filmov
tv
Antti Honkela: Accurate privacy accounting for differentially private machine learning
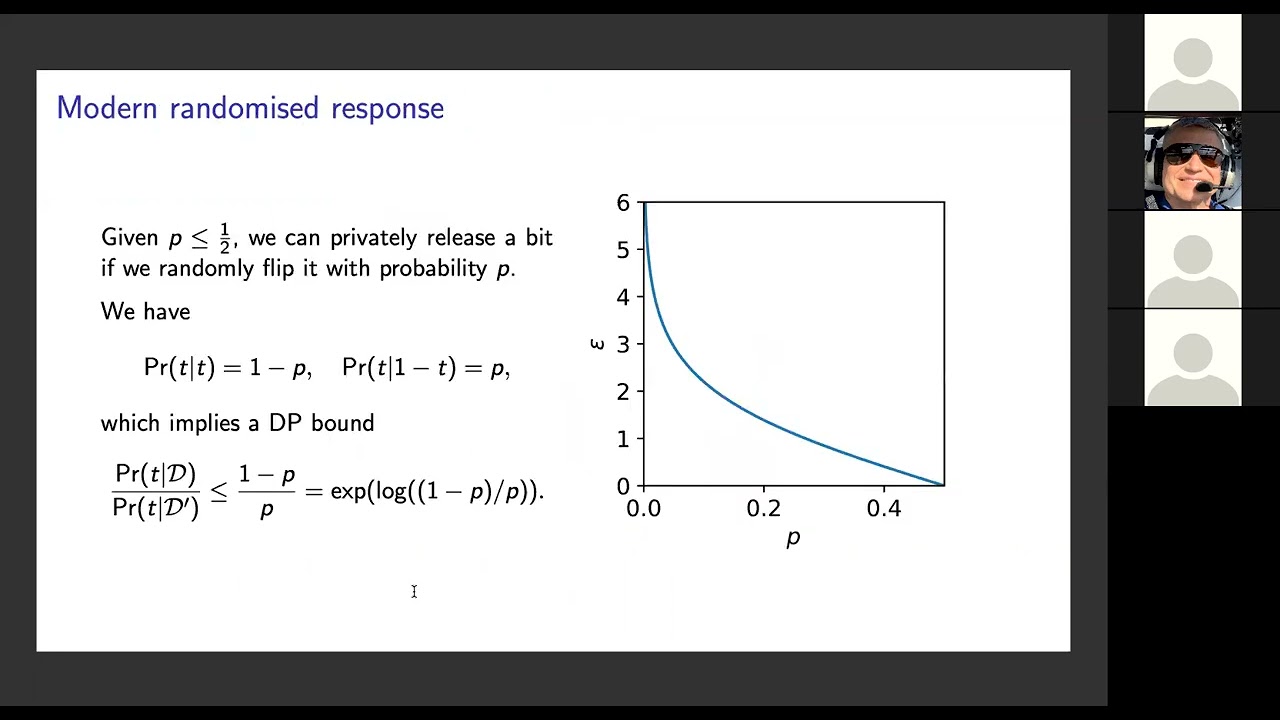
Показать описание
Differential privacy (DP) has recently emerged as the standard foundation for privacy-preserving machine learning and data analytics. One important theoretical property of DP is compositionality: releasing the results of multiple DP mechanisms satisfies DP with weaker privacy. Quantifying composition privacy in different situations, also known as privacy accounting, is an important research topic as many prominent applications of DP in machine learning, such as training a neural network under DP, can require compositions of thousands of atomic mechanisms. Over the past decade increasingly accurate composition theorems and privacy accountants have been developed, culminating in our introduction of a numerical method allowing arbitrarily accurate accounting (Koskela et al., AISTATS 2021). In my talk I will introduce this method along with some more recent developments.