filmov
tv
Machine Learning and Predictive Analytics Animation
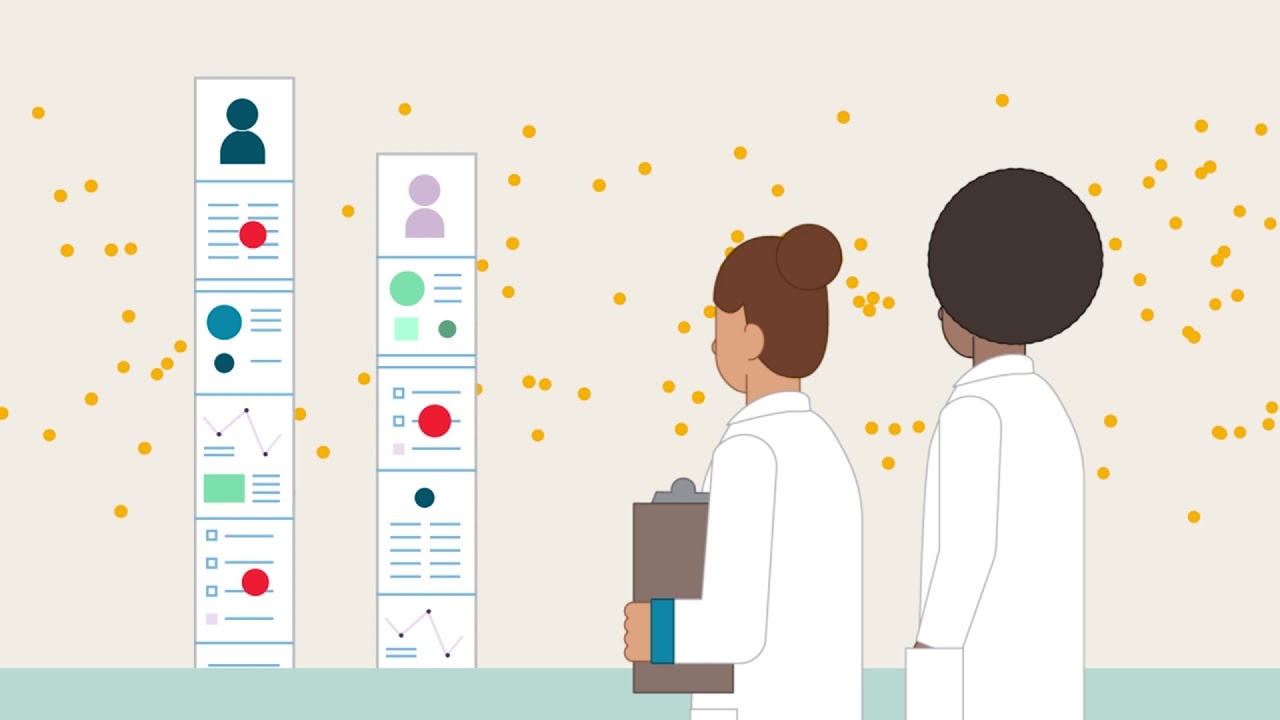
Показать описание
Health Catalyst believes the introduction and widespread use of machine learning in healthcare will be one of the most important, life-saving technologies ever introduced. We believe the opportunities are virtually limitless for the technology to improve and accelerate clinical, workflow, and financial outcomes. The following are just a few examples:
Reduce readmissions. Machine learning can reduce readmissions in a targeted, efficient, and patient-centered manner. Clinicians can receive daily guidance as to which patients are most likely to be readmitted and how they might be able to reduce that risk.
Prevent hospital acquired infections (HAIs). Health systems can reduce HAIs, such as central-line associated bloodstream infections (CLABSIs)—40 percent of CLABSI patients die—by predicting which patients with a central line will develop a CLABSI. Clinicians can monitor high-risk patients and intervene to reduce that risk by focusing on patient-specific risk factors.
Reduce hospital Length-of-Stay (LOS). Health systems can reduce LOS and improve other outcomes like patient satisfaction by identifying patients that are likely to have an increased LOS and then ensure that best practices are followed.
Predict chronic disease. Machine learning can help hospital systems identify patients with undiagnosed or misdiagnosed chronic disease, predict the likelihood that patients will develop chronic disease, and present patient-specific prevention interventions.
Reduce 1-year mortality. Health systems can reduce 1-year mortality rates by predicting the likelihood of death within one year of discharge and then match patients with appropriate interventions, care providers, and support.
Predict propensity-to-pay. Health systems can determine who needs reminders, who needs financial assistance, and how the likelihood of payment changes over time and after particular events.
Predict no-shows. Health systems can create accurate predictive models to assess, with each scheduled appointment, the risk of a no-show, ultimately improving patient care and the efficient use of resources.