filmov
tv
Model Lifecycle Management | Introduction to DataRobot AI Production
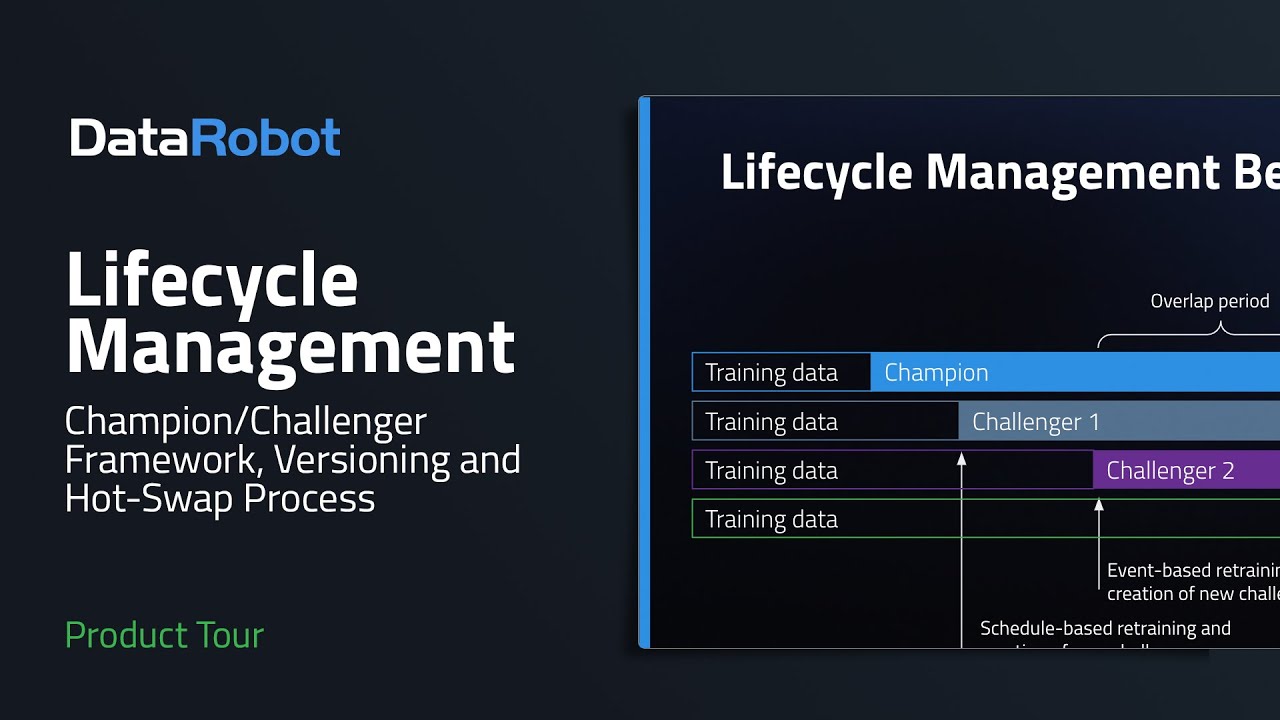
Показать описание
Model lifecycle management in mature organization is a continuous process of model retraining, production model hot-swaps, and model archiving. Keeping up with it all is only possible with automation. That’s where DataRobot comes in.
Learn more
Content
In this tutorial you'll learn about model lifecycle management with DataRobt. This includes an introduction to the Champion/Challenger framework, Automated retraining policies and hot-swaps of models in production.
This is important because value from AI is closely intertwined with keeping models current and avoiding business disruptions and fire drills when models need to be replaced.
DataRobot makes it easy to adopt and adhere to Lifecycle Management best practices. Suppose your organization has the blue model labeled Champion running in production. Based on a schedule you define, a new model is created with additional training data that has become available and added as the Grey Challenger. Then degradation of the Champion below your threshold accuracy triggers the retraining of the purple model and it is added as a challenger. There is a period of overlap for the three models leading to a decision date. On the hot-swap date the purple model is promoted to Champion and the blue and grey models are archived. The purple model is now in production but notably has not been trained on all the available data. So the cycle repeats itself with a scheduled retraining that results in the green model as a challenger. This is how a mature organization operates with constant trigger-based retraining, overlap periods, model hot-swaps and model archiving. Keeping up with it all is only possible with automation. That’s where DataRobot comes in.
I’m inside a single deployment in DataRobot. This deployment has undergone numerous model replacements which are automatically recorded in the log. If necessary for an audit or investigation, any user can access archived models from the past.
This deployment has 2 active retraining policies - one based on the calendar and one based on model accuracy. New retraining policies only take a few minutes to configure and then will run continuously until halted. Model decline triggers can be based on Accuracy or Data Drift.
You have very fine control over the retraining process. Selected between repeating the entire DataRobot AutoPilot model competition, or re-run only the current champion with the same (or updated hyperparameters). Choose which of three actions DataRobot should take when retraining concludes - Register the model, add as challenger or initiate model replacement.
If you choose to add as a Challenger, monitor the multiple models during an overlap period. In this two month overlap, the purple model’s RMSE is consistently lower than the turquoise champion model. Lower is better so the purple model should be promoted. Initiate the hot swap from the double arrows. Provide a reason for the replacement front he drop-down list. The view you see next will depend upon the governance and approval workflows that your organizations has created. In this example, I have the ability to finalize this replacement without approvals from other users.
The final part of model lifecycle management to demonstrate is keeping training data fresh. On the Setting tab, select one single dataset from AI Catalog as the retraining data for the deployment. Then use the scheduler within AI Catalog to periodically refresh the dataset. If the initial data set pulled from an enterprise system such as Snowflake, BigQuery or similar, then each refresh will use the same connection and there is more manual intervention required. Retraining will default to use the most recent version in the version history.
That’s how DataRobot is able to automate this entire story. The training data refreshes are scheduled and automated. The challenger creation is scheduled or event based. Both are fully automated. The hot swap maintains the same API endpoint for the deployment and does not disrupt any scoring jobs connected to the deployment. Finally, all changes are recorded in the log.
Stay connected with DataRobot!
Learn more
Content
In this tutorial you'll learn about model lifecycle management with DataRobt. This includes an introduction to the Champion/Challenger framework, Automated retraining policies and hot-swaps of models in production.
This is important because value from AI is closely intertwined with keeping models current and avoiding business disruptions and fire drills when models need to be replaced.
DataRobot makes it easy to adopt and adhere to Lifecycle Management best practices. Suppose your organization has the blue model labeled Champion running in production. Based on a schedule you define, a new model is created with additional training data that has become available and added as the Grey Challenger. Then degradation of the Champion below your threshold accuracy triggers the retraining of the purple model and it is added as a challenger. There is a period of overlap for the three models leading to a decision date. On the hot-swap date the purple model is promoted to Champion and the blue and grey models are archived. The purple model is now in production but notably has not been trained on all the available data. So the cycle repeats itself with a scheduled retraining that results in the green model as a challenger. This is how a mature organization operates with constant trigger-based retraining, overlap periods, model hot-swaps and model archiving. Keeping up with it all is only possible with automation. That’s where DataRobot comes in.
I’m inside a single deployment in DataRobot. This deployment has undergone numerous model replacements which are automatically recorded in the log. If necessary for an audit or investigation, any user can access archived models from the past.
This deployment has 2 active retraining policies - one based on the calendar and one based on model accuracy. New retraining policies only take a few minutes to configure and then will run continuously until halted. Model decline triggers can be based on Accuracy or Data Drift.
You have very fine control over the retraining process. Selected between repeating the entire DataRobot AutoPilot model competition, or re-run only the current champion with the same (or updated hyperparameters). Choose which of three actions DataRobot should take when retraining concludes - Register the model, add as challenger or initiate model replacement.
If you choose to add as a Challenger, monitor the multiple models during an overlap period. In this two month overlap, the purple model’s RMSE is consistently lower than the turquoise champion model. Lower is better so the purple model should be promoted. Initiate the hot swap from the double arrows. Provide a reason for the replacement front he drop-down list. The view you see next will depend upon the governance and approval workflows that your organizations has created. In this example, I have the ability to finalize this replacement without approvals from other users.
The final part of model lifecycle management to demonstrate is keeping training data fresh. On the Setting tab, select one single dataset from AI Catalog as the retraining data for the deployment. Then use the scheduler within AI Catalog to periodically refresh the dataset. If the initial data set pulled from an enterprise system such as Snowflake, BigQuery or similar, then each refresh will use the same connection and there is more manual intervention required. Retraining will default to use the most recent version in the version history.
That’s how DataRobot is able to automate this entire story. The training data refreshes are scheduled and automated. The challenger creation is scheduled or event based. Both are fully automated. The hot swap maintains the same API endpoint for the deployment and does not disrupt any scoring jobs connected to the deployment. Finally, all changes are recorded in the log.
Stay connected with DataRobot!