filmov
tv
Krzysztof Choromański: Charming kernels, colorful Jacobians and Hadamard-minitaurs
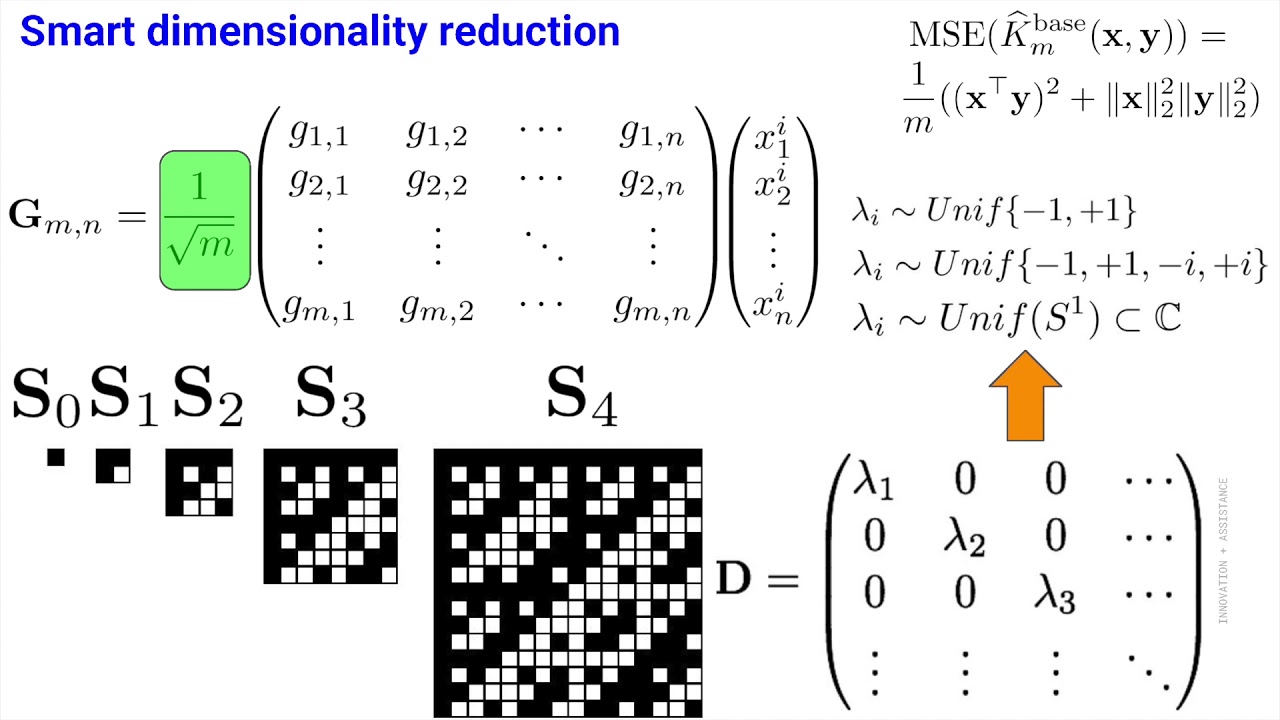
Показать описание
Deep mathematical ideas is what drives innovation in machine learning even though it is often underestimated in the era of massive computations. In this talk we show few mathematical ideas that can be applied in many important and unrelated at first glance machine learning problems. We will talk about speeding up algorithms that approximate certain similarity measures used on a regular basis in machine learning via random walks in the space of orthogonal matrices. We show how these can be also used to improve the accuracy of several machine learning models, among them some recent RNN-based architectures that already beat state-of-the-art LSTMs. We explain how to "backpropagate through robots" with compressed sensing, Hadamard matrices and strongly-polynomial LP-programming. We will teach robots how to walk and show you that what you were taught in school might be actually wrong - there exist free-lunch theorems and after this lecture you will apply them in practice.