filmov
tv
NIPS 2011 Domain Adaptation Workshop: Adaptation without Retraining
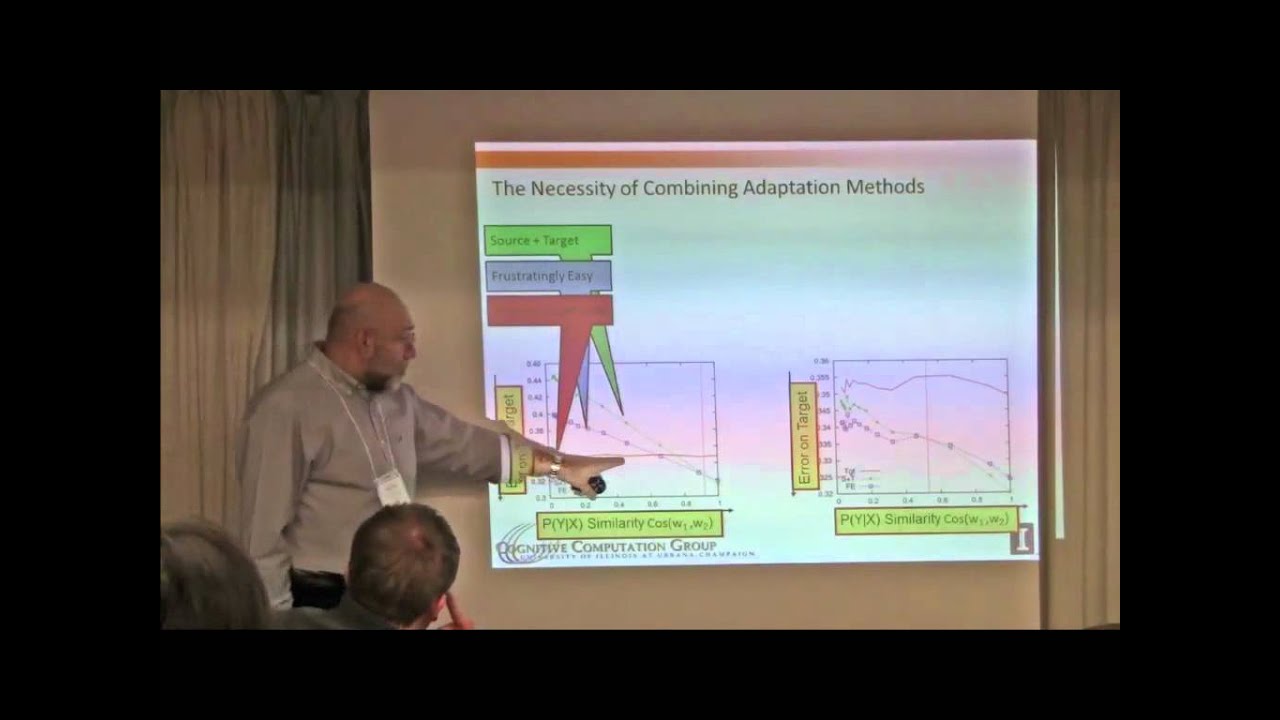
Показать описание
Domain Adaptation Workshop: Theory and Application at NIPS 2011
Invited Speaker: Adaptation without Retraining by Dan Roth
Dan Roth is a Professor in the Department of Computer Science and the Beckman Institute at the University of Illinois at Urbana-Champaign and a University of Illinois Scholar. He is also a Fellow of AAAI, for his contributions to the foundations of machine learning and inference and for developing learning centered solutions for natural language processing problems.
Abstract: Natural language models trained on labeled data from one domain do not perform well on other domains. Most adaptation algorithms proposed in the literature train a new model for the target domain using a mix of labeled and unlabeled data. We discuss some limitations of existing general purpose adaptation algorithms that are due to the interaction betweendifferences in base feature statistics and task differences and illustrate howthis should be taken into account jointly.
With these insights we propose a new approach to adaptation that avoids the need for retraining models. I nstead, at evaluation time, we perturb the given instance to be more similar to instances the model can h andle well, or perturb the model outcomes to fit our expectation of the target domain better, given some prior knowledge on the task and the target domain.
We provide experimental evidence in a range of natural language processing, including semantic role labeling and English as a Second Language (ESL) text correction tasks.
Invited Speaker: Adaptation without Retraining by Dan Roth
Dan Roth is a Professor in the Department of Computer Science and the Beckman Institute at the University of Illinois at Urbana-Champaign and a University of Illinois Scholar. He is also a Fellow of AAAI, for his contributions to the foundations of machine learning and inference and for developing learning centered solutions for natural language processing problems.
Abstract: Natural language models trained on labeled data from one domain do not perform well on other domains. Most adaptation algorithms proposed in the literature train a new model for the target domain using a mix of labeled and unlabeled data. We discuss some limitations of existing general purpose adaptation algorithms that are due to the interaction betweendifferences in base feature statistics and task differences and illustrate howthis should be taken into account jointly.
With these insights we propose a new approach to adaptation that avoids the need for retraining models. I nstead, at evaluation time, we perturb the given instance to be more similar to instances the model can h andle well, or perturb the model outcomes to fit our expectation of the target domain better, given some prior knowledge on the task and the target domain.
We provide experimental evidence in a range of natural language processing, including semantic role labeling and English as a Second Language (ESL) text correction tasks.