filmov
tv
LLM Understanding: 22. Danilo BZDOK + Jack STANLEY
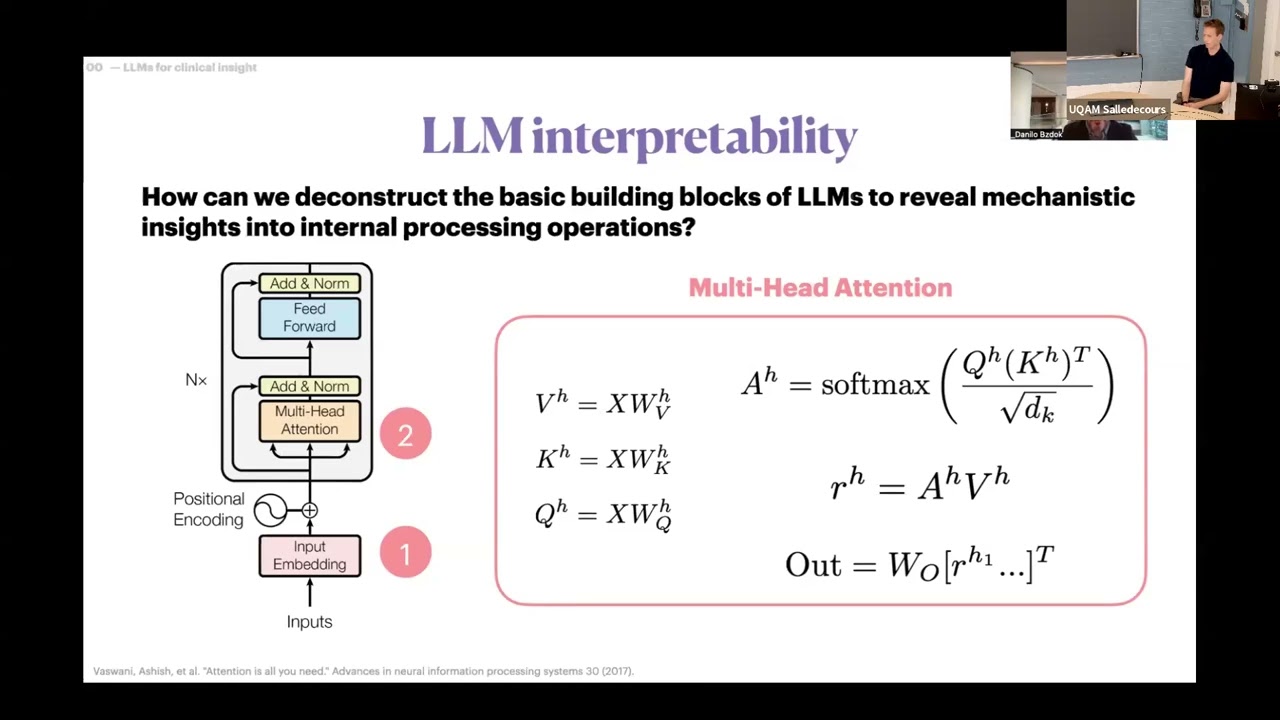
Показать описание
LLMs as Aid in Medical Diagnosis
Danilo Bzdok
Biomedical Engineering, McGill & MILA
ISC Summer School on Large Language Models: Science and Stakes, June 3-14, 2024
Mon, June 10, 11am-12:30pm EDT
Abstract: Despite considerable effort, we see diminishing returns in detecting people with autism using genome-wide assays or brain scans. In contrast, the clinical intuition of healthcare professionals, from longstanding first-hand experience, remains the best way to diagnose autism. In an alternative approach, we used deep learning to dissect and interpret the mind of the clinician. After pre-training on hundreds of millions of general sentences, we applied large language models (LLMs) to 4000 free-form health records from medical professionals to distinguish confirmed from suspected cases autism. With a mechanistic explanatory strategy, our extended LLM architecture could pin down the most salient single sentences in what drives clinical thinking towards correct diagnoses. It identified stereotyped repetitive behaviors, special interests, and perception-based behavior as the most autism-critical DSM-5 criteria. This challenges today’s focus on deficits in social interplay and suggests that long-trusted diagnostic criteria in gold standard instruments need to be revised.
Danilo Bzdok is an MD and computer scientist with a dual background in systems neuroscience and machine learning algorithms. His interdisciplinary research centers on the brain basis of human-defining types of thinking, with a special focus on the higher association cortex in health and disease. He uses population datasets (such as UK Biobank, HCP, CamCAN, ABCD) across levels of observation (brain structure and function, consequences from brain lesion, or common-variant genetics) with a broad toolkit of bioinformatic methods (machine-learning, high-dimensional statistics, and probabilistic Bayesian hierarchical modeling).
Bzdok, Danilo, et al. Data science opportunities of large language models for neuroscience and biomedicine. Neuron (2024).
Smallwood, J., Bernhardt, B. C., Leech, R., Bzdok, D., Jefferies, E., & Margulies, D. S. (2021). The default mode network in cognition: a topographical perspective. Nature Reviews Neuroscience, 22(8), 503-513.
Stanley, J., Rabot, E., Reddy, S., Belilovsky, E., Mottron, L., Bzdok, D (2024). Large language models deconstruct the clinical intuition behind diagnosing autism. Manuscript under review
Danilo Bzdok
Biomedical Engineering, McGill & MILA
ISC Summer School on Large Language Models: Science and Stakes, June 3-14, 2024
Mon, June 10, 11am-12:30pm EDT
Abstract: Despite considerable effort, we see diminishing returns in detecting people with autism using genome-wide assays or brain scans. In contrast, the clinical intuition of healthcare professionals, from longstanding first-hand experience, remains the best way to diagnose autism. In an alternative approach, we used deep learning to dissect and interpret the mind of the clinician. After pre-training on hundreds of millions of general sentences, we applied large language models (LLMs) to 4000 free-form health records from medical professionals to distinguish confirmed from suspected cases autism. With a mechanistic explanatory strategy, our extended LLM architecture could pin down the most salient single sentences in what drives clinical thinking towards correct diagnoses. It identified stereotyped repetitive behaviors, special interests, and perception-based behavior as the most autism-critical DSM-5 criteria. This challenges today’s focus on deficits in social interplay and suggests that long-trusted diagnostic criteria in gold standard instruments need to be revised.
Danilo Bzdok is an MD and computer scientist with a dual background in systems neuroscience and machine learning algorithms. His interdisciplinary research centers on the brain basis of human-defining types of thinking, with a special focus on the higher association cortex in health and disease. He uses population datasets (such as UK Biobank, HCP, CamCAN, ABCD) across levels of observation (brain structure and function, consequences from brain lesion, or common-variant genetics) with a broad toolkit of bioinformatic methods (machine-learning, high-dimensional statistics, and probabilistic Bayesian hierarchical modeling).
Bzdok, Danilo, et al. Data science opportunities of large language models for neuroscience and biomedicine. Neuron (2024).
Smallwood, J., Bernhardt, B. C., Leech, R., Bzdok, D., Jefferies, E., & Margulies, D. S. (2021). The default mode network in cognition: a topographical perspective. Nature Reviews Neuroscience, 22(8), 503-513.
Stanley, J., Rabot, E., Reddy, S., Belilovsky, E., Mottron, L., Bzdok, D (2024). Large language models deconstruct the clinical intuition behind diagnosing autism. Manuscript under review