filmov
tv
Smart RAG: Domain-Specific Fine-Tuning for End-to-End Retrieval
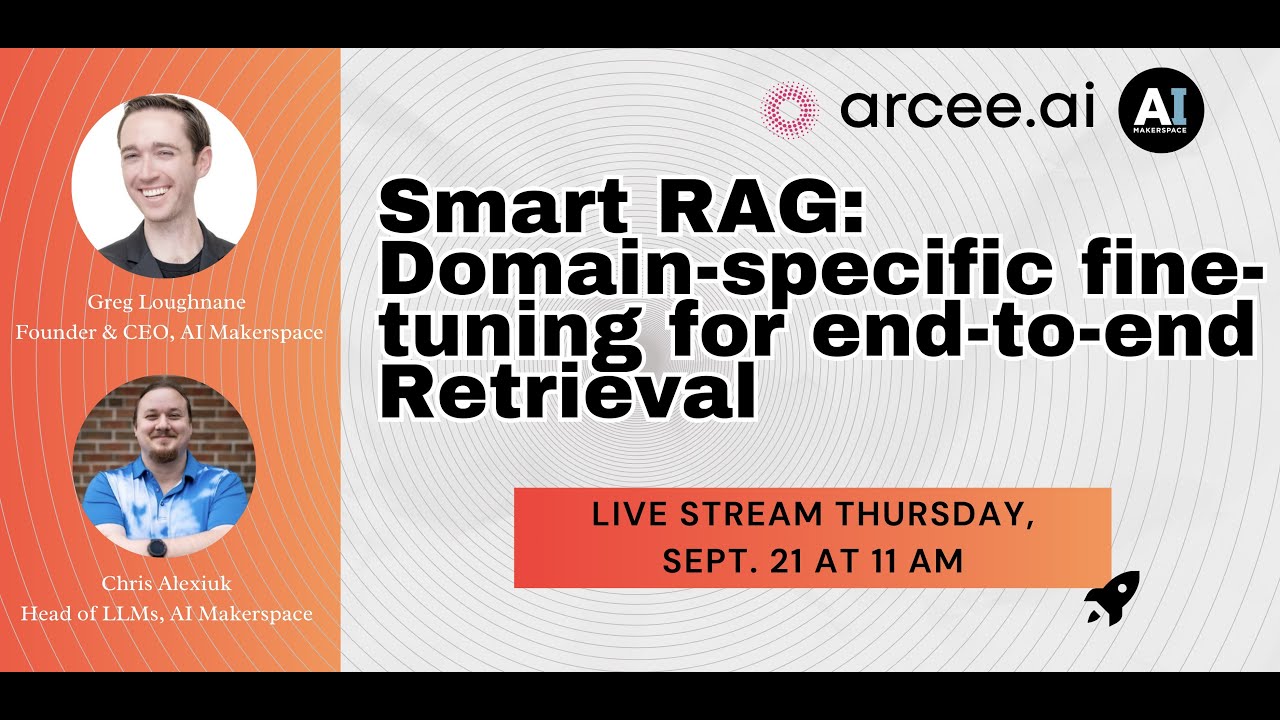
Показать описание
Have a question for a speaker? Drop them here:
Speakers:
Dr. Greg Loughnane, Founder & CEO, AI Makerspace
Chris Alexiuk, CTO, AI Makerspace
Join our community to start building, shipping, and sharing with us today!
Apply to our upcoming LLM Ops: LLMs in production course on Maven today!
How'd we do? Share your feedback and suggestions for future events.
Smart RAG: Domain-Specific Fine-Tuning for End-to-End Retrieval
Build a Large Language Model AI Chatbot using Retrieval Augmented Generation
'I want Llama3 to perform 10x with my private knowledge' - Local Agentic RAG w/ llama3
How Large Language Models Work
Tutorial 2- Fine Tuning Pretrained Model On Custom Dataset Using 🤗 Transformer
LlamaIndex Webinar: Finetuning + RAG
How do domain-specific chatbots work? An Overview of Retrieval Augmented Generation (RAG)
Should I Use RAG or Fine-Tuning?
Generative AI Fine Tuning LLM Models Crash Course
Building with Instruction-Tuned LLMs: A Step-by-Step Guide
Vector databases are so hot right now. WTF are they?
Introduction to large language models
Train a Small Language Model for Disease Symptoms | Step-by-Step Tutorial
How to Build an AI Document Chatbot in 10 Minutes
LlamaIndex Webinar: Retrieval-Augmented Fine-Tuning (RAFT)
Improving the Sample Efficiency of Prompt Tuning with Domain Adaptation
Using Retrieval-Augmented Generation to make domain-specific agents
High-performance RAG with LlamaIndex
LLM Explained | What is LLM
Custom Training Question Answer Model Using Transformer BERT
Embeddings vs Fine Tuning - Part 1, Embeddings
Knowledge Graph Construction Demo from raw text using an LLM
Build Anything with Llama 3 Agents, Here’s How
LlamaIndex Webinar: Learn about Fine-tuning + RAG (w/ Victoria Lin, author of RA-DIT)
Комментарии