parallelism strategies, etc. Some of the notable work includes scaling Flan paper (Flan-T5, Flan-PaLM) and T5X, the training framework used to train the PaLM language model. Before OpenAI, he was at Google Brain and before that he received a PhD from MIT.
Комментарии