filmov
tv
Equivariant Neural Networks | Part 1/3 - Introduction
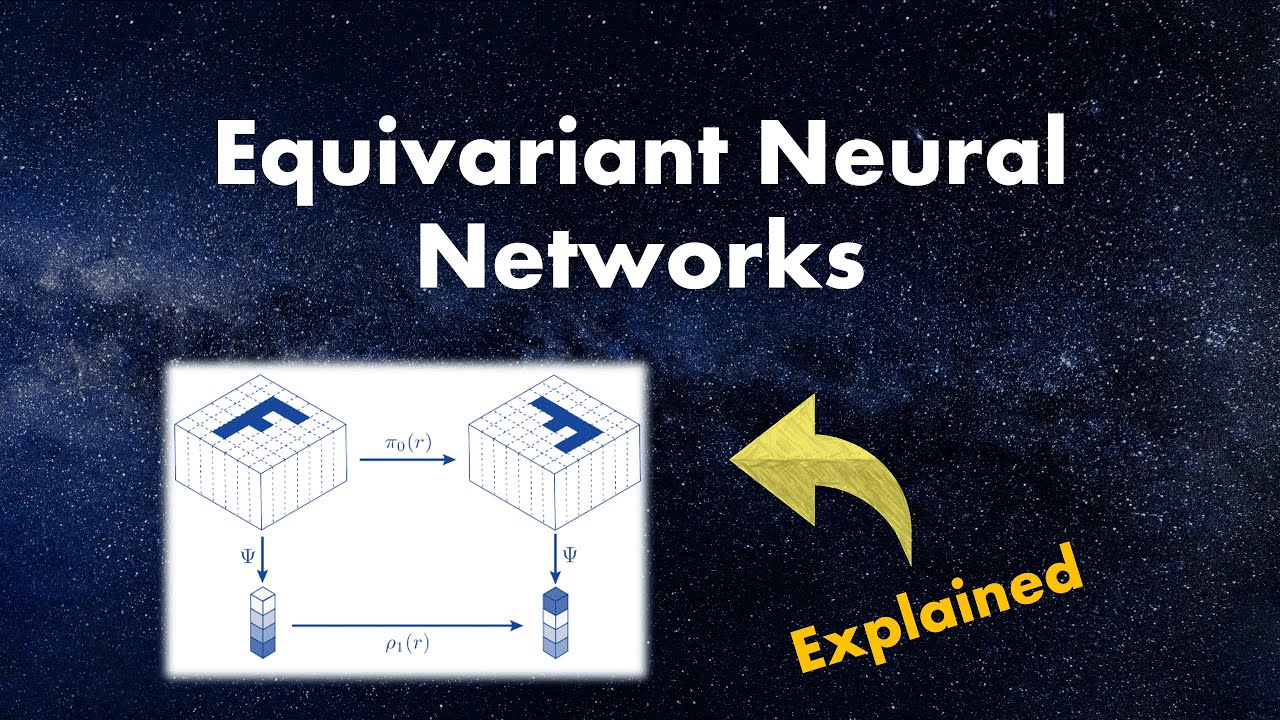
Показать описание
▬▬ Papers / Resources ▬▬▬
▬▬ Used Music ▬▬▬▬▬▬▬▬▬▬▬
Music from #Uppbeat (free for Creators!):
License code: WXVHOOZRRWDUCKIU
▬▬ Used Icons ▬▬▬▬▬▬▬▬▬▬
▬▬ Timestamps ▬▬▬▬▬▬▬▬▬▬▬
00:00 Introduction
00:45 Equivariance and Invariance
03:03 CNNs are translation equivariant
04:00 Math notation
04:25 Visual intuition
05:08 Symmetries
06:22 Why are CNNs not rotation equivariant?
07:14 Inductive biases reduce the flexibility
08:10 What's wrong with data augmentations?
09:32 Motivations for Equivariant Neural Networks
09:55 You've unlocked a checkpoint.
10:07 Naturally occuring equivariance
10:50 Group Equivariant Convolutional Neural Networks
11:37 Group Theory (on a high level)
12:41 An example and the matrix notation
13:50 Group axioms
14:32 Cayley tables
15:33 Examples for groups
16:38 Applications of Equivariant Neural Networks
18:30 Final Checkpoint :)
▬▬ Support me if you like 🌟
▬▬ My equipment 💻
▬▬ Used Music ▬▬▬▬▬▬▬▬▬▬▬
Music from #Uppbeat (free for Creators!):
License code: WXVHOOZRRWDUCKIU
▬▬ Used Icons ▬▬▬▬▬▬▬▬▬▬
▬▬ Timestamps ▬▬▬▬▬▬▬▬▬▬▬
00:00 Introduction
00:45 Equivariance and Invariance
03:03 CNNs are translation equivariant
04:00 Math notation
04:25 Visual intuition
05:08 Symmetries
06:22 Why are CNNs not rotation equivariant?
07:14 Inductive biases reduce the flexibility
08:10 What's wrong with data augmentations?
09:32 Motivations for Equivariant Neural Networks
09:55 You've unlocked a checkpoint.
10:07 Naturally occuring equivariance
10:50 Group Equivariant Convolutional Neural Networks
11:37 Group Theory (on a high level)
12:41 An example and the matrix notation
13:50 Group axioms
14:32 Cayley tables
15:33 Examples for groups
16:38 Applications of Equivariant Neural Networks
18:30 Final Checkpoint :)
▬▬ Support me if you like 🌟
▬▬ My equipment 💻
Equivariant Neural Networks | Part 1/3 - Introduction
Equivariant Neural Networks | Part 2/3 - Generalized CNNs
Equivariant Neural Networks | Part 3/3 - Transformers and GNNs
Equivariant Neural Networks (SGP Graduate School 2024)
Equivariant Neural Networks for Learning Spatiotemporal Dynamics, R.Walters, Northeastern University
A Complete Beginner's Guide To G-Invariant and G-Equivariant Neural Networks (02/08/2024) - Par...
Geometric Deep Learning, visualising a forward pass through an equivariant neural network
Group Equivariant Deep Learning - Lecture 3.1: Motivation for SE(3) equivariant graph NNs
Clifford Group Equivariant Neural Networks | David Ruhe
Part 3: E(n) Equivariant Graph Neural Networks
Group Equivariant Deep Learning - Lecture 3.2: Equivariant message passing as non-linear convolution
Equivariant Subgraph Aggregation Networks | Beatrice Bevilacqua, Fabrizio Frasca & Derek Lim
A Complete Beginner's Guide To G-Invariant and G-Equivariant Neural Networks (02/08/2024) - Par...
G-invariant Neural Networks (part 1)
E($3$) Equivariant Graph Neural Networks for Particle-Based Fluid Mechanics - ArXiv:2304
E(n) Equivariant Graph Neural Networks - ECS 289G Talk
A Complete Beginner's Guide To G-Invariant and G-Equivariant Neural Networks (02/09/2024)
CNNs on Surfaces using Rotation-equivariant Features
Enhancing interpretability of equivariant neural networks... - Hao Xu - 3DSIG - Poster - ISMB 2022
E(3)-equivariant graph neural networks for data-efficient and accurate interatomic po... | RTCL.TV
Equivariant Machine Learning
Rotation Equivariant Deep Neural Network (RED-NN)
Equivariant Networks and Natural Graph Networks - Taco Cohen
Group Equivariant Deep Learning - Lecture 1.3: Regular group convolutional neural networks
Комментарии