filmov
tv
How much sample size is needed for SEM Modeling?
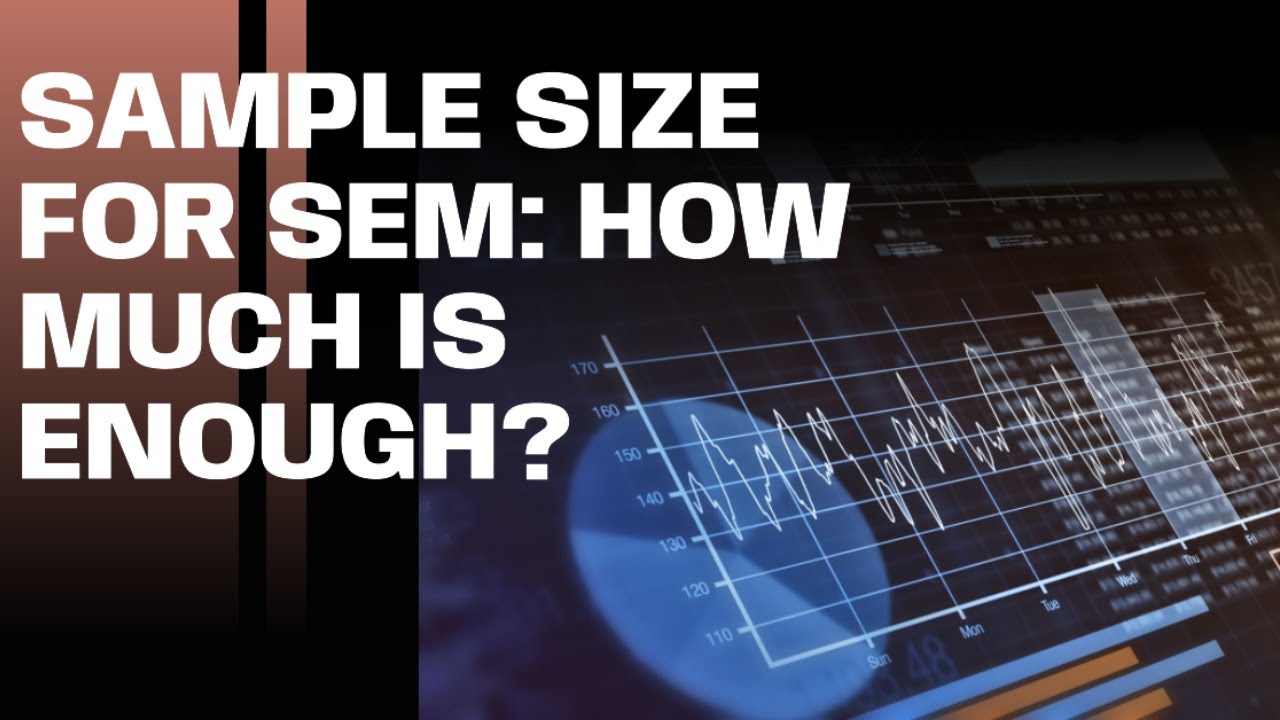
Показать описание
How Much Sample Size is Needed for SEM Modelling?
In this insightful video, we explore the critical question of how much sample size is required for covariance-based Structural Equation Modelling (SEM). Understanding the appropriate sample size is crucial for ensuring the validity and accuracy of your SEM analysis.
Covariance-based SEM comes with a fundamental assumption - it demands a larger sample size compared to other statistical techniques. To shed light on this important aspect, we delve into well-established rules of thumb for determining the sample size.
Nunnally and Bernstein's (1994) rule of 10 is a widely-known guideline that advises having at least 10 observations for each indicator in your model. Additionally, we discuss Stevens' (1996) suggestion, which recommends a minimum of 15 cases per indicator for robust SEM analysis.
But it doesn't end there - we introduce another key rule of thumb: the "critical sample size" of 200. Supported by research by Garver and Mentzer (1999) and Hoelter (1983), this threshold ensures stable parameter estimates and provides sufficient power to test your model effectively.
However, it's essential to remember that sample size decisions shouldn't be solely based on fixed guidelines. We offer a small suggestion from our side: Consider the complexity of your model and tailor the sample size accordingly, rather than merely settling for the bare minimum.
If you're diving into the world of SEM or seeking to enhance your understanding of sample size determination, this video is a must-watch. Master the art of selecting the appropriate sample size for your covariance-based SEM modelling, and unlock the full potential of your research.
Reference:
Nunnally, Jum C. and Ira H. Bernstein. (1994), Psychometric Theory (3rd ed.). New York, NY: McGraw-Hill.
Stevens, James P. (1996), Applied Multivariate Statistics for Social Sciences. New York, NY: Routledge and Taylor & Francis.
Garver, Michael S. and John T. Mentzer. (1999), “Logistics Research Methods: Employing Structural Equation Modeling to Test for Construct Validity”, Journal of Business Logistics, 20 (1), 33–57.
Hoelter, Jon W. (1983), “The Analysis of Covariance Structures Goodness of Fit Indices”, Sociological Methods Research, 11 (3), 325–344.
Don't miss this opportunity to enhance your SEM knowledge and take your research to the next level. Hit that play button and let's unravel the mystery of sample size in covariance-based SEM!
Please, subscribe the channel, Like and share the video with the other researchers.
What's App +91 9849676109 or +91 8555041411
#DATAANALYTICS #DATASCIENCE #PREDICTIVEANALYTICS #MultivariateAnalysis
List of my other videos which you may be Interested:
Henry Garrett Ranking Method with an Example
Multiple Response Analysis using SPSS Statistics
Exploratory Factor Analysis using SPSS
Friedman test using SPSS
Kruskal Wallis Test is a non-parametric test for independent variables
McNemar's test is a statistical test used on paired nominal data.
Visual Binning in SPSS :
Structural Equation Modeling using SPSS Amos Introduction Part1:
Structural Equation Modeling using Amos Introduction Part1:
Structural Equation Modelling using Amos Software- part 2
Structural Equation Modeling using Amos software - part 3:
Key words:
how to calculate sample size,sample size calculator,minimum sample size,sample size for surveys,sample size for experiments,sample size for statistical analysis,sample size for research,determining sample size,sample size for clinical trials,sample size for SEM,sample size for SEM in psychology,sample size for SEM in marketing,sample size for SEM in education,sample size for SEM in healthcare,sample size for SEM in business,sample size for COVID-19 study
In this insightful video, we explore the critical question of how much sample size is required for covariance-based Structural Equation Modelling (SEM). Understanding the appropriate sample size is crucial for ensuring the validity and accuracy of your SEM analysis.
Covariance-based SEM comes with a fundamental assumption - it demands a larger sample size compared to other statistical techniques. To shed light on this important aspect, we delve into well-established rules of thumb for determining the sample size.
Nunnally and Bernstein's (1994) rule of 10 is a widely-known guideline that advises having at least 10 observations for each indicator in your model. Additionally, we discuss Stevens' (1996) suggestion, which recommends a minimum of 15 cases per indicator for robust SEM analysis.
But it doesn't end there - we introduce another key rule of thumb: the "critical sample size" of 200. Supported by research by Garver and Mentzer (1999) and Hoelter (1983), this threshold ensures stable parameter estimates and provides sufficient power to test your model effectively.
However, it's essential to remember that sample size decisions shouldn't be solely based on fixed guidelines. We offer a small suggestion from our side: Consider the complexity of your model and tailor the sample size accordingly, rather than merely settling for the bare minimum.
If you're diving into the world of SEM or seeking to enhance your understanding of sample size determination, this video is a must-watch. Master the art of selecting the appropriate sample size for your covariance-based SEM modelling, and unlock the full potential of your research.
Reference:
Nunnally, Jum C. and Ira H. Bernstein. (1994), Psychometric Theory (3rd ed.). New York, NY: McGraw-Hill.
Stevens, James P. (1996), Applied Multivariate Statistics for Social Sciences. New York, NY: Routledge and Taylor & Francis.
Garver, Michael S. and John T. Mentzer. (1999), “Logistics Research Methods: Employing Structural Equation Modeling to Test for Construct Validity”, Journal of Business Logistics, 20 (1), 33–57.
Hoelter, Jon W. (1983), “The Analysis of Covariance Structures Goodness of Fit Indices”, Sociological Methods Research, 11 (3), 325–344.
Don't miss this opportunity to enhance your SEM knowledge and take your research to the next level. Hit that play button and let's unravel the mystery of sample size in covariance-based SEM!
Please, subscribe the channel, Like and share the video with the other researchers.
What's App +91 9849676109 or +91 8555041411
#DATAANALYTICS #DATASCIENCE #PREDICTIVEANALYTICS #MultivariateAnalysis
List of my other videos which you may be Interested:
Henry Garrett Ranking Method with an Example
Multiple Response Analysis using SPSS Statistics
Exploratory Factor Analysis using SPSS
Friedman test using SPSS
Kruskal Wallis Test is a non-parametric test for independent variables
McNemar's test is a statistical test used on paired nominal data.
Visual Binning in SPSS :
Structural Equation Modeling using SPSS Amos Introduction Part1:
Structural Equation Modeling using Amos Introduction Part1:
Structural Equation Modelling using Amos Software- part 2
Structural Equation Modeling using Amos software - part 3:
Key words:
how to calculate sample size,sample size calculator,minimum sample size,sample size for surveys,sample size for experiments,sample size for statistical analysis,sample size for research,determining sample size,sample size for clinical trials,sample size for SEM,sample size for SEM in psychology,sample size for SEM in marketing,sample size for SEM in education,sample size for SEM in healthcare,sample size for SEM in business,sample size for COVID-19 study